Generating Higher Order Modes from Binary Black Hole mergers with Machine Learning
Physical Review D(2024)
摘要
We introduce a machine learning model designed to rapidly and accurately
predict the time domain gravitational wave emission of non-precessing binary
black hole coalescences, incorporating the effects of higher order modes of the
multipole expansion of the waveform. Expanding on our prior work, we decompose
each mode by amplitude and phase and reduce dimensionality using principal
component analysis. An ensemble of artificial neural networks is trained to
learn the relationship between orbital parameters and the low-dimensional
representation of each mode. We train our model on ∼ 10^5 signals with
mass ratio q ∈ [1,10] and dimensionless spins χ_i ∈ [-0.9, 0.9],
generated with the state-of-the-art approximant SEOBNRv4HM. We find that it
achieves a median faithfulness of 10^-4 averaged across the parameter
space. We show that our model generates a single waveform two orders of
magnitude faster than the training model, with the speed up increasing when
waveforms are generated in batches. This framework is entirely general and can
be applied to any other time domain approximant capable of generating waveforms
from aligned spin circular binaries, possibly incorporating higher order modes.
更多查看译文
AI 理解论文
溯源树
样例
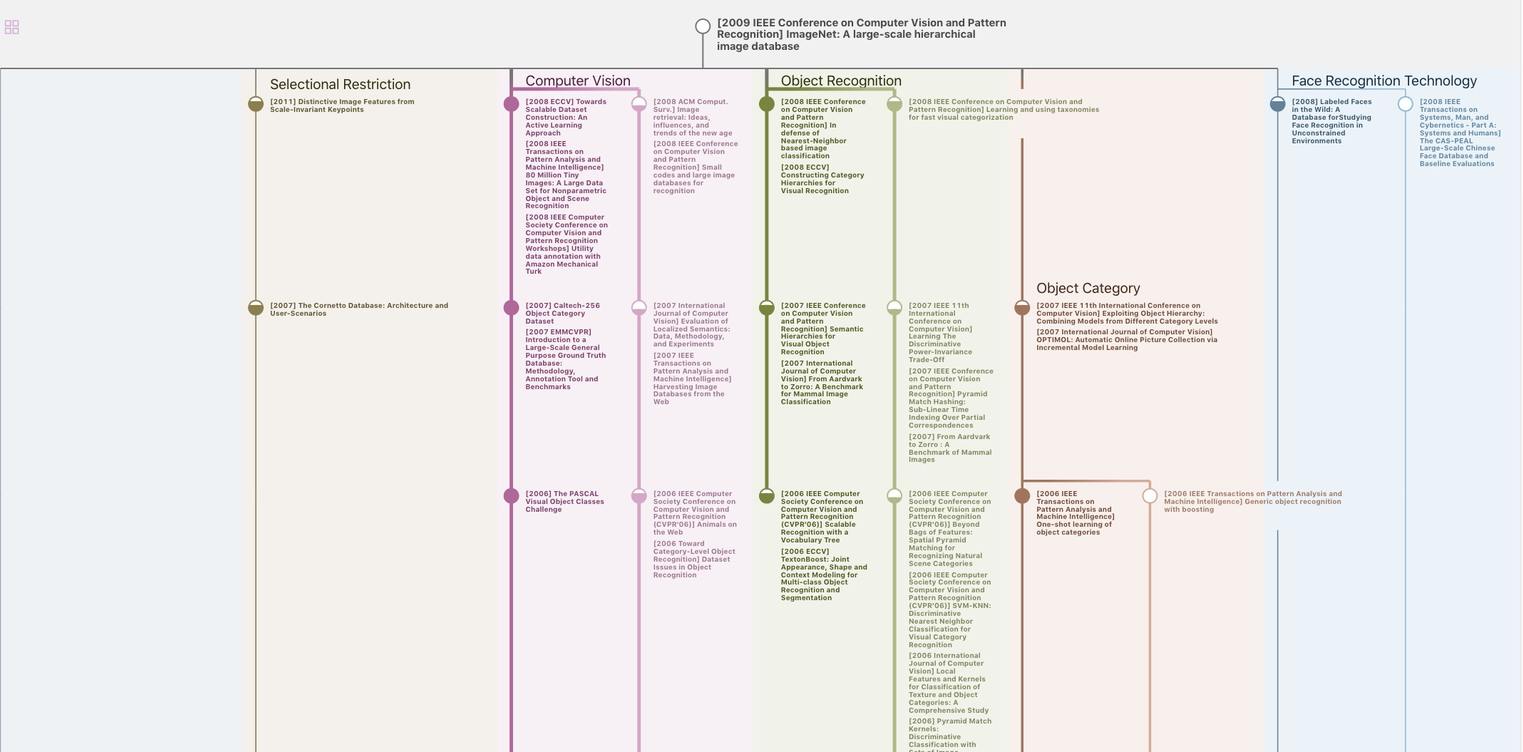
生成溯源树,研究论文发展脉络
Chat Paper
正在生成论文摘要