Extraction of vehicle behaviors at intersections.
2023 IEEE 26th International Conference on Intelligent Transportation Systems (ITSC)(2023)
摘要
Simulation of realistic urban environments is essential for the validation of decision-making algorithms in automated driving. To populate such spaces with independent agents that execute trajectories and that correctly interact with each other it is necessary to identify all possible behaviors that may arise from a set of real users trajectories. Using a two-step clustering process on trajectories with different duration, first on the position vector and then on the longitudinal velocity and acceleration, a number of behavior profiles can be identified per trajectory in intersections. To speed up the maneuver identification, the position clustering is executed on the dissimilarity matrix created using the dynamic time warp (DTW) measure between trajectories. As for the second clustering, the use of both longitudinal velocity and acceleration allows the representation of specific behaviors for each possible path. In both procedures the k-means clustering with DTW dissimilarity measure was used, combined with the Davies- Boudin score to define the optimal number of clusters. The final result provides the identification of behaviors in different geometric dispositions independently from map information and keeping the explainability property concerning each cluster.
更多查看译文
关键词
Position Vector,Dissimilarity Matrix,Map Information,Behavioral Profiles,Independent Agency,Longitudinal Velocity,Dynamic Time Warping,Time Series,Centroid,Distancing Measures,Pedestrian,Cluster Centers,Triangle Inequality,Number Of Trajectories,Road Users,Fuzzy C-means,Set Of Series,Automated Vehicles,Rand Index,Gap Statistic,Davies-Bouldin Index,Cluster Operator,Trajectory Dataset,Series Of Sizes
AI 理解论文
溯源树
样例
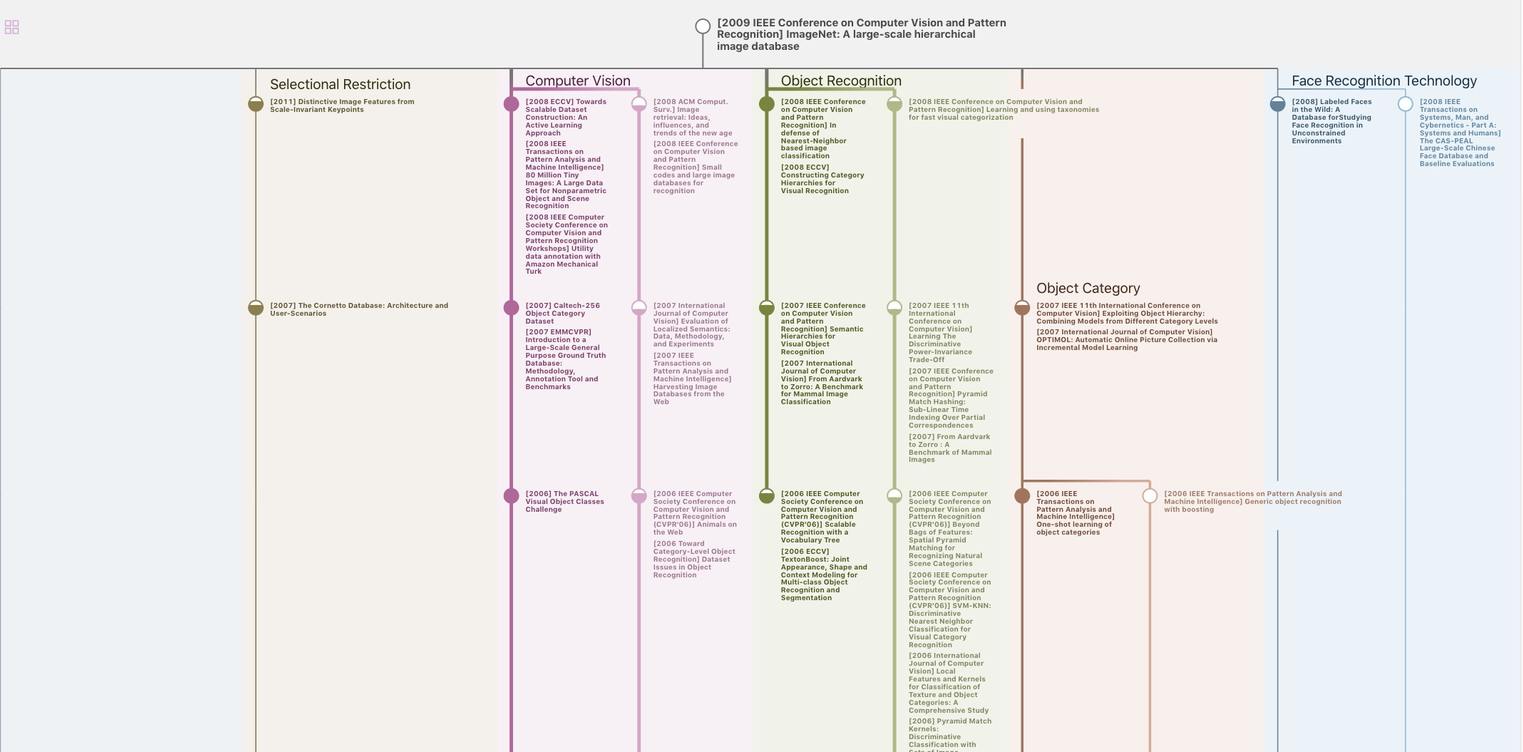
生成溯源树,研究论文发展脉络
Chat Paper
正在生成论文摘要