Machine Learning and Deep Learning Guided Assessment of Groundwater Reservoir Hydrodynamic Parameters: A Case Study of The El Haouz Aquifer
E3S Web of Conferences(2024)
摘要
The Plio-Quaternary aquifer in the EL-Haouz-Mejjate region of Morocco is critical for water supply, necessitating accurate characterization for sustainable management. This study pioneers machine learning (ML) and deep learning (DL) techniques to elucidate the aquifer’s properties. Supervised algorithms, including random forest, regression, support vector machines, Gaussian process regression and neural networks, are trained on available hydrogeological data. Diverse features capture complex input-output relationships to predict key hydrodynamic factors like hydraulic conductivity and transmissivity fields. Aquifer architecture attributes, including substratum depth, thickness, and height, are also estimated. Model outputs are validated with field measurements, demonstrating promising accuracy. Enhanced hydrodynamic insights improve the conceptual model and groundwater flow modeling confidence. Uncertainties are reduced through this data-driven approach, enabling optimized aquifer management. Overall, this work shows how useful it is to combine ML and DL with traditional hydrogeology in order to get a better understanding of complicated aquifer systems. The techniques pioneered provide a pathway for sustainable management of this vital water resource.
更多查看译文
AI 理解论文
溯源树
样例
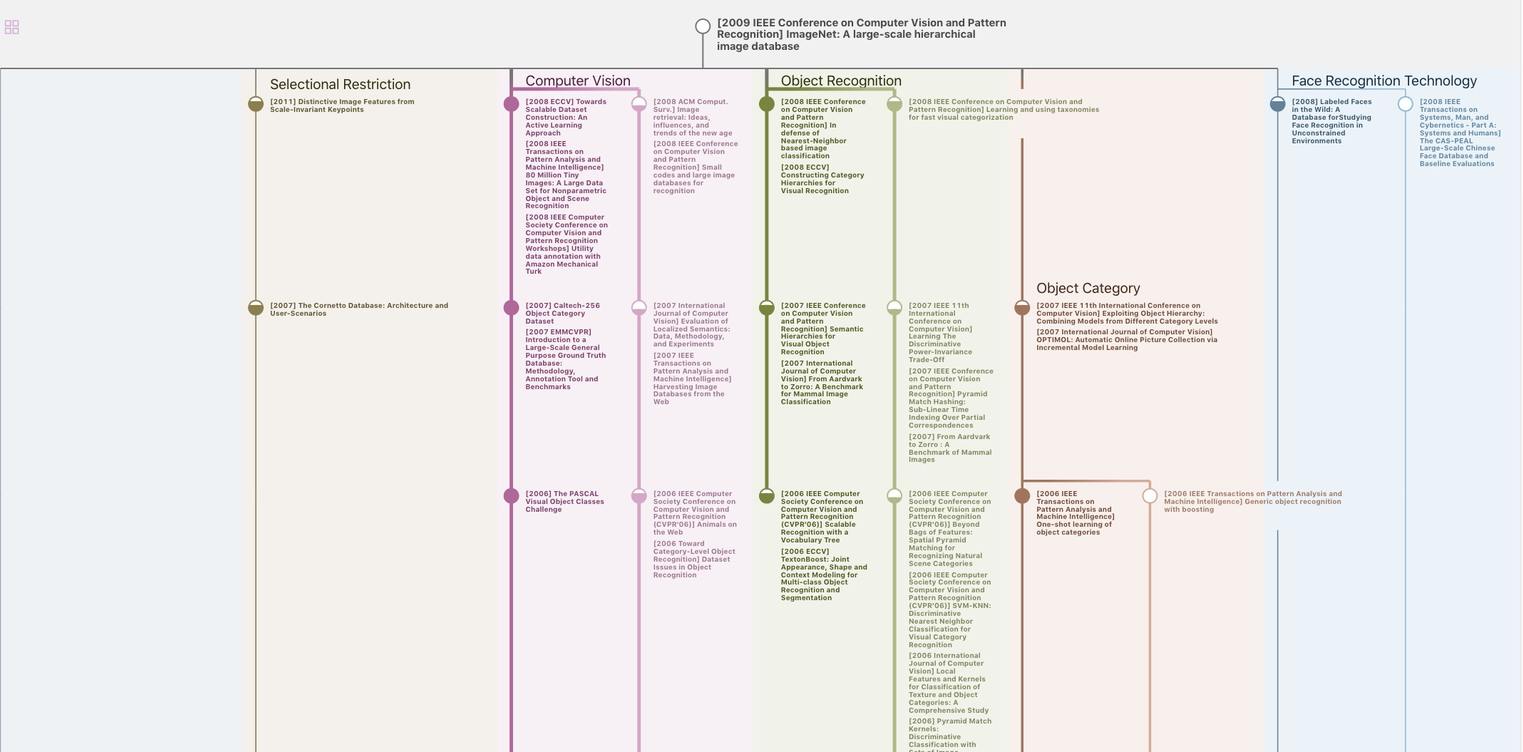
生成溯源树,研究论文发展脉络
Chat Paper
正在生成论文摘要