Improving Peer-Review Score Prediction via Pretrained Model with Intermediate-Task Training.
International Conference on Ubiquitous Information Management and Communication(2024)
摘要
Peer review plays an essential role in validating academic work and elevating the quality of published research. Developing an accurate system for predicting peer-review scores can be challenging due to the restricted annotated peer-review information. This paper presents intermediate-task training to improve the efficiency of the pretrained models. Recent research has demonstrated the efficacy of pretrained language models for downstream tasks. Applying intermediate-task training can help a model learn beneficial information before fine-tuning it on a peer-review score prediction. We also extend a pretrained model to overcome one of its primary drawbacks - application to documents that are longer than a thousand words (e.g., academic papers). Our technique is conceptually straightforward. We partition a document into sentences and feed each one into a pretrained model to acquire sentence embedding. Then, we utilize a sequence of sentence embeddings as an input of that pretrained model. The experimental results demonstrated that intermediate-task training helps increase the performance of the pretrained model on peer-review score prediction.
更多查看译文
关键词
Document Classification,Intermediate Task Training,Peer-Review Score Prediction
AI 理解论文
溯源树
样例
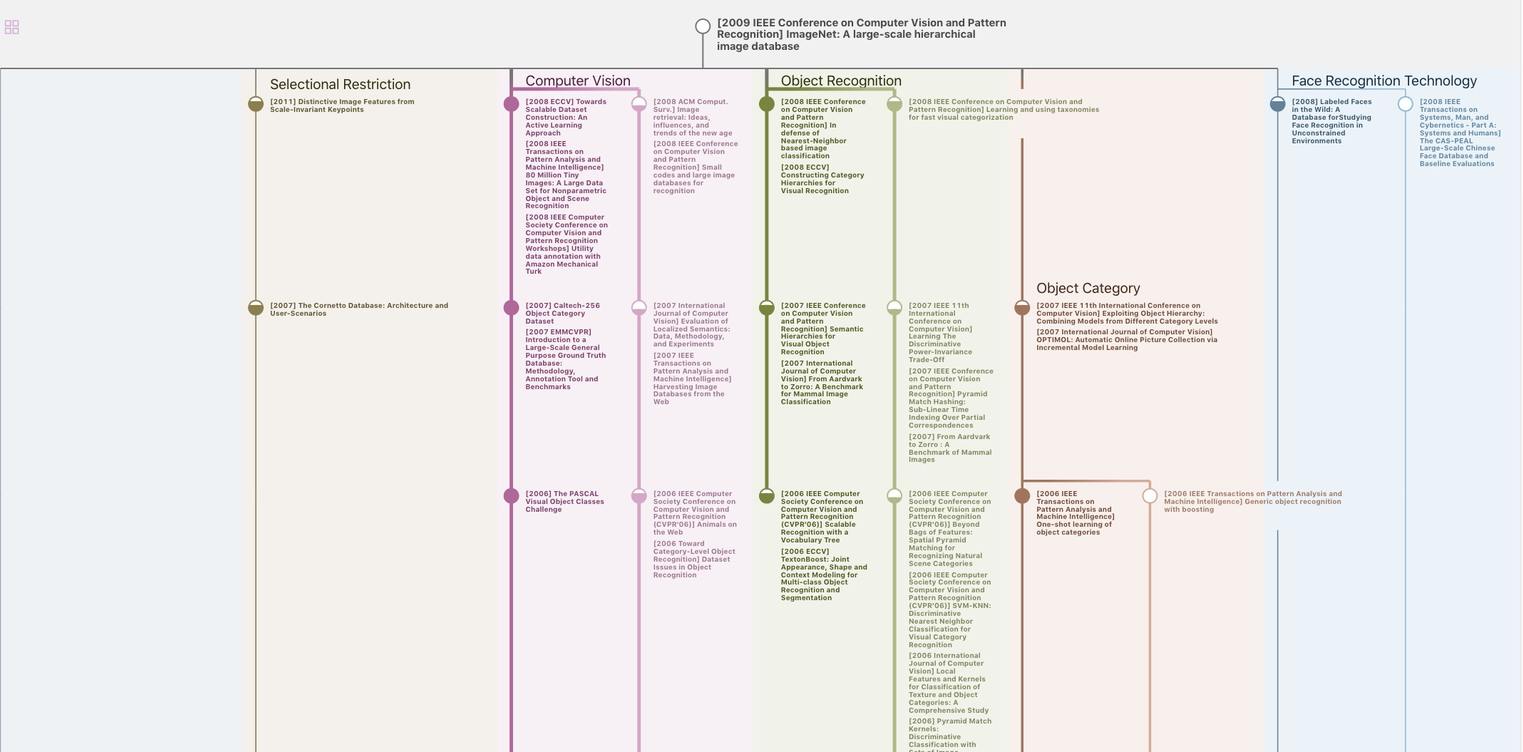
生成溯源树,研究论文发展脉络
Chat Paper
正在生成论文摘要