Prediction of the Tribological Properties of Polytetrafluoroethylene Composites Based on Experiments and Machine Learning.
Polymers(2024)
摘要
Because of the complex nonlinear relationship between working conditions, the prediction of tribological properties has become a difficult problem in the field of tribology. In this study, we employed three distinct machine learning (ML) models, namely random forest regression (RFR), gradient boosting regression (GBR), and extreme gradient boosting (XGBoost), to predict the tribological properties of polytetrafluoroethylene (PTFE) composites under high-speed and high-temperature conditions. Firstly, PTFE composites were successfully prepared, and tribological properties under different temperature, speed, and load conditions were studied in order to explore wear mechanisms. Then, the investigation focused on establishing correlations between the friction and wear of PTFE composites by testing these parameters through the prediction of the friction coefficient and wear rate. Importantly, the correlation results illustrated that the friction coefficient and wear rate gradually decreased with the increase in speed, which was also proven by the correlation coefficient. In addition, the GBR model could effectively predict the tribological properties of the PTFE composites. Furthermore, an analysis of relative importance revealed that both load and speed exerted a greater influence on the prediction of the friction coefficient and wear rate.
更多查看译文
关键词
prediction,polytetrafluoroethylene composites,tribology,machine learning,Pearson correlation coefficient
AI 理解论文
溯源树
样例
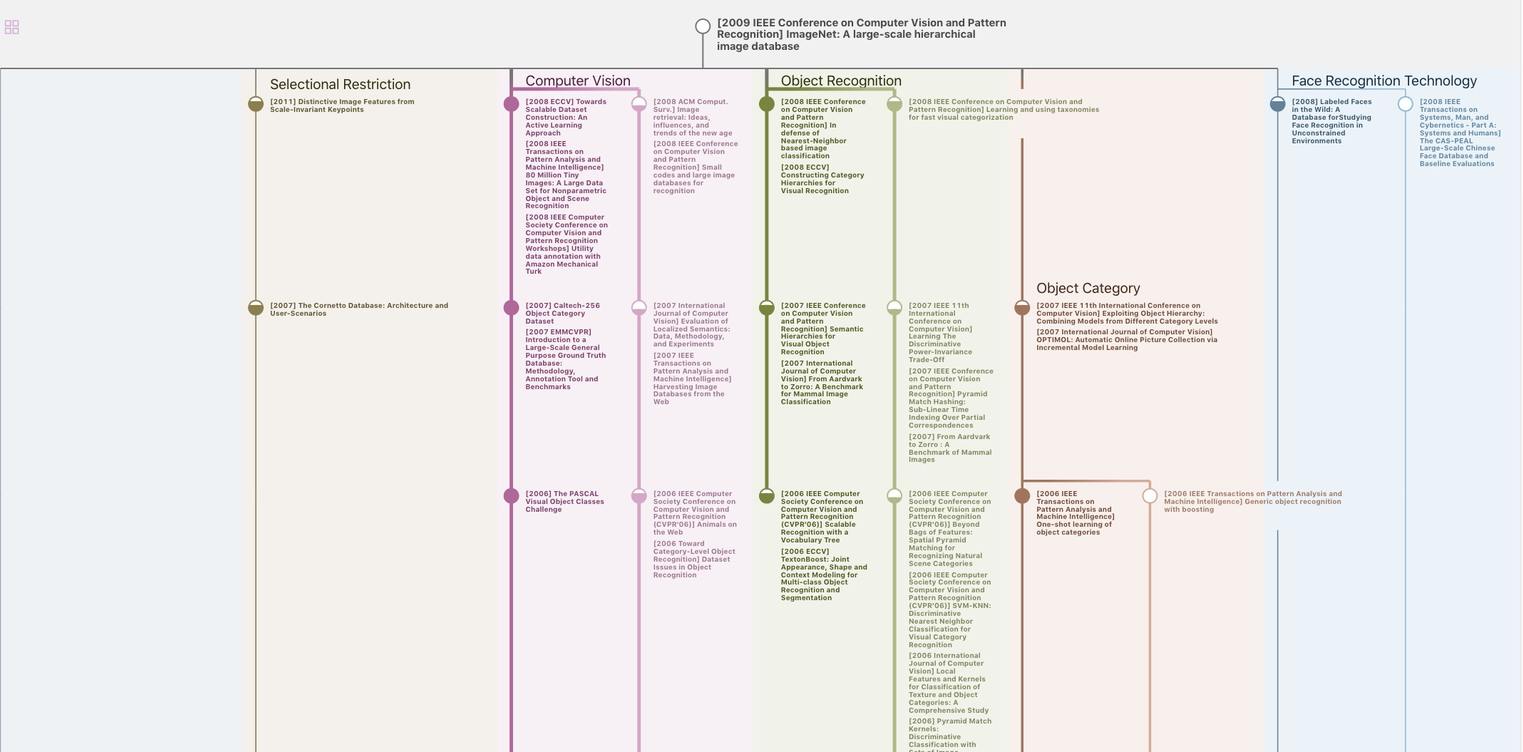
生成溯源树,研究论文发展脉络
Chat Paper
正在生成论文摘要