A Data-Driven Long-Term Prediction Method of Mandatory and Discretionary Lane Change Based on Transformer.
2023 IEEE 26th International Conference on Intelligent Transportation Systems (ITSC)(2023)
摘要
Current common choices of data-driven lane change predicting targets are left lane change, right lane change and car-following. However, to achieve accurate long-term lane change prediction, the motivation behind drivers' lane changes must also be considered. Motivated by this necessary consideration, we propose a Lane Change Attention Model (LCAM). Unlike past data-driven lane change prediction models, LCAM applied mandatory lane change (MLC), discretionary lane change (DLC) and lane-keeping (LK) as predicted driving states instead of left lane change, right lane change and car-following. This approach expands the prediction horizon of LCAM, as left/right lane changes and car-following are merely external manifestations of the process of achieving strategic driving targets. By imitating human drivers' prediction and attention mechanisms towards surrounding vehicles and road information while driving, LCAM applies Transformer architecture to solve the lane change prediction problem. Considering the specificity of lane change prediction, we specially design a route information embedding module. With its contribution, LCAM achieves accurate long-term lane change prediction.
更多查看译文
关键词
Lane change prediction,Transformer,Mandatory lane change,Discretionary lane change
AI 理解论文
溯源树
样例
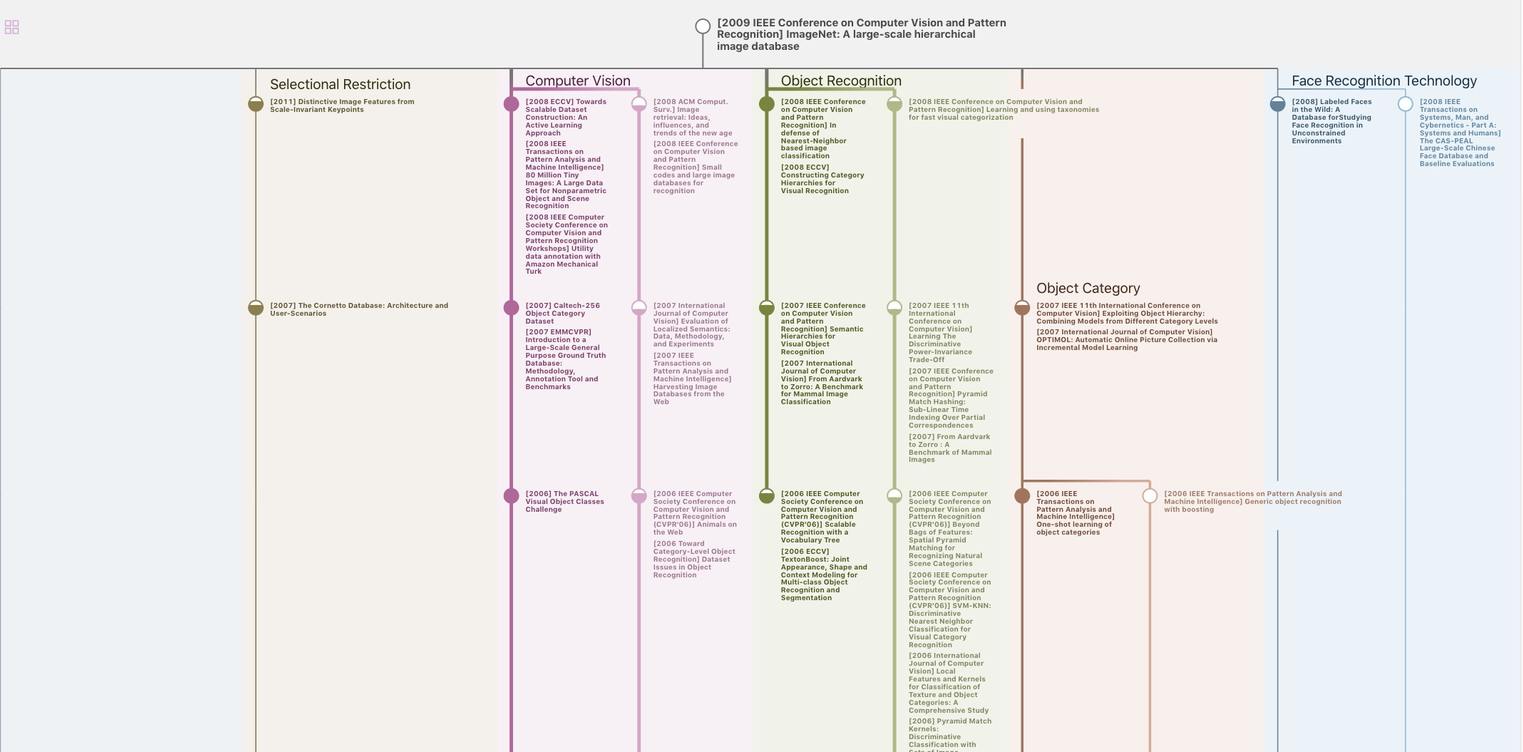
生成溯源树,研究论文发展脉络
Chat Paper
正在生成论文摘要