Echocardiographic artificial intelligence for pulmonary hypertension classification
HEART(2024)
摘要
Objective The classification of pulmonary hypertension (PH) is crucial for determining the appropriate therapeutic strategy. We investigated whether machine learning (ML) algorithms may assist in echocardiographic PH prediction, where current guidelines recommend integrating several different parameters. Methods We obtained physical and echocardiographic data from 885 patients who underwent right heart catheterisation (RHC). Patients were classified into three groups: non-PH, precapillary PH and postcapillary PH, based on values obtained from RHC. Using 24 parameters, we created predictive models employing four different classifiers and selected the one with the highest area under the curve. We then calculated the macro-average classification accuracy for PH on the derivation cohort (n=720) and prospective validation data set (n=165), comparing the results with guideline-based echocardiographic assessment obtained from each cohort. Results Logistic regression with elastic net regularisation had the highest classification accuracy, with areas under the curves of 0.789, 0.766 and 0.742 for normal, precapillary PH and postcapillary PH, respectively. The ML model demonstrated significantly better predictive accuracy than the guideline-based echocardiographic assessment in the derivation cohort (59.4% vs 51.6%, p<0.01). In the independent validation data set, the ML model's accuracy was comparable to the guideline-based PH classification (59.4% vs 57.8%, p=0.638). Conclusions This preliminary study suggests promising potential for our ML model in predicting echocardiographic PH. Further research and validation are needed to fully assess its clinical utility in PH diagnosis and treatment decision-making.
更多查看译文
关键词
Echocardiography,Heart Failure, Diastolic,Hypertension, Pulmonary
AI 理解论文
溯源树
样例
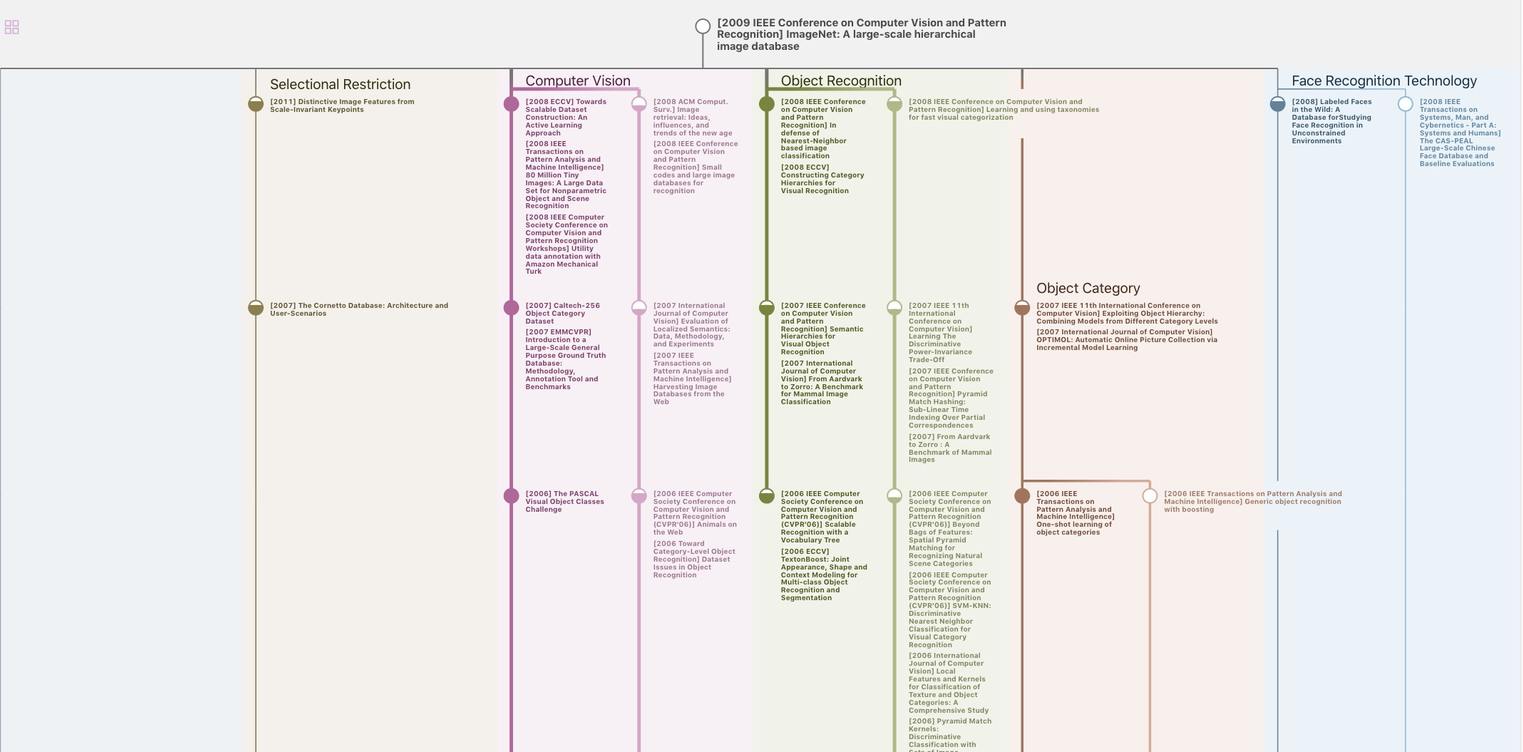
生成溯源树,研究论文发展脉络
Chat Paper
正在生成论文摘要