Lessons learned from covid-19 modelling efforts for policy decision-making in lower- and middle-income countries
medrxiv(2024)
摘要
Introduction The COVID-19 pandemic had devastating health and socio-economic effects, partly due to mitigating policy choices. There is little evidence of approaches that guided policy decisions in settings that had limited modelling capacity pre-pandemic. We sought to identify knowledge translation mechanisms, enabling factors, and structures needed to translate modelled evidence to policy decisions effectively.
Methods We utilised convergent mixed methods in a participatory action approach, with quantitative data from a survey and qualitative data from a scoping review, in-depth interviews, and workshop notes. Participants included researchers and policy actors involved in COVID-19 evidence generation and decision-making. They were mostly from lower-and middle-income countries (LMICs) in Africa, Southeast Asia, and Latin America. Quantitative and qualitative data integration occurred during data analysis through triangulation and during reporting in a narrative synthesis.
Results We engaged 147 researchers and 57 policy actors from 28 countries. We found that the strategies required to use modelling evidence effectively include capacity building of modelling expertise and communication, improved data infrastructure, sustained funding, and dedicated knowledge translation platforms. The common knowledge translation mechanisms used during the pandemic included policy briefs, face-to-face debriefings, and dashboards. Some enabling factors for knowledge translation comprised solid relationships and open communication between researchers and policymakers, credibility of researchers, co-production of policy questions, and embedding researchers in policymaking spaces. Barriers included competition among modellers, negative attitude of policymakers towards research, political influences and demand for quick outputs.
Conclusion Our findings led to the co-development of a knowledge translation framework useful in various settings to guide decision-making, especially for public health emergencies. Furthermore, we provide a contextualised understanding of knowledge translation for LMICs during the COVID-19 pandemic. Finally, we share key lessons on how knowledge translation from mathematical modelling complements the broader learning agenda related to pandemic preparedness and long-term investments in evidence-to-policy translation.
What is already known on this topic
What this study adds
How this study might affect research, practice, or policy
### Competing Interest Statement
The authors have declared no competing interest.
### Funding Statement
Funds from the Bill and Melinda Gates Foundation grant (INV-034291) awarded to Dr Jacquie N Oliwa supported this work. The views in this article are those of the authors and do not represent the official views of the Gates Foundation or WHO. The funders had no direct role in study design, data collection and analysis, the decision to publish or the preparation of the manuscript.
### Author Declarations
I confirm all relevant ethical guidelines have been followed, and any necessary IRB and/or ethics committee approvals have been obtained.
Yes
The details of the IRB/oversight body that provided approval or exemption for the research described are given below:
This study was approved by The KEMRI (Kenya Medical Research Institute) Scientific and Ethics Review Unit (SERU): Protocol number - KEMRI/SERU/CGMR-C/4425.
I confirm that all necessary patient/participant consent has been obtained and the appropriate institutional forms have been archived, and that any patient/participant/sample identifiers included were not known to anyone (e.g., hospital staff, patients or participants themselves) outside the research group so cannot be used to identify individuals.
Yes
I understand that all clinical trials and any other prospective interventional studies must be registered with an ICMJE-approved registry, such as ClinicalTrials.gov. I confirm that any such study reported in the manuscript has been registered and the trial registration ID is provided (note: if posting a prospective study registered retrospectively, please provide a statement in the trial ID field explaining why the study was not registered in advance).
Yes
I have followed all appropriate research reporting guidelines, such as any relevant EQUATOR Network research reporting checklist(s) and other pertinent material, if applicable.
Yes
The survey responses and qualitative interview transcripts are housed on secure servers at the KEMRI-Wellcome Trust Research Programme. Access applications can be made through the Data Governance Committee, with details available at [www.kemri-wellcome.org][1] or email to dgc{at}kemri-wellcome.org.
[1]: http://www.kemri-wellcome.org
更多查看译文
AI 理解论文
溯源树
样例
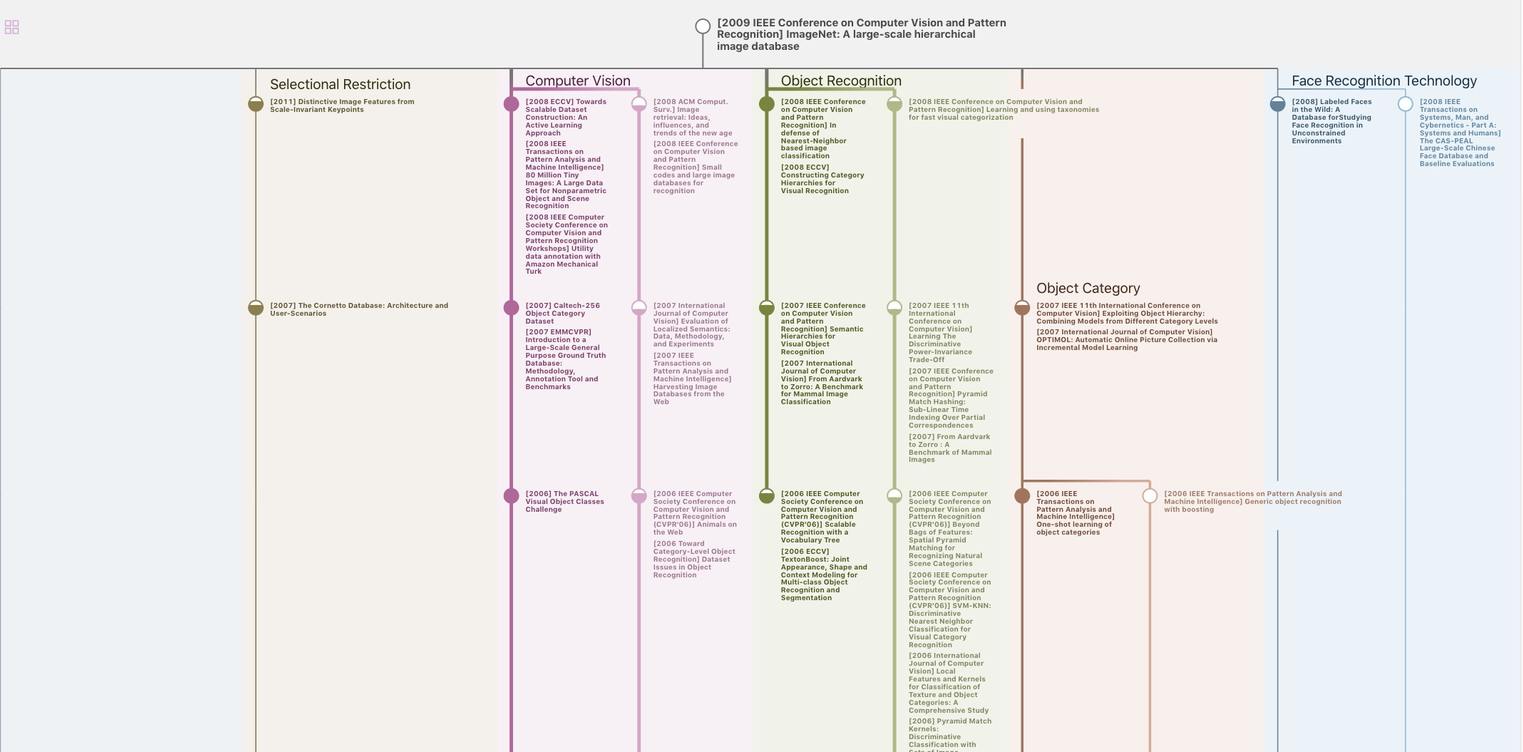
生成溯源树,研究论文发展脉络
Chat Paper
正在生成论文摘要