ICSFF: Information Constraint on Self-Supervised Feature Fusion for Few-Shot Remote Sensing Image Classification
IEEE TRANSACTIONS ON GEOSCIENCE AND REMOTE SENSING(2024)
摘要
The self-supervised few-shot remote sensing image classification task is to achieve efficient and accurate remote sensing image classification through autonomous learning and feature exploitation with limited data labels. However, at this stage, one common challenge in self-supervised learning is the significant disparity between the self-supervised learning task and the main classification task. This disparity can lead to a situation where the model overly emphasizes features or local information emphasized by the self-supervised task while neglecting the essential global semantic information relevant to the main classification task. To solve these problems, this article proposes a few-shot remote sensing image classification framework based on information constraint on self-supervised feature fusion (ICSFF). First, we train a supervised model to capture important semantic information. Then, we leverage this supervised information to constrain the learning process of the self-supervised model. We utilize an attention mechanism to integrate supervised information and self-supervised information through a graph structural feature fusion approach, resulting in enhanced feature representations. In addition, we design a new feature extractor called GCCANet. It helps the model to better utilize the key features by incorporating the global attention module, the group convolution, the residual operation, and the channel shuffle module techniques. We conduct comparative experiments on three public remote sensing datasets, and the experimental results show that ICSFF achieves outstanding performance in the remote sensing image classification task.
更多查看译文
关键词
Feature fusion,few-shot learning,information constraint,remote sensing image classification,self-supervised learning
AI 理解论文
溯源树
样例
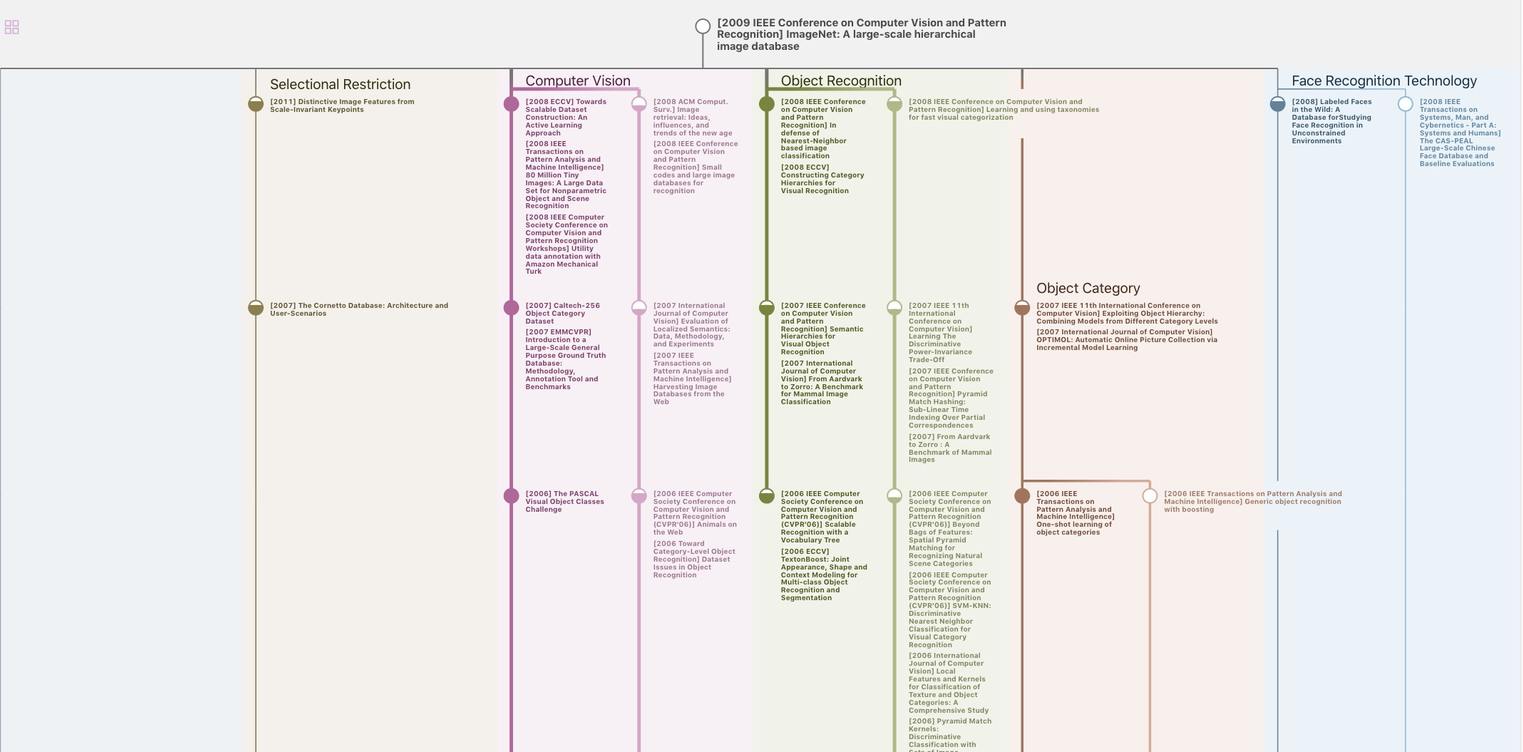
生成溯源树,研究论文发展脉络
Chat Paper
正在生成论文摘要