Towards Explainable, Safe Autonomous Driving with Language Embeddings for Novelty Identification and Active Learning: Framework and Experimental Analysis with Real-World Data Sets
CoRR(2024)
摘要
This research explores the integration of language embeddings for active
learning in autonomous driving datasets, with a focus on novelty detection.
Novelty arises from unexpected scenarios that autonomous vehicles struggle to
navigate, necessitating higher-level reasoning abilities. Our proposed method
employs language-based representations to identify novel scenes, emphasizing
the dual purpose of safety takeover responses and active learning. The research
presents a clustering experiment using Contrastive Language-Image Pretrained
(CLIP) embeddings to organize datasets and detect novelties. We find that the
proposed algorithm effectively isolates novel scenes from a collection of
subsets derived from two real-world driving datasets, one vehicle-mounted and
one infrastructure-mounted. From the generated clusters, we further present
methods for generating textual explanations of elements which differentiate
scenes classified as novel from other scenes in the data pool, presenting
qualitative examples from the clustered results. Our results demonstrate the
effectiveness of language-driven embeddings in identifying novel elements and
generating explanations of data, and we further discuss potential applications
in safe takeovers, data curation, and multi-task active learning.
更多查看译文
AI 理解论文
溯源树
样例
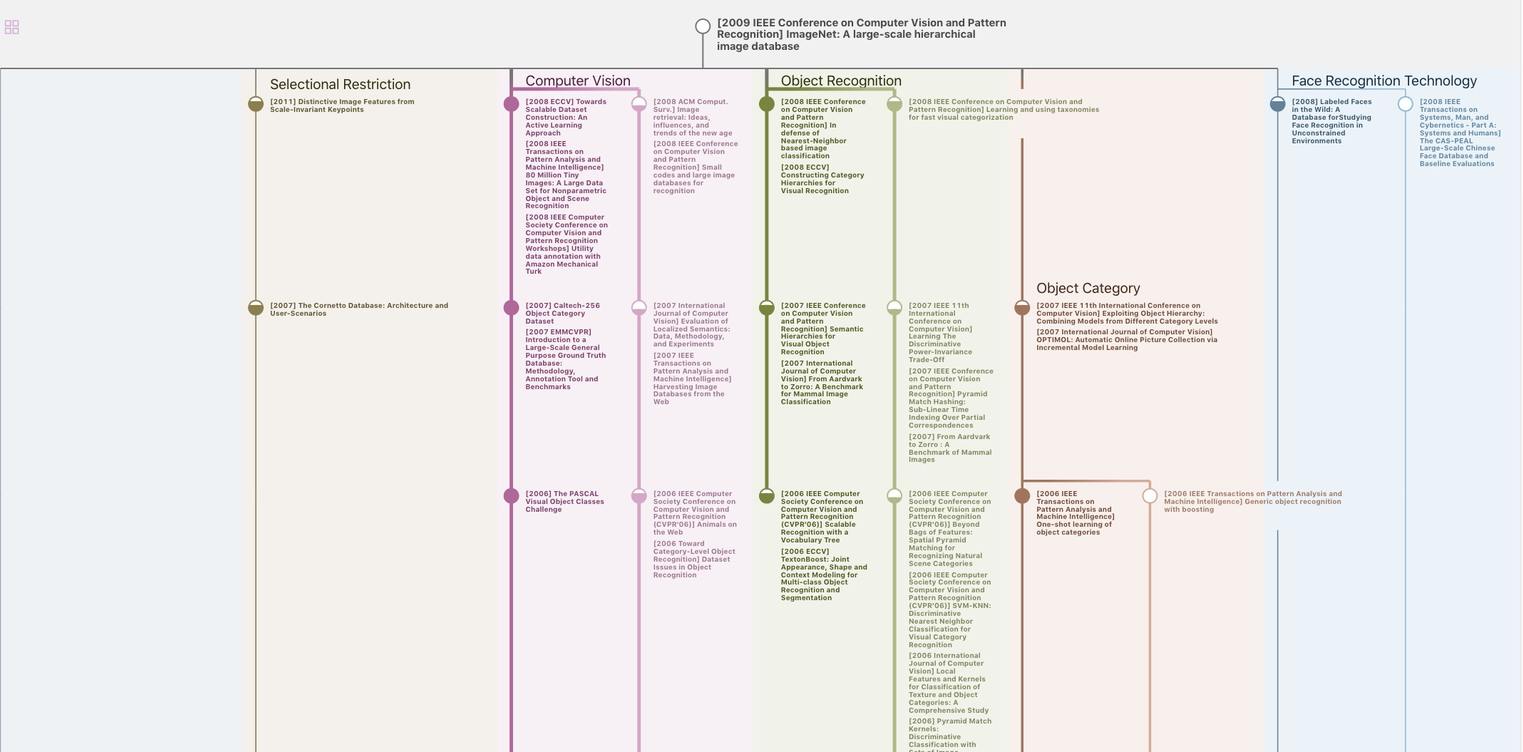
生成溯源树,研究论文发展脉络
Chat Paper
正在生成论文摘要