NoSimple: Data Bias Evaluation Metrics.
International Conference on Ubiquitous Information Management and Communication(2024)
摘要
Simple objects are defined as objects invariably correctly classified by all outlier detectors. Its presence impairs performance of binary classifiers such as ROC or F1 score. A large number of simple objects falsely improve performance of binary classifiers when evaluated by ROC or F1 score. This impairs reliability of classifier evaluation. This manuscript proposes evaluation without simple objects (NoSimple). NoSimple preprocesses data to factor in simple objects by removing the simple objects for the evaluation phase. Experiments with 30 realworld datasets demonstrate that NoSimple significantly reduced the average ROC of all classifiers by
$0.04 \sim 0.06$
. NoSimple is most effective when the percentage of simple objects exceeds
$30{\% }$
. By introducing a new method to reliably evaluate outlier classifiers, NoSimple has the potential to revolutionize evaluation metrics and has a multitude of applications in data science research.
更多查看译文
关键词
evaluation metric,ROC,F1 score,NoSimple,evaluation without simple objects,outlier detection,CTR
AI 理解论文
溯源树
样例
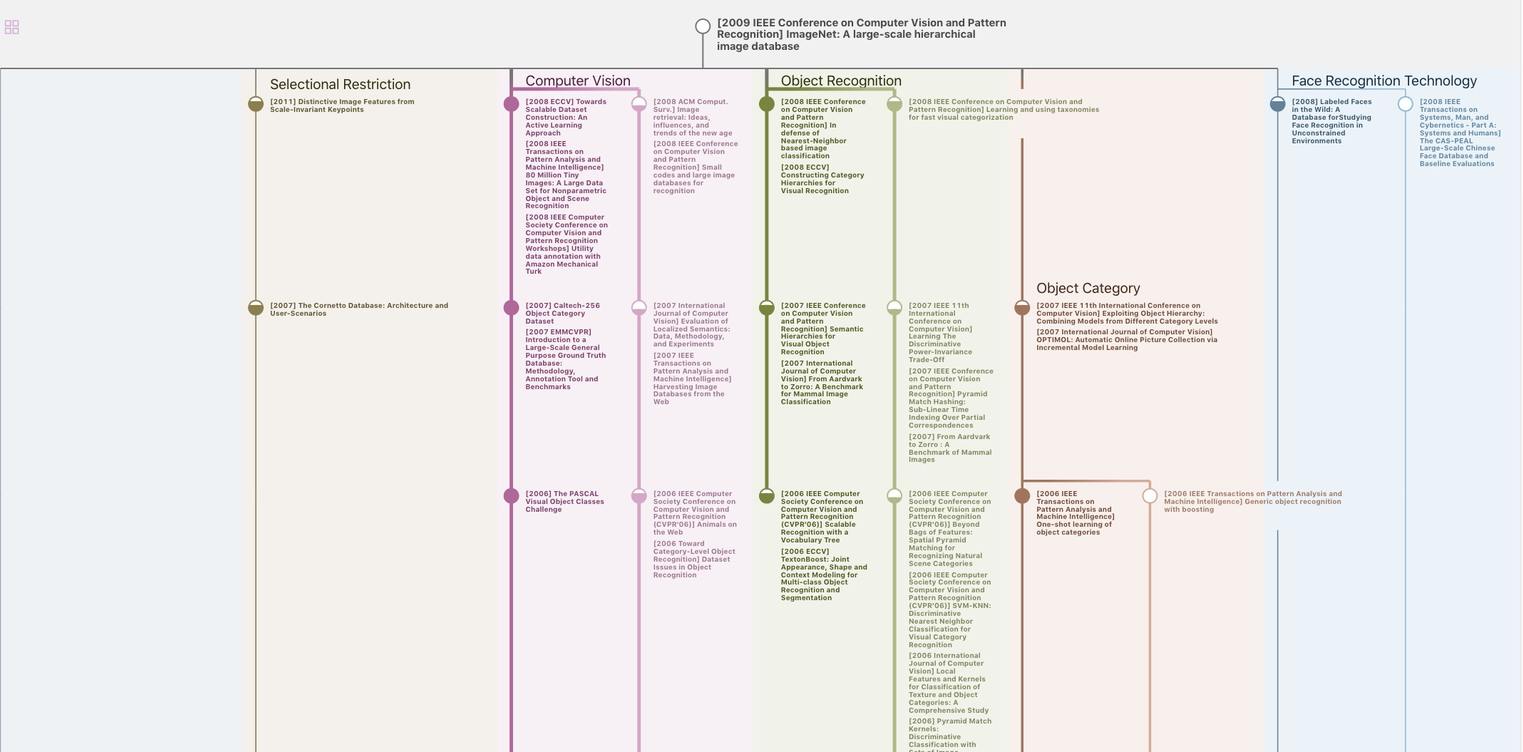
生成溯源树,研究论文发展脉络
Chat Paper
正在生成论文摘要