Animated Stickers: Bringing Stickers to Life with Video Diffusion
CoRR(2024)
摘要
We introduce animated stickers, a video diffusion model which generates an
animation conditioned on a text prompt and static sticker image. Our model is
built on top of the state-of-the-art Emu text-to-image model, with the addition
of temporal layers to model motion. Due to the domain gap, i.e. differences in
visual and motion style, a model which performed well on generating natural
videos can no longer generate vivid videos when applied to stickers. To bridge
this gap, we employ a two-stage finetuning pipeline: first with weakly
in-domain data, followed by human-in-the-loop (HITL) strategy which we term
ensemble-of-teachers. It distills the best qualities of multiple teachers into
a smaller student model. We show that this strategy allows us to specifically
target improvements to motion quality while maintaining the style from the
static image. With inference optimizations, our model is able to generate an
eight-frame video with high-quality, interesting, and relevant motion in under
one second.
更多查看译文
AI 理解论文
溯源树
样例
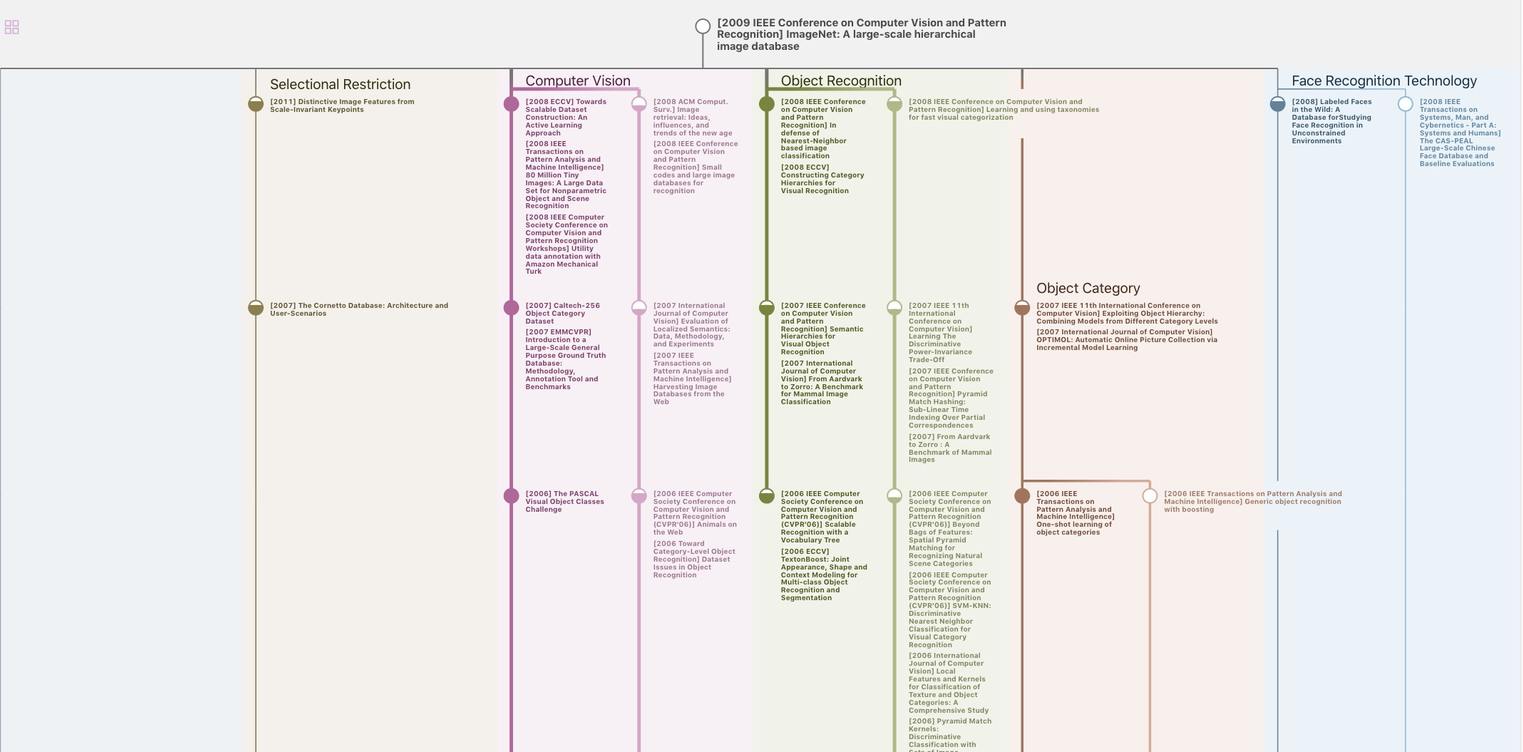
生成溯源树,研究论文发展脉络
Chat Paper
正在生成论文摘要