Detective-Dee: a Non-intrusive In Situ Anomaly Detection and Fault Localization Framework
2023 42ND INTERNATIONAL SYMPOSIUM ON RELIABLE DISTRIBUTED SYSTEMS, SRDS 2023(2023)
摘要
Maintaining the high availability of online systems requires reliable and fast online anomaly detection and fault localization. However, existing anomaly detection methods either suffer high training costs and low generalization capabilities or are designed and evaluated using offline data with limited efficacy in online usage. Furthermore, these methods ' fault localization capabilities are often inadequate due to external observability constraints. Therefore, designing a new approach to address these limitations effectively is essential. To address the aforementioned limitations, this paper proposes a novel non-intrusive in situ anomaly detection and fault localization framework, Detective-Dee. The proposed framework leverages a compressed sensing method for anomaly detection, which exhibits strong generalization capabilities and eliminates extensive training. Detective-Dee further improves its performance by incorporating three optimization techniques: concurrent substitution sampling, Look-Up-Table-based similarity calculation, and substitution window-based threshold selection to improve parallelism and reduce computational and comparison overheads. Additionally, the framework adopts an innovative non-intrusive fault localization strategy based on anomaly detection triggering. This approach utilizes the dynamic instrumentation capabilities of eBPF, combined with extracting vulnerable function and function call chains through source code analysis, to improve the online anomaly detection capability and achieve robust fault localization with low overhead. To validate the effectiveness of Detective-Dee, we developed a prototype system and conducted a comprehensive evaluation. The results demonstrate that, compared to the state-of-the-art anomaly detection method, Detective-Dee exhibits a 4x improvement in anomaly detection speed while maintaining higher online and comparable offline detection ability. Furthermore, under 33 real-world fault cases across eight popular distributed systems, Detective-Dee successfully detects 31 cases and accurately locates 26 cases with less than 1% overhead, outperforming the state-ofthe-art method.
更多查看译文
关键词
cloud-based online service systems,anomaly detection,fault localization,operating system,eBPF
AI 理解论文
溯源树
样例
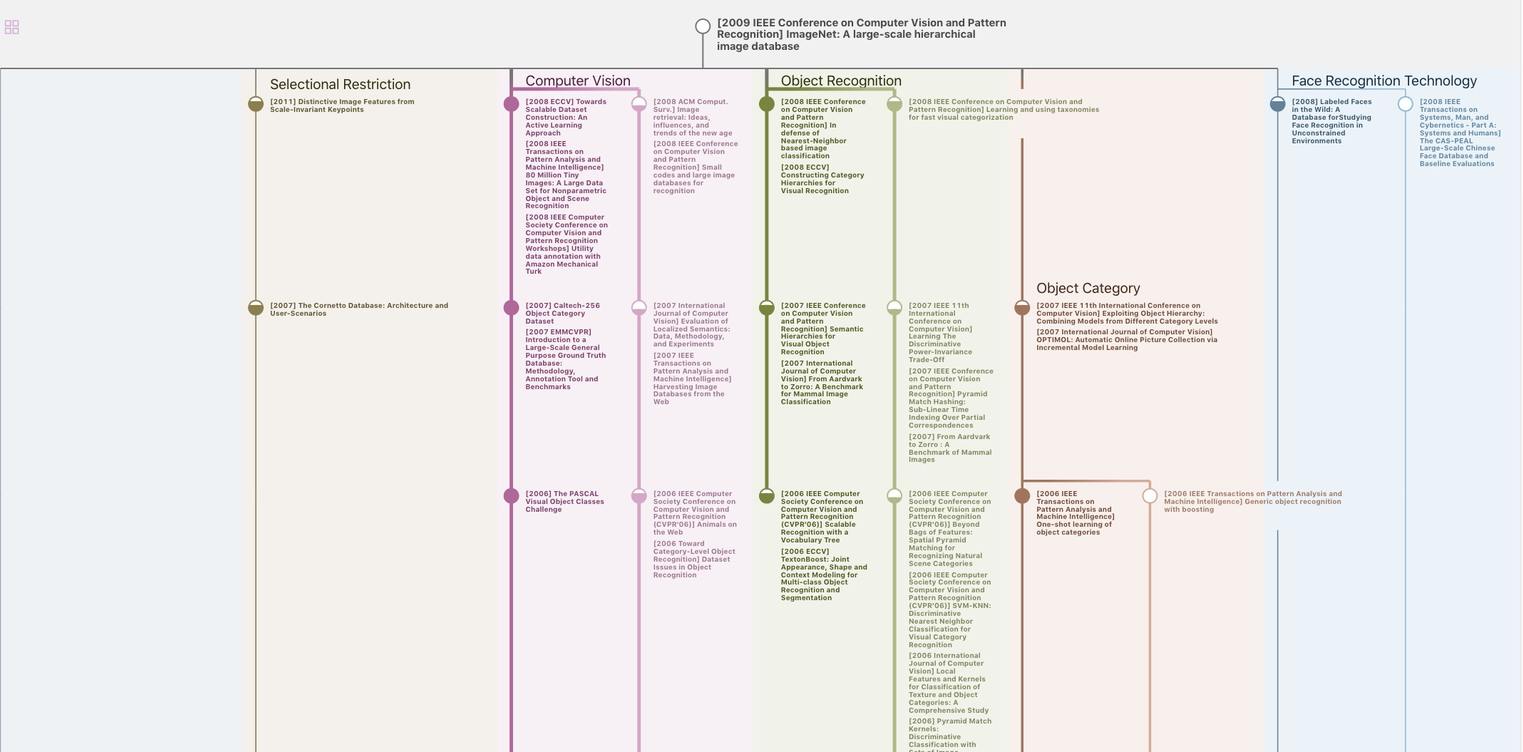
生成溯源树,研究论文发展脉络
Chat Paper
正在生成论文摘要