Multi-Task-Based Spatio-Temporal Generative Inference Network for Predicting Highway Traffic Speed.
2023 IEEE 26th International Conference on Intelligent Transportation Systems (ITSC)(2023)
摘要
Vehicles and traffic management departments have a strong desire to know the traffic speed on the high-way network in a certain future time. A multi-task-based spatio-temporal generative inference network (MT-STGIN) is proposed to predict highway traffic speed in this paper. MT-STGIN can handle the following three challenges: (1) dynamic spatio-temporal correlations, (2) prediction error propagation elimination, and (3) traffic speed heterogeneity on the highway network. First, the encoder is used to extract the dynamic spatio-temporal correlations of the highway network. Second, the decoder concentrates on correlating historical and target sequences and generates the target hidden outputs rather than a dynamic step-by-step decoding way. Finally, a multi-task learning method is used to predict traffic speed on different types of roads because of heterogeneity and shares the underlying network parameters. The evaluation experiments demonstrate that the performance of the proposed prediction model is better than that of the baselines, which are conducted based on the monitoring data of the highway in Yinchuan City, Ningxia Province, China.
更多查看译文
关键词
Traffic Flow,Development Program Of China,Error Propagation,Multi-task Learning,Dynamic Correlation,Spatiotemporal Correlation,Historical Sequence,Highway Network,Spatiotemporal Network,Ningxia Province,Multi-task Learning Method,Neural Network,Time Step,Convolutional Neural Network,Support Vector Machine,Temporal Dimension,Mean Absolute Error,Recurrent Neural Network,Spatial Dimensions,Temporal Correlation,Traffic Prediction,Traffic Data,Road Segments,Graph Neural Networks,Long-term Prediction,Mean Absolute Percentage Error,Speed Prediction,Gated Recurrent Unit,Temporal Attention,Urban Network
AI 理解论文
溯源树
样例
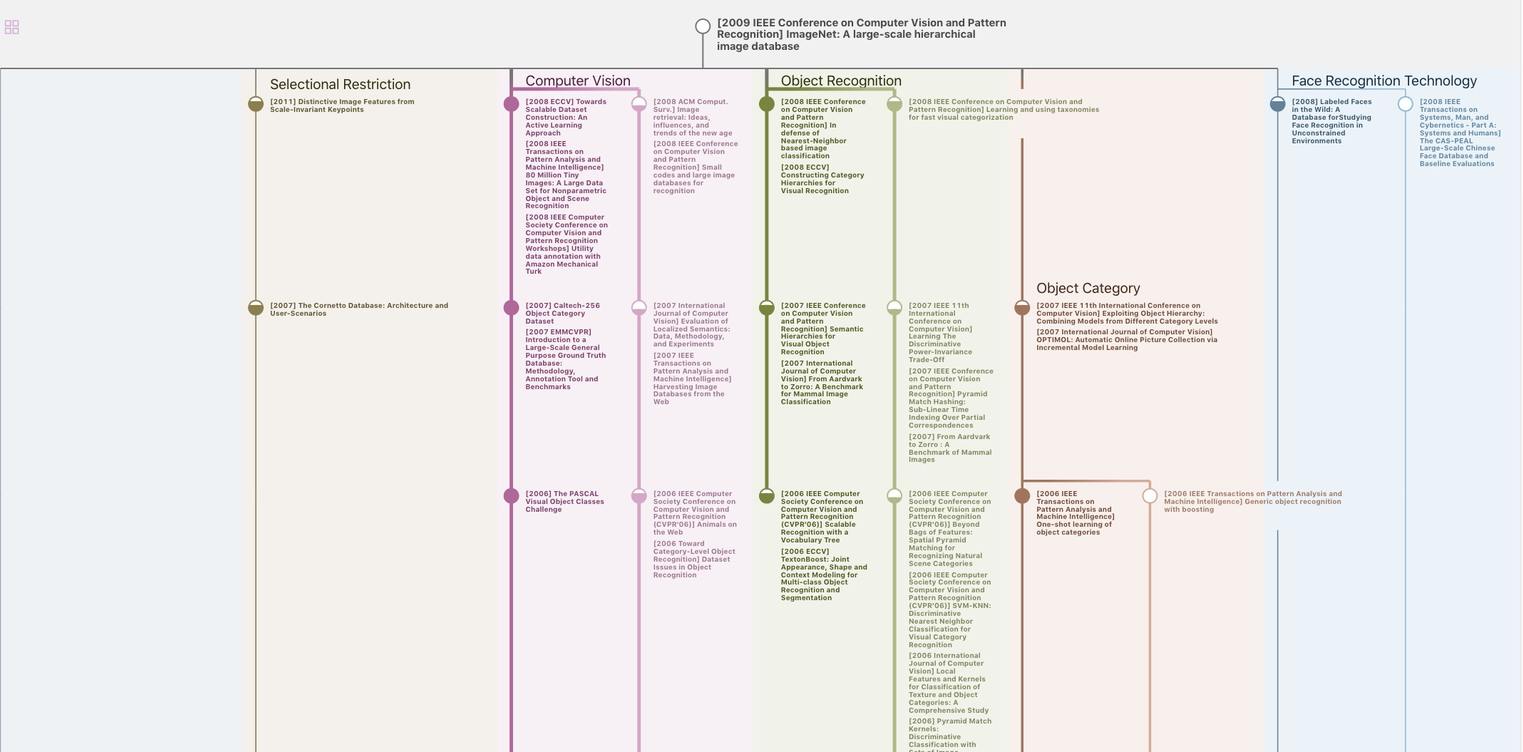
生成溯源树,研究论文发展脉络
Chat Paper
正在生成论文摘要