Effects of single and multiple imputation strategies on addressing over-fitting issues caused by imbalanced data from various scenarios
Applied Intelligence(2024)
摘要
The presence of missing values consistently emerges as a critical issue in most machine learning tasks, as they can alter the distribution of the training data and consequently lead to overfitting. The theoretical framework for missing value imputation has reached a considerable level of maturity, with numerous imputation models having been proposed. However, there has been limited research conducted on the underlying causes of missing values and scenarios where imbalanced data is significantly correlated with target variables due to business logic. In this study, we conducted simulation studies to evaluate the imputation performance of six imputation models on six datasets under three missing mechanisms, including random dropout, imbalance dropout based on features, and imbalance dropout based on labels, to identify an appropriate approach to deal with imbalanced missing data with certain patterns. By recognizing the missing pattern and imputing the data with a suitable imputation method, the overfitting issue caused by missingness has been significantly mitigated in a real-world application.
更多查看译文
关键词
Missing imputation,Imbalanced data,Simulation study
AI 理解论文
溯源树
样例
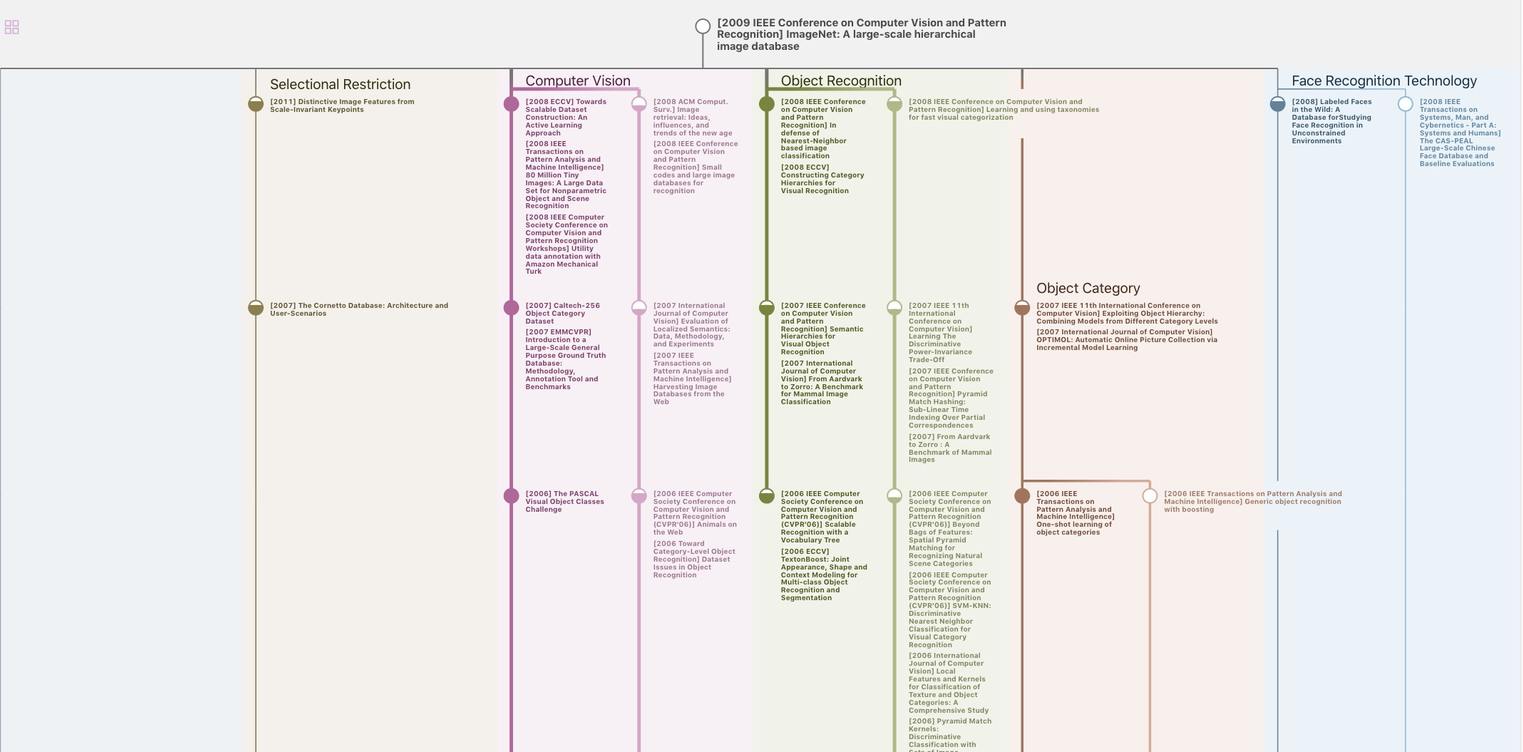
生成溯源树,研究论文发展脉络
Chat Paper
正在生成论文摘要