Service Decision Mechanism Based On Traffic Flow Prediction in the Internet of Vehicles.
International Conference on Communication Technology(2023)
摘要
The service time of Road-Side Units (RSUs) for vehicle users will directly affect the Quality of Service (QoS) in the Internet of Vehicles (IoV), and it is related to the traffic flow and service decisions. Consequently, a sophisticated service decision mechanism based on the traffic flow prediction is necessary beforehand in order to optimize QoS. In this work, we propose a service decision based on traffic flow prediction to reduce the service waiting time of vehicles. Firstly, we propose a traffic flow prediction model based on deep learning. Convolutional Neural Network (CNN) is used to capture spatial data in order to handle the complicated road network layout in metropolitan roads. First, to reveal people's travel patterns, temporal features are captured by two different models. While short-term features are captured from historical data by Auto-Encoder, daily period feature as well as weekly period feature are captured from bi-directional data by Bidirectional Long Short-Term Memory (Bi-LSTM). Second, by simulating the process of RSUs serving vehicles using a queuing theory model, we propose a service decision mechanism based on the anticipated traffic flow. The proposed service decision mechanism aims to reduce the average waiting time of vehicles for service. Particularly, the RSUs will ask the Macro Base Station (MBS) for assistance when there are too many vehicles traveling through the RSUs. Finally, we conduct comprehensive experiments using real data to evaluate the model performance, and it is demonstrated that our proposed scheme outperforms other benchmarks.
更多查看译文
关键词
Internet of Vehicles,traffic flow prediction,service decision mechanism,queuing theory
AI 理解论文
溯源树
样例
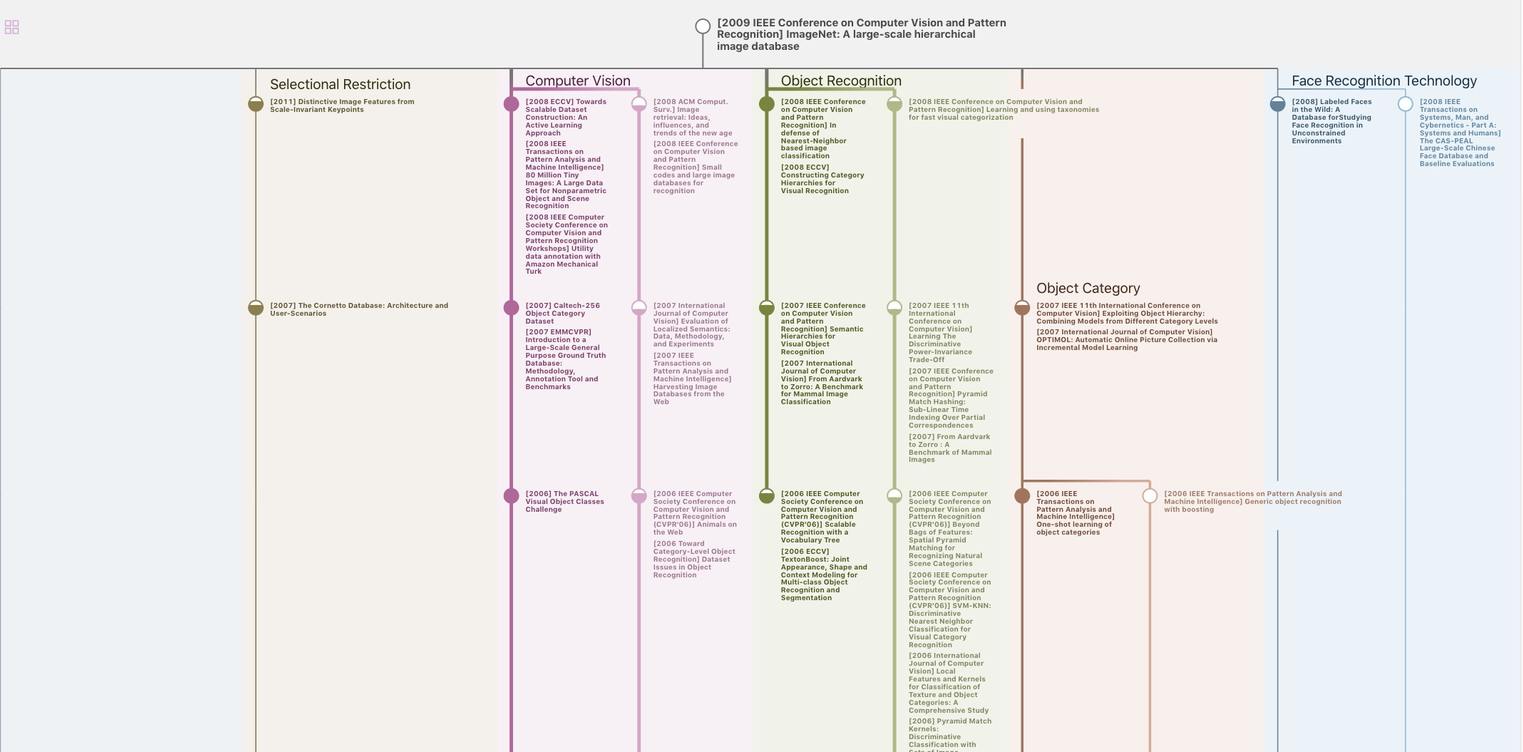
生成溯源树,研究论文发展脉络
Chat Paper
正在生成论文摘要