Thermal Transport of Glasses Via Machine Learning Driven Simulations
Frontiers in materials(2024)
摘要
Accessing the thermal transport properties of glasses is a major issue forthe design of production strategies of glass industry, as well as for theplethora of applications and devices where glasses are employed. From thecomputational standpoint, the chemical and morphological complexity of glassescalls for atomistic simulations where the interatomic potentials are able tocapture the variety of local environments, composition, and (dis)order thattypically characterize glassy phases. Machine-learning potentials (MLPs) areemerging as a valid alternative to computationally expensive ab initiosimulations, inevitably run on very small samples which cannot account fordisorder at different scales, as well as to empirical force fields, fast butoften reliable only in a narrow portion of the thermodynamic and compositionphase diagrams. In this article, we make the point on the use of MLPs tocompute the thermal conductivity of glasses, through a review of recenttheoretical and computational tools and a series of numerical applications onvitreous silica and vitreous silicon, both pure and intercalated with lithium.
更多查看译文
关键词
thermal transport,machine learning,glasses,thermal properties,Green Kubo method,molecular dynamics,cepstral analisys
AI 理解论文
溯源树
样例
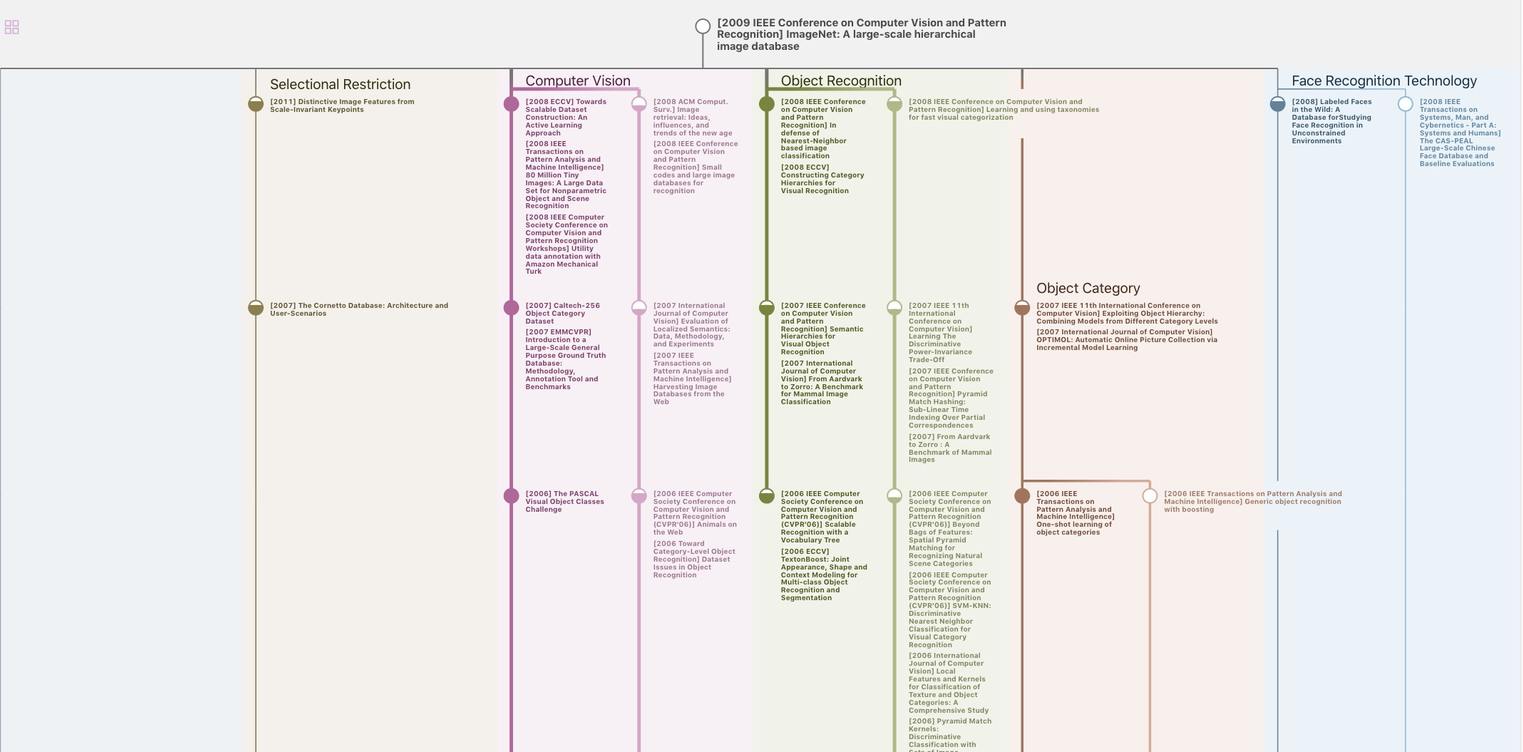
生成溯源树,研究论文发展脉络
Chat Paper
正在生成论文摘要