Auxiliary Reward Generation with Transition Distance Representation Learning
CoRR(2024)
摘要
Reinforcement learning (RL) has shown its strength in challenging sequential
decision-making problems. The reward function in RL is crucial to the learning
performance, as it serves as a measure of the task completion degree. In
real-world problems, the rewards are predominantly human-designed, which
requires laborious tuning, and is easily affected by human cognitive biases. To
achieve automatic auxiliary reward generation, we propose a novel
representation learning approach that can measure the “transition distance”
between states. Building upon these representations, we introduce an auxiliary
reward generation technique for both single-task and skill-chaining scenarios
without the need for human knowledge. The proposed approach is evaluated in a
wide range of manipulation tasks. The experiment results demonstrate the
effectiveness of measuring the transition distance between states and the
induced improvement by auxiliary rewards, which not only promotes better
learning efficiency but also increases convergent stability.
更多查看译文
AI 理解论文
溯源树
样例
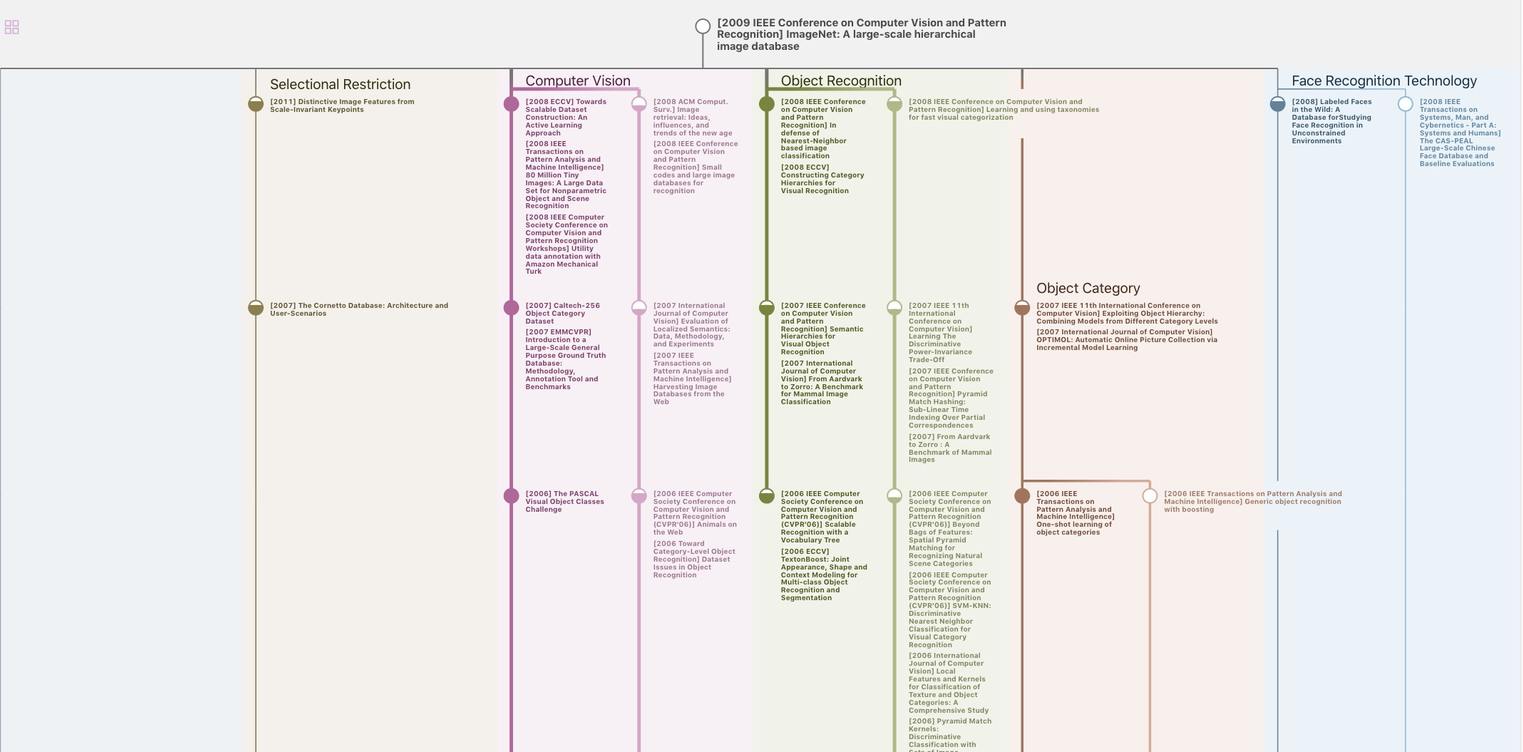
生成溯源树,研究论文发展脉络
Chat Paper
正在生成论文摘要