Few-Shot Specific Emitter Identification Method Using Rotation Feature Decoupling for Secure 6G.
International Conference on Communication Technology(2023)
摘要
Specific Emitter Identification (SEI) can be considered as a passive physical layer authentication technology for future secure six-generation (6G) wireless communications. Traditionally, Deep Learning (DL) is used for extracting deep features from Radio Frequency (RF) for identification. However, this method often encounters the challenge of limited training samples. To address this problem, we propose a Few-Shot SEI (FS-SEI) method using Rotation Feature Decoupling (RFD). Specifically, we construct a pretext task that enables an encoder to learn a split feature representation containing a rotation-relevant part and a rotation-irrelevant part from an unlabeled auxiliary dataset. In the downstream task, we fine-tune the pretrained encoder on target dataset consisting of few-shot samples. Simulation results show that the accuracy of our method can reach up to more than 90% when the number of training sample is 30 per category. The code and related dataset can be downloaded from https://github.com/IcedWatermelonJuice/FS-SEI/tree/main/Rotation-Feature-Decoupling.
更多查看译文
关键词
Specific emitter identification (SEI),few-shot learning,self-supervised learning,rotation prediction
AI 理解论文
溯源树
样例
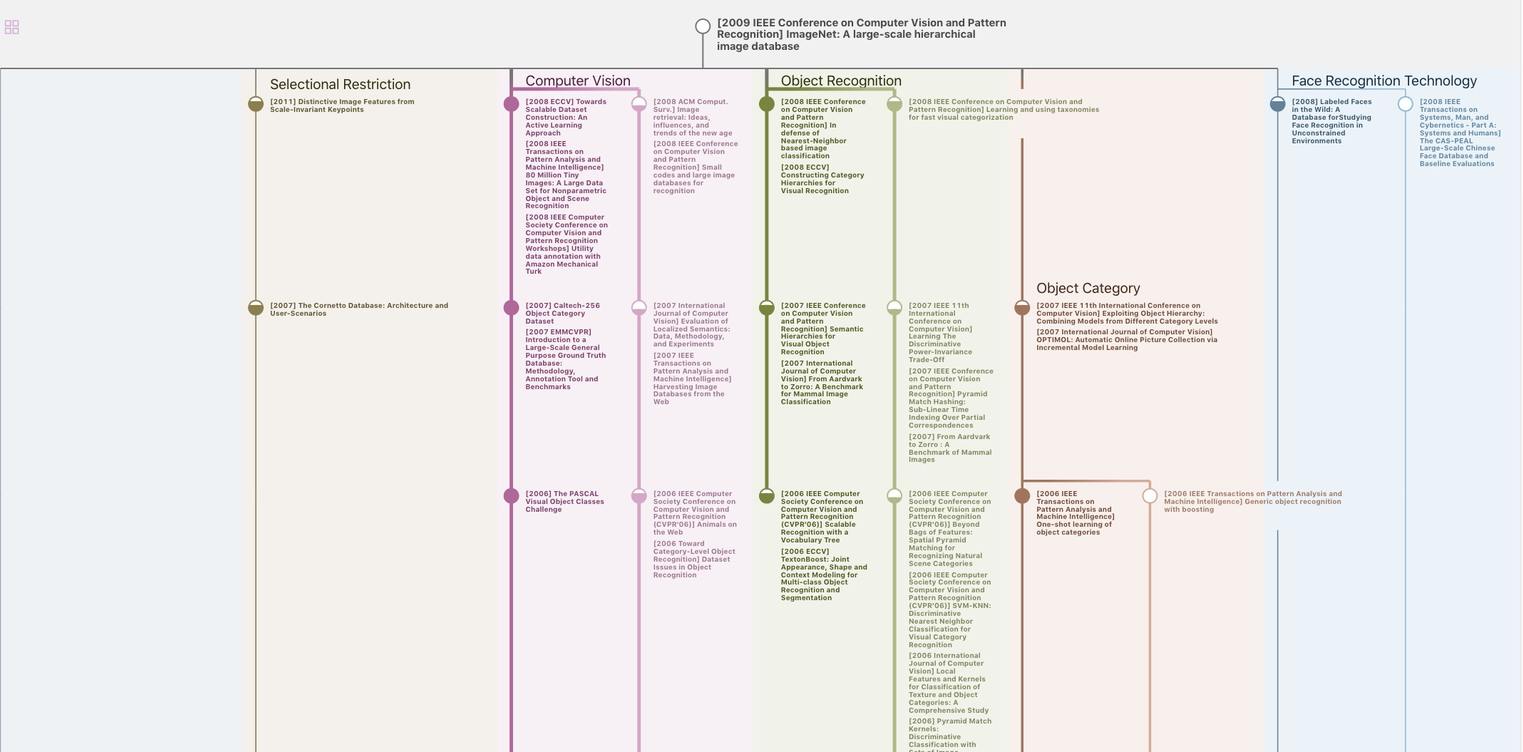
生成溯源树,研究论文发展脉络
Chat Paper
正在生成论文摘要