Ensemble learning for prominent feature selection and electric power prediction in agriculture sector
Multimedia Tools and Applications(2024)
摘要
The agricultural industry is critical to the Indian GDP, yet it faces several issues, such as energy, unemployment, and water scarcity. Among these challenges, reliable access to electricity stands out as a crucial factor for implementing smart farming techniques tailored to diverse crops and irrigation patterns. This paper addresses the intricate task of load forecasting in the agriculture sector, employing machine learning and ensemble learning methods. By scrutinizing various agroclimatic and weather characteristics, we pinpoint exogenous features influencing load prediction and prioritize their significance. The study introduces a groundbreaking approach, Temporal Adaptive Ensemble Learning (TAEL), designed to augment accuracy by incorporating temporal dynamics. Six distinct cases, varying in input parameters, are meticulously examined, employing ensemble approaches and benchmarking against Multiple Linear Regression (MLR) and K-Nearest Neighbor (KNN) models. The findings emphasize the vital role of integrating agroclimatic and time series features, showcasing notable enhancements in algorithmic performance across all cases. The proposed TAEL model stands out, achieving a remarkable mean accuracy of 87.1% and a 20.4% MAPE error. This underscores its effectiveness compared to traditional statistical and machine learning approaches, addressing limitations observed in existing studies. The research not only identifies critical factors influencing load prediction but also pioneers a novel approach, TAEL, contributing to the advancement of agricultural load forecasting methodologies.
更多查看译文
关键词
Agricultural sector ensemble learning,Feature engineering,Load forecasting,Machine learning
AI 理解论文
溯源树
样例
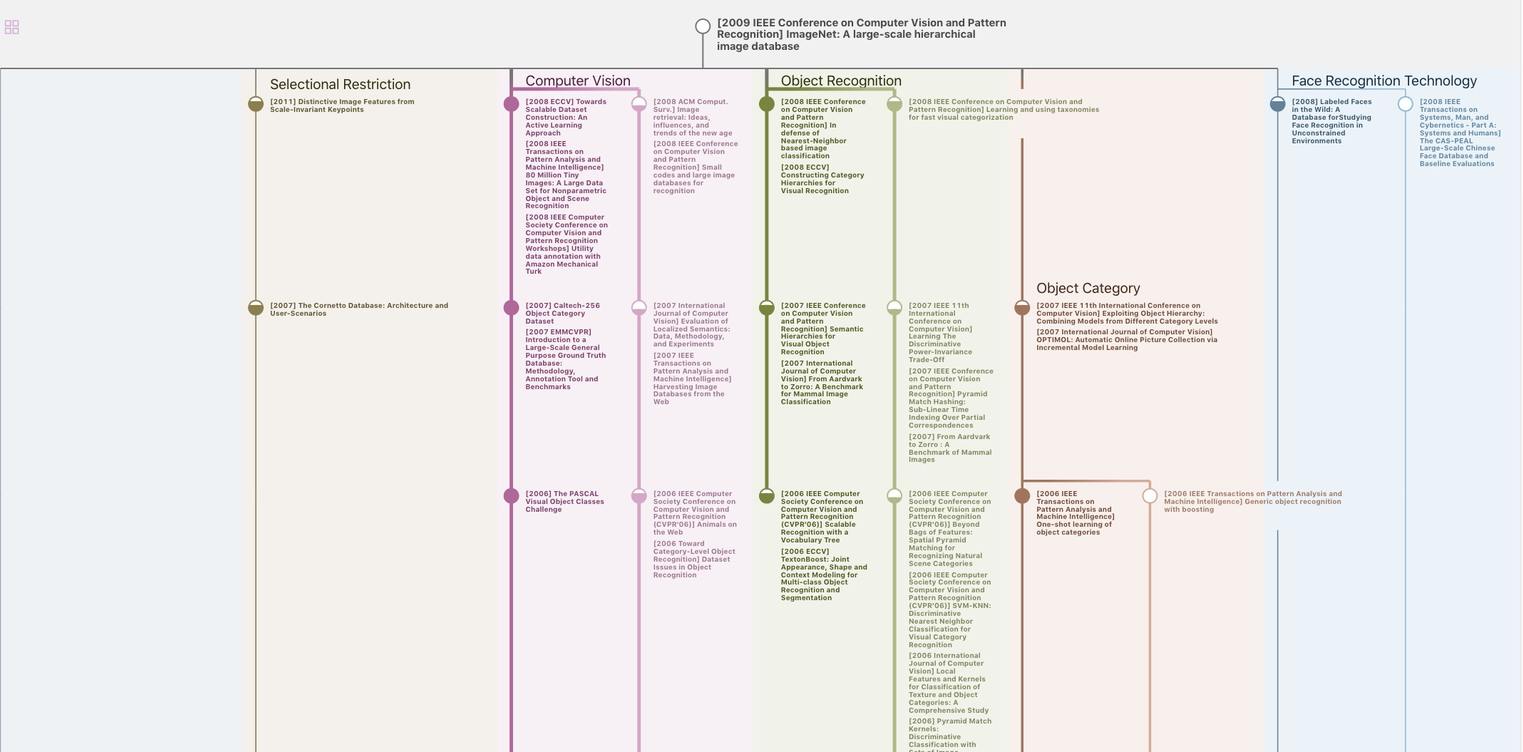
生成溯源树,研究论文发展脉络
Chat Paper
正在生成论文摘要