AI-based Landing Zone Detection for Vertical Takeoff and Land LiDAR Localization and Mapping Pipelines
Drone Systems and Applications(2024)
摘要
This paper proposed a novel point-based neural network landing zone detection architecture that can operate with a VLOAM navigation pipeline and investigates the accuracy-runtime trade-offs of the method for real-time applications. Based on the Semantic3D benchmark leaderboard, ConvPoint architecture was selected as the target model for the task. The work investigated different combinations of hyperparameters, i.e., batch size and sampling size, in terms of the performance metrics, i.e., inference time, throughput, and accuracy. Validation of the method was performed using custom datasets captured on a DJI M600 drone and a Bell 412 aircraft to generate the LZ module's maps at a target update rate (~ 1 Hz) while operating within a VLOAM navigation pipeline. Accurate detection of water bodies, marshlands, and low vegetation as non-landable is crucial for VTOL operations. From the results described in this paper, it is evident that to get a comparatively accurate detection of water areas in the given dataset, a larger sampling size should be set, which also can lead to lower throughput (higher inference time). This bottleneck can be resolved by fusing the semantic labels generated by the point cloud segmentation with the pixel labels generated by the color image semantic segmentation of the same region and by using a broader range of datasets to train the neural network model.
更多查看译文
AI 理解论文
溯源树
样例
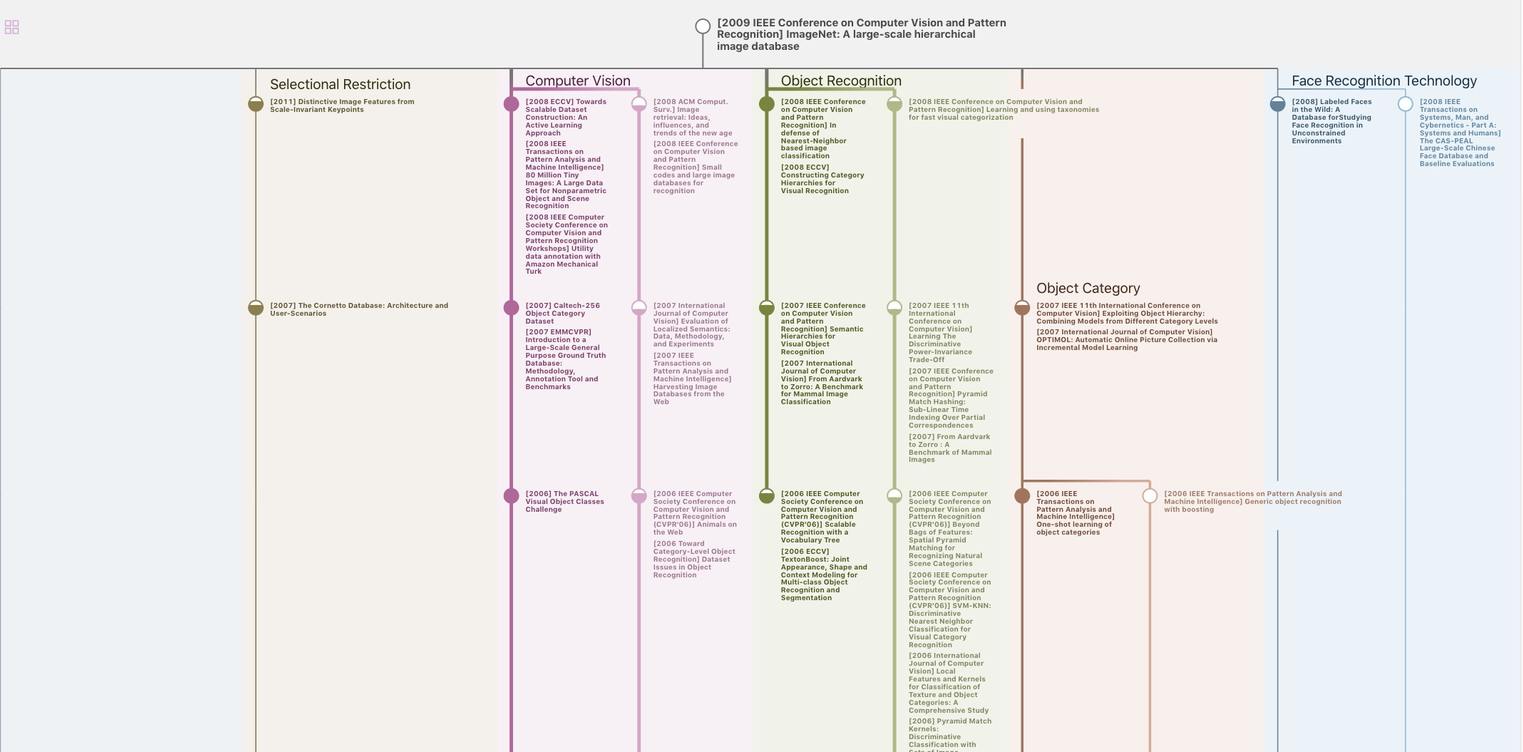
生成溯源树,研究论文发展脉络
Chat Paper
正在生成论文摘要