Correspondence-based Generative Bayesian Deep Learning for semi-supervised volumetric medical image segmentation
COMPUTERIZED MEDICAL IMAGING AND GRAPHICS(2024)
摘要
Automated medical image segmentation plays a crucial role in diverse clinical applications. The high annotation costs of fully -supervised medical segmentation methods have spurred a growing interest in semisupervised methods. Existing semi -supervised medical segmentation methods train the teacher segmentation network using labeled data to establish pseudo labels for unlabeled data. The quality of these pseudo labels is constrained as these methods fail to effectively address the significant bias in the data distribution learned from the limited labeled data. To address these challenges, this paper introduces an innovative Correspondencebased Generative Bayesian Deep Learning (C-GBDL) model. Built upon the teacher-student architecture, we design a multi -scale semantic correspondence method to aid the teacher model in generating high -quality pseudo labels. Specifically, our teacher model, embedded with the multi -scale semantic correspondence, learns a better -generalized data distribution from input volumes by feature matching with the reference volumes. Additionally, a double uncertainty estimation schema is proposed to further rectify the noisy pseudo labels. The double uncertainty estimation takes the predictive entropy as the first uncertainty estimation and takes the structural similarity between the input volume and its corresponding reference volumes as the second uncertainty estimation. Four groups of comparative experiments conducted on two public medical datasets demonstrate the effectiveness and the superior performance of our proposed model. Our code is available on https://github.com/yumjoo/C-GBDL.
更多查看译文
关键词
Medical image segmentation,Semi-supervision,Semantic correspondence,Bayesian Deep Learning,Double uncertainty estimation
AI 理解论文
溯源树
样例
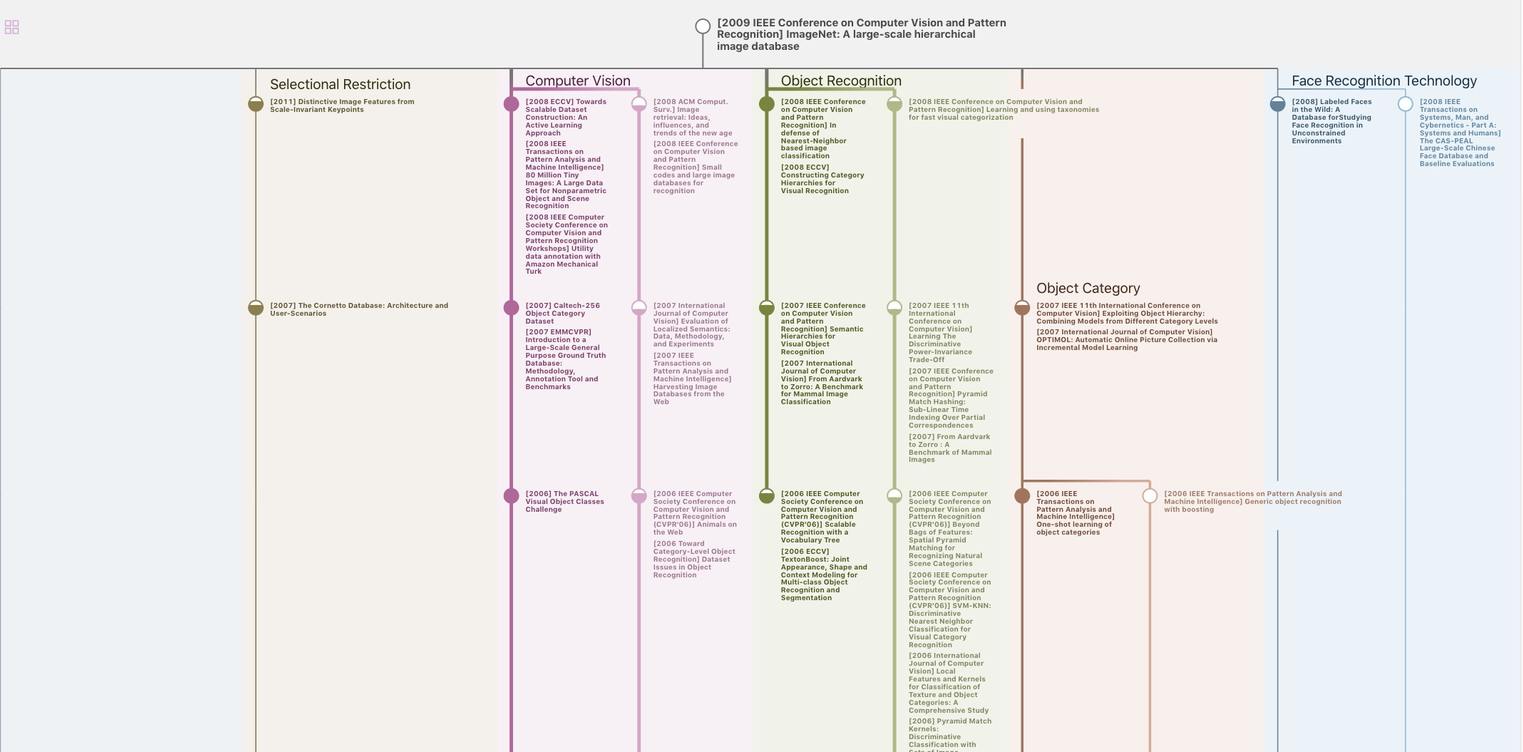
生成溯源树,研究论文发展脉络
Chat Paper
正在生成论文摘要