Multimodality radiomics for tumor prognosis in nasopharyngeal carcinoma
PLOS ONE(2024)
摘要
Background The prognosis of nasopharyngeal carcinoma (NPC) is challenging due to late-stage identification and frequently undetectable Epstein-Barr virus (EBV) DNA. Incorporating radiomic features, which quantify tumor characteristics from imaging, may enhance prognosis assessment. Purpose To investigate the predictive power of radiomic features on overall survival (OS), progression-free survival (PFS), and distant metastasis-free survival (DMFS) in NPC. Materials and methods A retrospective analysis of 183 NPC patients treated with chemoradiotherapy from 2010 to 2019 was conducted. All patients were followed for at least three years. The pretreatment CT images with contrast medium, MR images (T1W and T2W), as well as gross tumor volume (GTV) contours, were used to extract radiomic features using PyRadiomics v.2.0. Robust and efficient radiomic features were chosen using the intraclass correlation test and univariate Cox proportional hazard regression analysis. They were then combined with clinical data including age, gender, tumor stage, and EBV DNA level for prognostic evaluation using Cox proportional hazard regression models with recursive feature elimination (RFE) and were optimized using 20 repetitions of a five-fold cross-validation scheme. Results Integrating radiomics with clinical data significantly enhanced the predictive power, yielding a C-index of 0.788 +/- 0.066 to 0.848 +/- 0.079 for the combined model versus 0.745 +/- 0.082 to 0.766 +/- 0.083 for clinical data alone (p<0.05). Multimodality radiomics combined with clinical data offered the highest performance. Despite the absence of EBV DNA, radiomics integration significantly improved survival predictions (C-index ranging from 0.770 +/- 0.070 to 0.831 +/- 0.083 in combined model versus 0.727 +/- 0.084 to 0.734 +/- 0.088 in clinical model, p<0.05). Conclusions The combination of multimodality radiomic features from CT and MR images could offer superior predictive performance for OS, PFS, and DMFS compared to relying on conventional clinical data alone.
更多查看译文
AI 理解论文
溯源树
样例
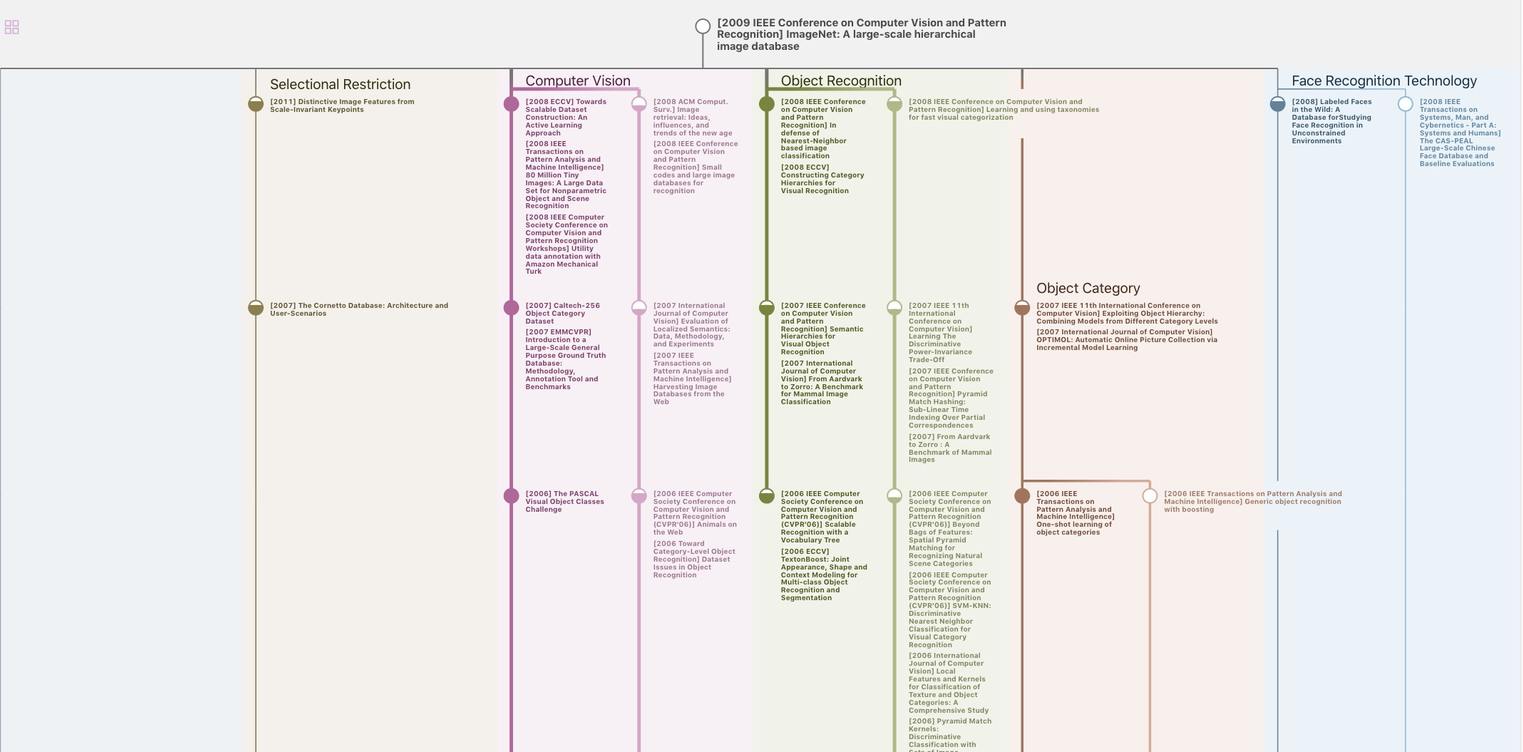
生成溯源树,研究论文发展脉络
Chat Paper
正在生成论文摘要