On Accelerating Substrate Optimization Using Computational Gibbs Energy Barriers: A Numerical Consideration Utilizing a Computational Data Set
ACS OMEGA(2024)
摘要
Substrate optimization is a time- and resource-consuming step in organic synthesis. Recent advances in chemo- and materials-informatics provide systematic and efficient procedures utilizing tools such as Bayesian optimization (BO). This study explores the possibility of reducing the required experiments further by utilizing computational Gibbs energy barriers. To thoroughly validate the impact of using computational Gibbs energy barriers in BO-assisted substrate optimization, this study employs a computational Gibbs energy barrier data set in the literature and performs an extensive numerical investigation virtually regarding the Gibbs energy barriers as virtual experimental results and those with systematic and random noises as virtual computational results. The present numerical investigation shows that even the computational reactivity affected by noises of as much as 20 kJ/mol helps reduce the number of required experiments.
更多查看译文
AI 理解论文
溯源树
样例
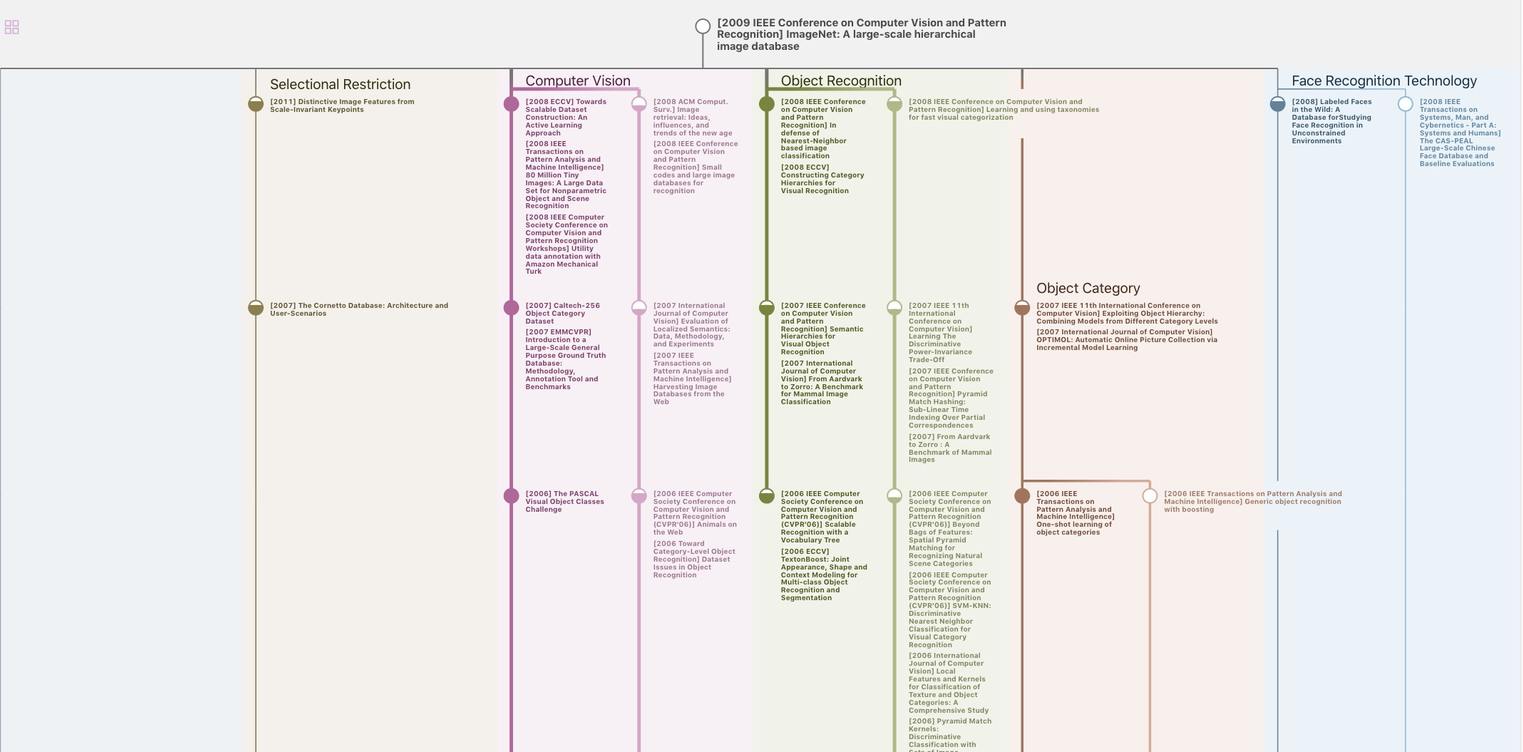
生成溯源树,研究论文发展脉络
Chat Paper
正在生成论文摘要