LCFNet: Loss Compensation Fusion Network for Real-time Semantic Segmentation of Urban Road Scenes
2023 IEEE 26TH INTERNATIONAL CONFERENCE ON INTELLIGENT TRANSPORTATION SYSTEMS, ITSC(2023)
摘要
Semantic segmentation is used by intelligent transportation systems to understand and sense the traffic environment. However, achieving semantic segmentation in realtime is a challenge due to the necessity of both high accuracy and fast processing. This is especially valuable for applications such as autonomous driving and industrial robotics. In this paper, we propose a real-time semantic segmentation network, called LCFNet, which makes use of three-branch structure. The LCFNet consists of Lightweight Detail Guidance Fusion (L-DGF) and Lightweight Semantic Guidance Fusion (L-SGF) modules. Both modules aggregate information from various network layers. In the termination of network, a Total Guidance Fusion (TGF) module is proposed for processing information from all three branches. Depth-wise Convolution Pyramid Pooling (DCPP) module is also included to optimize accuracy and simplify computation. The effectiveness of LCFNet is demonstrated on two typical semantic segmentation datasets, Cityscapes and CamVid. On a single NVIDIA GeForce GTX 2080 Ti GPU, LCFNet reaches 77.02% mIoU at 95.97 FPS and 81.17% mIoU at 204.82 FPS, respectively.
更多查看译文
关键词
Semantic Segmentation,Semantic Segmentation Network,Real-time Semantic Segmentation,Intelligent Transportation Systems,Pyramid Pooling,Depthwise Convolution,Pyramid Pooling Module,Convolutional Neural Network,Image Features,Feature Maps,Image Dataset,Receptive Field,Real-time Performance,Semantic Information,Stochastic Gradient Descent,Batch Normalization,Segmentation Accuracy,Inference Time,Global Average Pooling,Output Of Module,Inference Speed,Lightweight Convolutional Neural Network,Pointwise Convolution,Feature Pooling,Semantic Segmentation Task,Feature Map Size
AI 理解论文
溯源树
样例
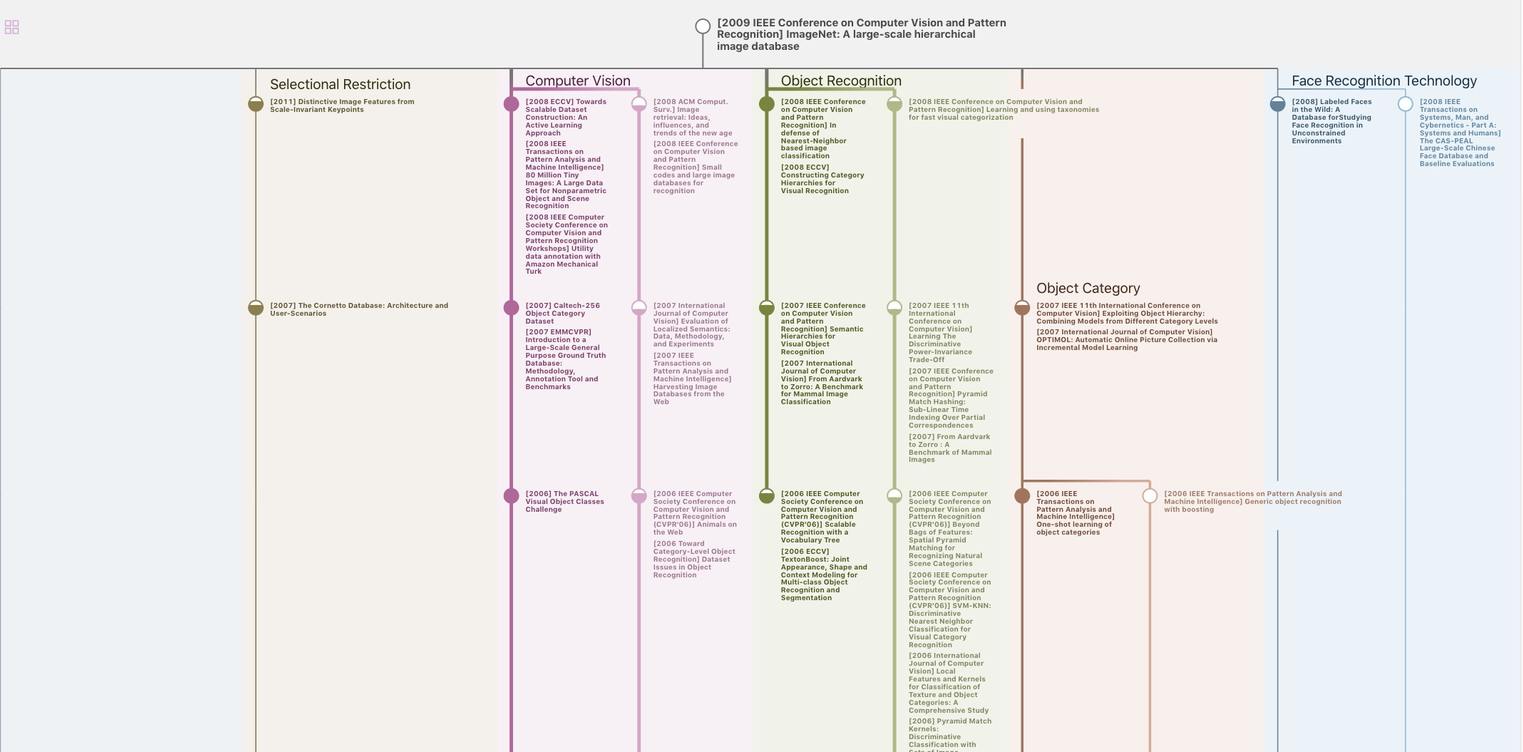
生成溯源树,研究论文发展脉络
Chat Paper
正在生成论文摘要