Characterizing advanced heart failure risk and hemodynamic phenotypes using interpretable machine learning
American Heart Journal(2024)
摘要
Background
Although previous risk models exist for advanced heart failure with reduced ejection fraction (HFrEF), few integrate invasive hemodynamics or support missing data. This study developed and validated a heart failure (HF) hemodynamic risk and phenotyping score for HFrEF, using Machine Learning (ML).
Methods
Prior to modeling, patients in training and validation HF cohorts were assigned to 1 of 5 risk categories based on the composite endpoint of death, left ventricular assist device (LVAD) implantation or transplantation (DeLvTx), and rehospitalization in 6 months of follow-up using unsupervised clustering. The goal of our novel interpretable ML modeling approach, which is robust to missing data, was to predict this risk category (1, 2, 3, 4, or 5) using either invasive hemodynamics alone or a rich and inclusive feature set that included noninvasive hemodynamics (all features). The models were trained using the ESCAPE trial and validated using 4 advanced HF patient cohorts collected from previous trials, then compared with traditional ML models. Prediction accuracy for each of these 5 categories was determined separately for each risk category to generate 5 areas under the curve (AUCs, or C-statistics) for belonging to risk category 1, 2, 3, 4, or 5, respectively.
Results
Across all outcomes, our models performed well for predicting the risk category for each patient. Accuracies of 5 separate models predicting a patient's risk category ranged from 0.896 +/- 0.074 to 0.969 +/- 0.081 for the invasive hemodynamics feature set and 0.858 +/- 0.067 to 0.997 +/- 0.070 for the all features feature set.
Conclusion
Novel interpretable ML models predicted risk categories with a high degree of accuracy. This approach offers a new paradigm for risk stratification that differs from prediction of a binary outcome. Prospective clinical evaluation of this approach is indicated to determine utility for selecting the best treatment approach for patients based on risk and prognosis.
更多查看译文
AI 理解论文
溯源树
样例
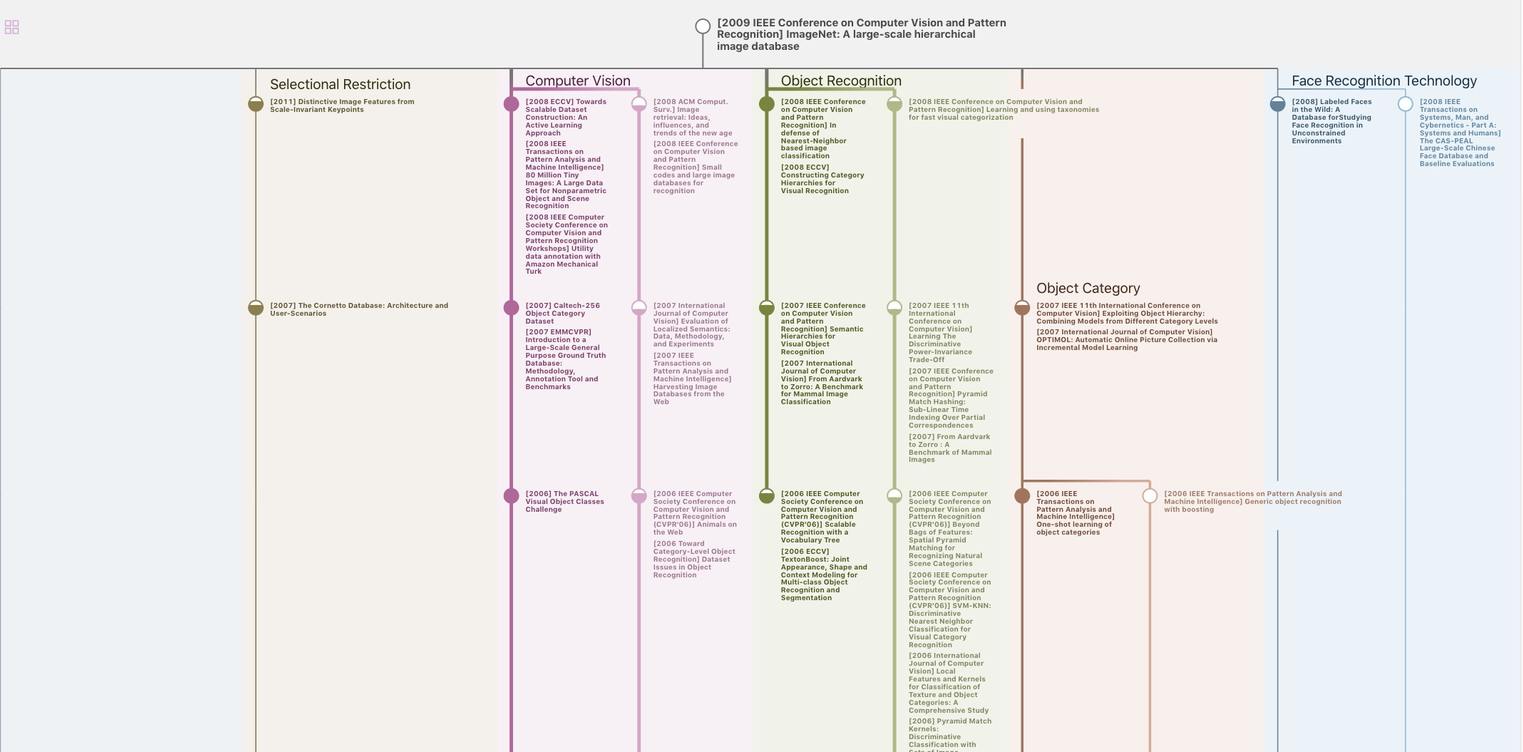
生成溯源树,研究论文发展脉络
Chat Paper
正在生成论文摘要