Using machine learning to integrate genetic and environmental data to model genotype-by-environment interactions
biorxiv(2024)
摘要
Complementing phenotypic traits and molecular markers with high-dimensional data such as climate and soil information is becoming a common practice in breeding programs. This study explored new ways to integrate non-genetic information in genomic prediction models using machine learning (ML). Using the multi-environment trial data from the Genomes To Fields initiative, different models to predict maize grain yield were adjusted using various inputs: genetic, environmental, or a combination of both, either in an additive (genetic-and-environmental; G+E) or a multiplicative (genotype-by-environment interaction; GEI) manner. When including environmental data, the mean predictive ability of machine learning genomic prediction models increased from 7-9% over the well-established Factor Analytic Multiplicative Mixed Model (FA) among the three cross-validation scenarios evaluated. Moreover, using the G+E model was more advantageous than the GEI model given the superior, or at least comparable, predictive ability, the lower usage of computational memory and time, and the flexibility of accounting for interactions by construction. Our results illustrate the flexibility provided by the ML framework, particularly with feature engineering. We show that the featured engineering stage offers a viable option for envirotyping and generates valuable information for machine learning-based genomic prediction models. Furthermore, we verified that the genotype-by-environment interactions may be considered using tree-based approaches without explicitly including interactions in the model. These findings support the growing interest in merging high-dimensional genotypic and environmental data into predictive modeling.
### Competing Interest Statement
The authors have declared no competing interest.
更多查看译文
AI 理解论文
溯源树
样例
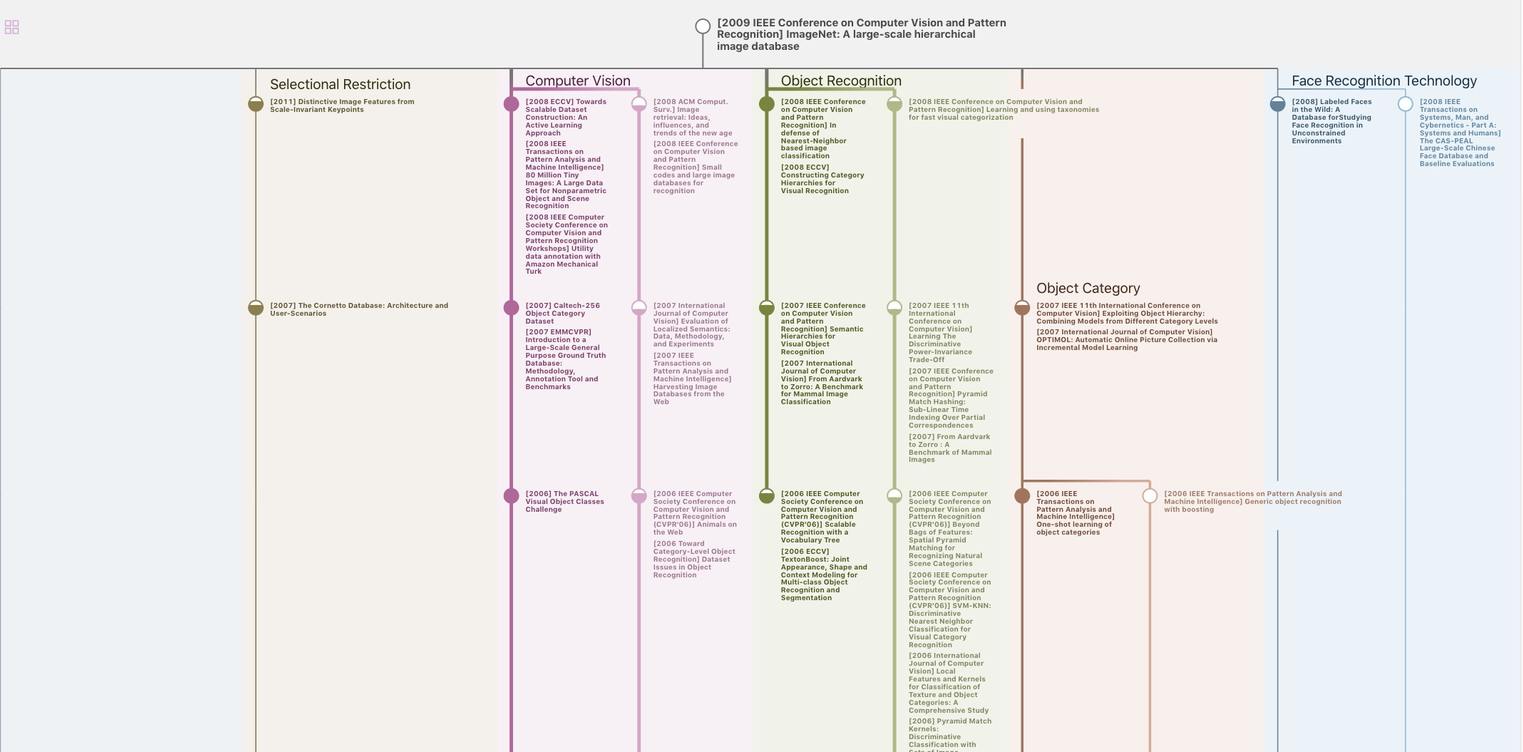
生成溯源树,研究论文发展脉络
Chat Paper
正在生成论文摘要