Intrinsic Task-based Evaluation for Referring Expression Generation
CoRR(2024)
摘要
Recently, a human evaluation study of Referring Expression Generation (REG)
models had an unexpected conclusion: on webnlg, Referring Expressions
(REs) generated by the state-of-the-art neural models were not only
indistinguishable from the REs in webnlg but also from the REs
generated by a simple rule-based system. Here, we argue that this limitation
could stem from the use of a purely ratings-based human evaluation (which is a
common practice in Natural Language Generation). To investigate these issues,
we propose an intrinsic task-based evaluation for REG models, in which, in
addition to rating the quality of REs, participants were asked to accomplish
two meta-level tasks. One of these tasks concerns the referential success of
each RE; the other task asks participants to suggest a better alternative for
each RE. The outcomes suggest that, in comparison to previous evaluations, the
new evaluation protocol assesses the performance of each REG model more
comprehensively and makes the participants' ratings more reliable and
discriminable.
更多查看译文
AI 理解论文
溯源树
样例
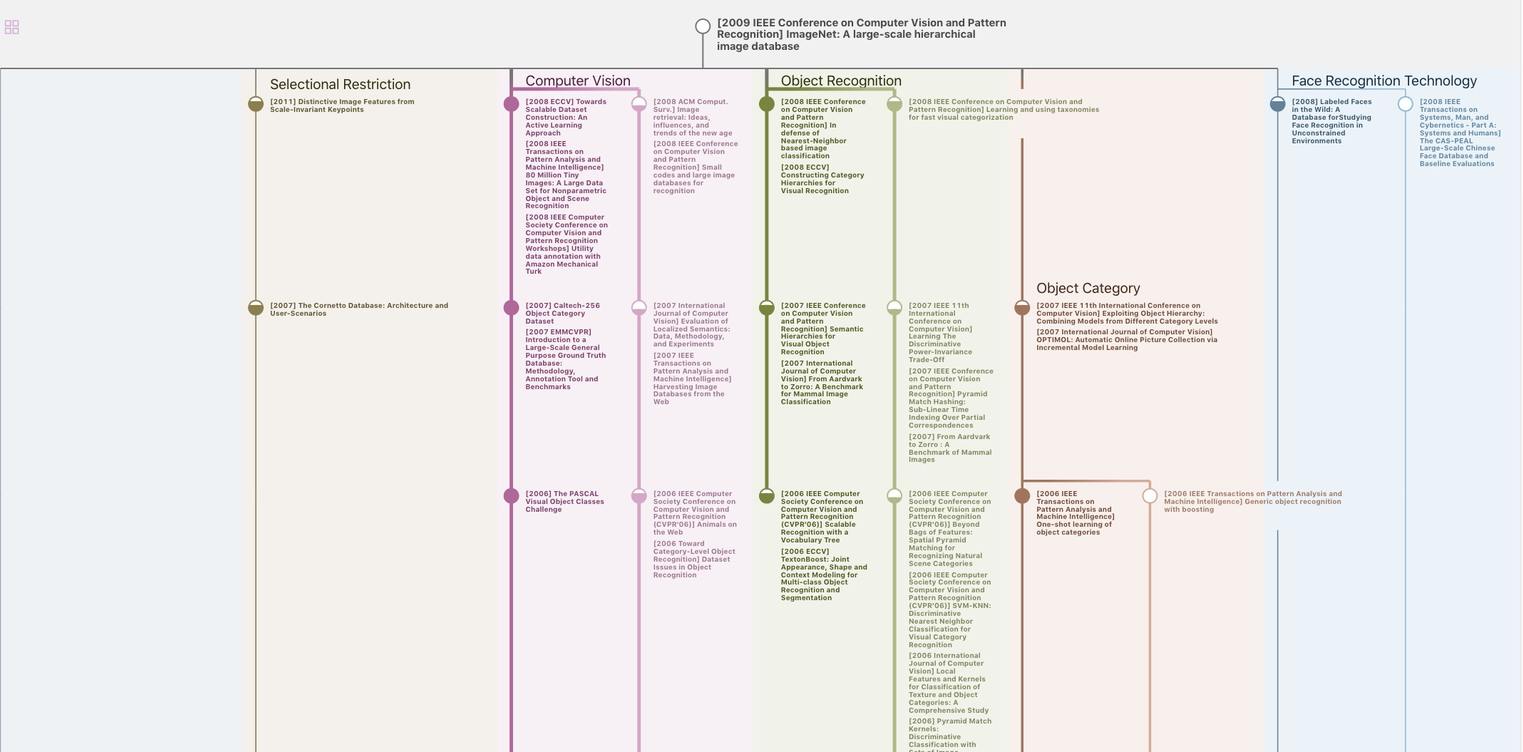
生成溯源树,研究论文发展脉络
Chat Paper
正在生成论文摘要