Development and application of a multi-task oriented deep learning model for quantifying drivers of air pollutant variations: A case study in Taiyuan, China
SCIENCE OF THE TOTAL ENVIRONMENT(2024)
摘要
Quantitative assessment of the drivers behind the variation of six criteria pollutants, namely fine particulate matter (PM2.5), ozone (O3), nitrogen dioxide (NO2), sulfur dioxide (SO2), particulate matter (PM10), and carbon monoxide (CO), in the warming climate will be critical for subsequent decision -making. Here, a novel hybrid model of multi -task oriented CNN-BiLSTM-Attention was proposed and performed in Taiyuan during 2015-2020 to synchronously and quickly quantify the impact of anthropogenic and meteorological factors on the six criteria pollutants variations. Empirical results revealed the residential and transportation sectors distinctly decreased SO2 by 25 % and 22 % and CO by 12 % and 10 %. Gradual downward trends for PM2.5, PM10, and NO2 were mainly ascribed to the stringent measures implemented in transportation and power sectors as part of the Blue Sky Defense War, which were further reinforced by the COVID-19 pandemic. Nevertheless, temperaturedependent adverse meteorological effects (27 %) and anthropogenic intervention (12 %) jointly increased O3 by 39 %. The O3 -driven pollution events may be inevitable or even become more prominent under climate warming. The industrial (5 %) and transportation sectors (6 %) were mainly responsible for the anthropogenicdriven increase of O3 and precursor NO2, respectively. Synergistic reduction of precursors (VOCs and NOx) from industrial and transportation sectors requires coordination with climate actions to mitigate the temperaturedependent O3 -driven pollution, thereby improving regional air quality. Meanwhile, the proposed model is expected to be applied flexibly in various regions to quantify the drivers of the pollutant variations in a warming climate, with the potential to offer valuable insights for improving regional air quality in near future.
更多查看译文
关键词
Air pollutants,Deep learning,Anthropogenic intervention,Meteorology,Meteorological normalization
AI 理解论文
溯源树
样例
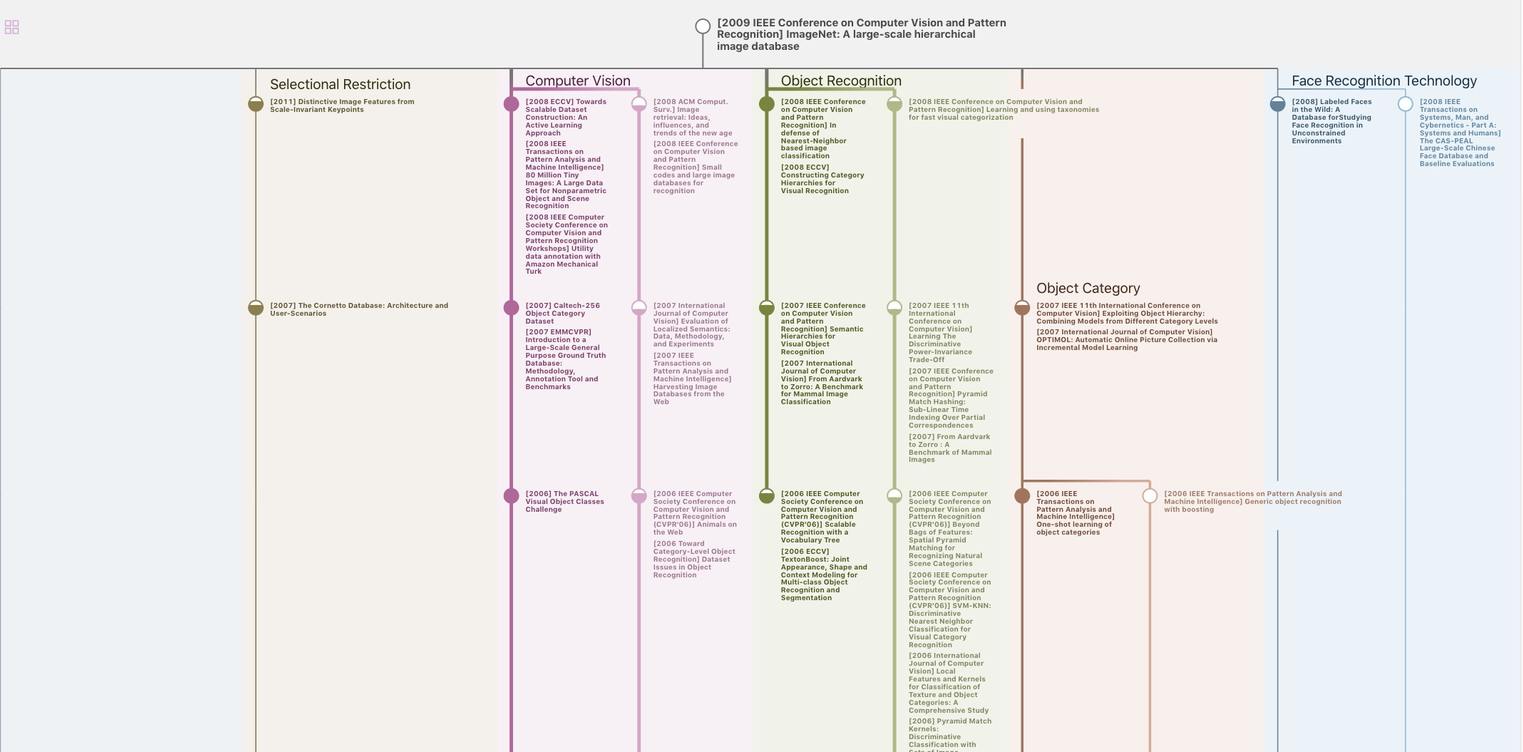
生成溯源树,研究论文发展脉络
Chat Paper
正在生成论文摘要