vLOFT: Variable Learning Rate for Online Federated Traffic Prediction.
2023 IEEE 26th International Conference on Intelligent Transportation Systems (ITSC)(2023)
摘要
Deep learning (DL) models have established remarkable success in traffic state estimation (TSE); however, the widely-studied approaches' centralized nature presents deployment challenges in Intelligent Transportation Systems (ITS), especially regarding data ownership, privacy, and response times. Online models trained using Federated Learning (FL) approaches can harvest the rich real-time data streams in ITS for continuously updating the TSE models. Though, there are limitations during the online learning process, such as model drift. In this paper, we propose a variable learning rate (VLR) scheduling approach that integrates online FL with cooperative edge computing (CEC). Continuously collected local traffic data by roadside units (RSUs) and connected sensors train localized models, and only the updated model parameters are shared instead of raw data. VLR adjustments within the Adaptive Gradient Algorithm (ADAM) optimizer framework adjust the learning rate based on traffic patterns. We conduct extensive experimental analysis and compare example VLR strategies with a control model. The results indicate that the best-performing VLR strategy reduced the average mean absolute error (MAE) across all detectors by about 10.29%.
更多查看译文
关键词
Rate Variability,Learning Rate,Traffic Prediction,Variable Learning Rate,Deep Learning,Deep Learning Models,Data Privacy,Mean Absolute Error,Online Learning,Data Streams,Parameter Update,Edge Computing,Traffic Data,Data Owner,Intelligent Transportation Systems,Federated Learning,Average Absolute Error,Roadside Units,Learning Rate Schedule,Real-time Streaming,Global Model,Long Short-term Memory,Traffic Management,Current Round,Adaptive Learning Rate,Local Training,Prediction Model,Inference Accuracy,Traffic Flow,Root Mean Square Error
AI 理解论文
溯源树
样例
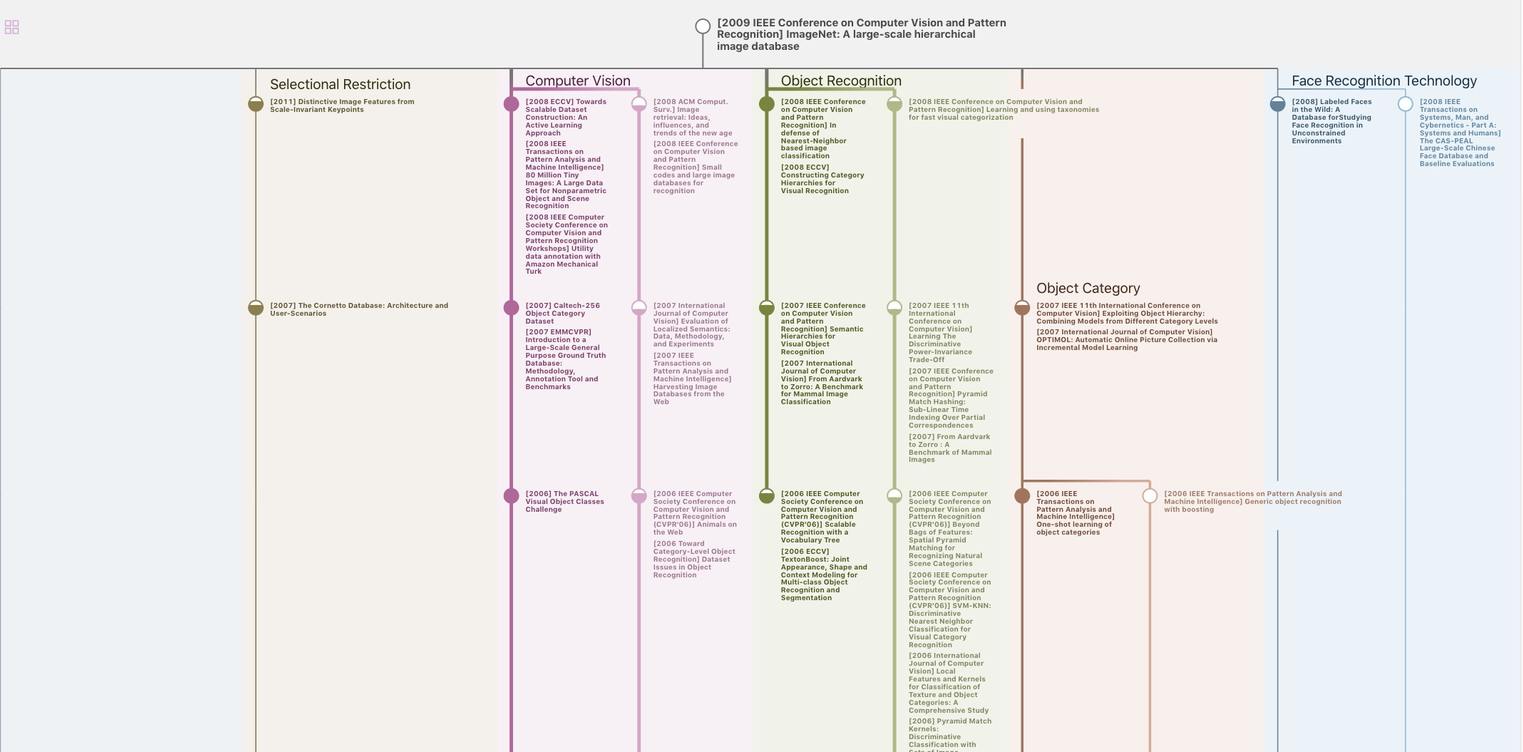
生成溯源树,研究论文发展脉络
Chat Paper
正在生成论文摘要