Avoiding Catastrophe in Continuous Spaces by Asking for Help
CoRR(2024)
摘要
Most reinforcement learning algorithms with formal regret guarantees assume
all mistakes are reversible and rely on essentially trying all possible
options. This approach leads to poor outcomes when some mistakes are
irreparable or even catastrophic. We propose a variant of the contextual bandit
problem where the goal is to minimize the chance of catastrophe. Specifically,
we assume that the payoff each round represents the chance of avoiding
catastrophe that round, and try to maximize the product of payoffs (the overall
chance of avoiding catastrophe). To give the agent some chance of success, we
allow a limited number of queries to a mentor and assume a Lipschitz continuous
payoff function. We present an algorithm whose regret and rate of querying the
mentor both approach 0 as the time horizon grows, assuming a continuous 1D
state space and a relatively "simple" payoff function. We also provide a
matching lower bound: without the simplicity assumption: any algorithm either
constantly asks for help or is nearly guaranteed to cause catastrophe. Finally,
we identify the key obstacle to generalizing our algorithm to a
multi-dimensional state space.
更多查看译文
AI 理解论文
溯源树
样例
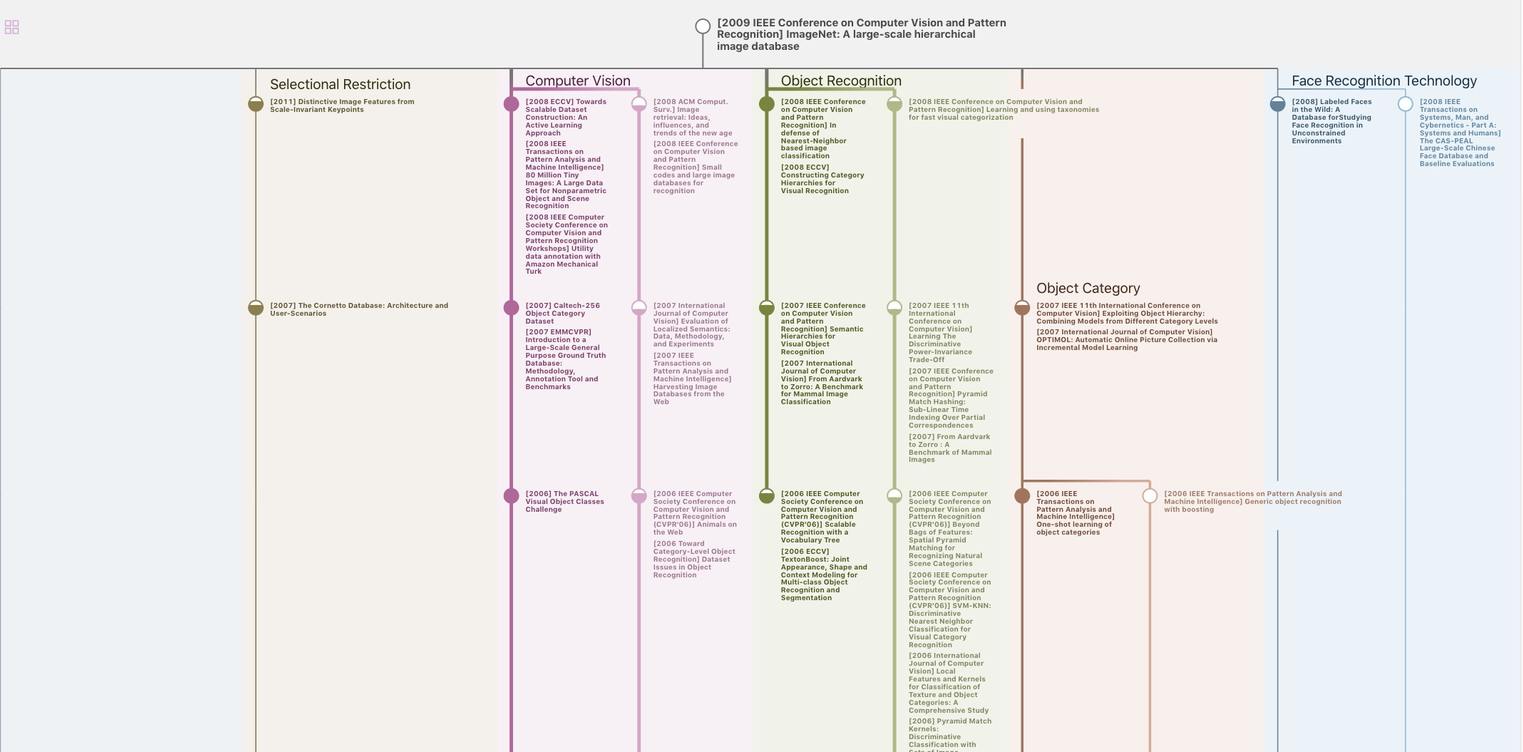
生成溯源树,研究论文发展脉络
Chat Paper
正在生成论文摘要