Joint estimation of the predictive ability of experts using a multi-output Gaussian process
arxiv(2024)
摘要
A multi-output Gaussian process (GP) is introduced as a model for the joint
posterior distribution of the local predictive ability of set of models and/or
experts, conditional on a vector of covariates, from historical predictions in
the form of log predictive scores. Following a power transformation of the log
scores, a GP with Gaussian noise can be used, which allows faster computation
by first using Hamiltonian Monte Carlo to sample the hyper-parameters of the GP
from a model where the latent GP surface has been marginalized out, and then
using these draws to generate draws of joint predictive ability conditional on
a new vector of covariates. Linear pools based on learned joint local
predictive ability are applied to predict daily bike usage in Washington DC.
更多查看译文
AI 理解论文
溯源树
样例
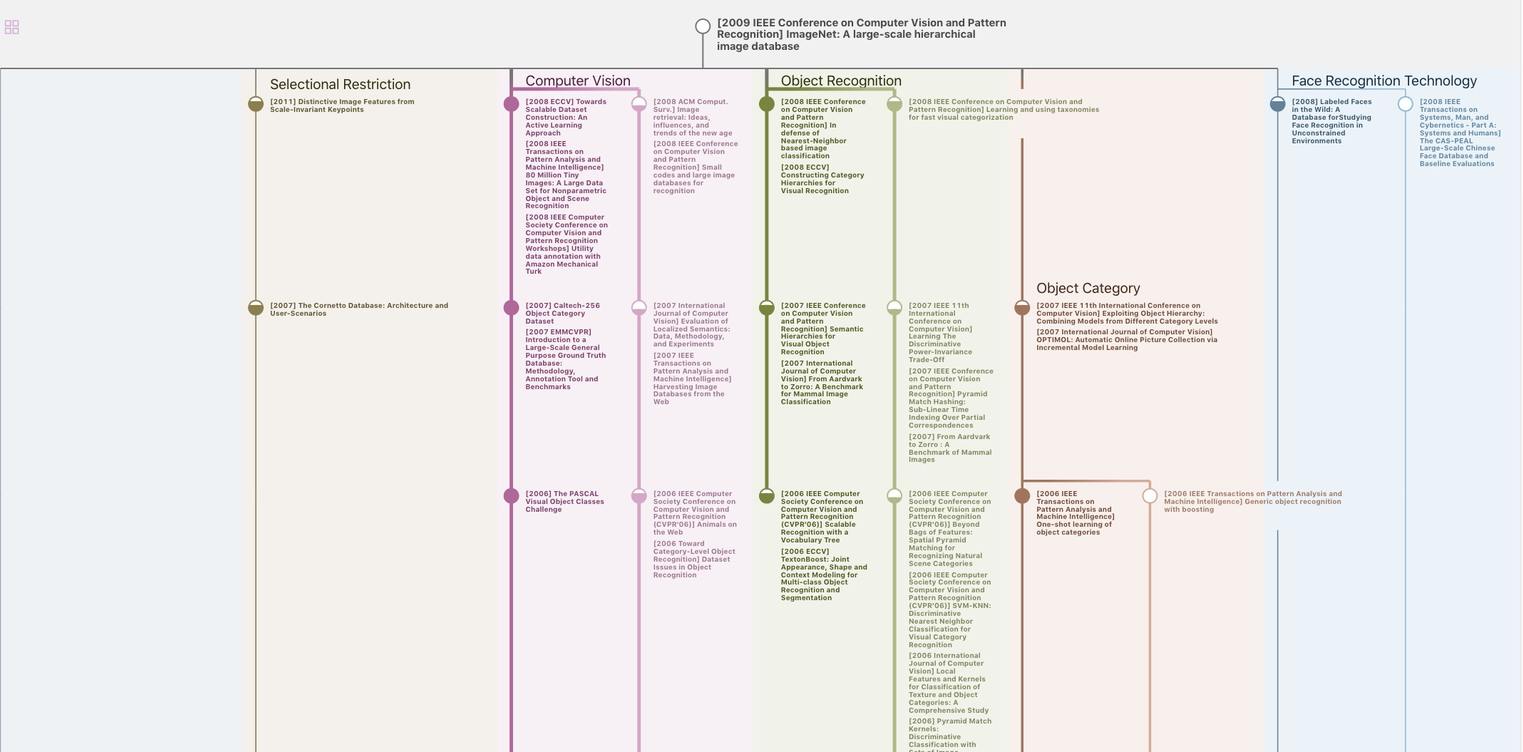
生成溯源树,研究论文发展脉络
Chat Paper
正在生成论文摘要