Adverse drug reaction prediction using voting ensemble training approach
AUT Journal of Mathematics and Computing(2024)
摘要
Identifying and controlling adverse drug reactions (ADRs) is a challenging problem in the pharmacological field. For instance, the drug Rosiglitazone has been associated with adverse reactions that were only recognized after its release. Due to such experiences, pharmacists are now more interested in using computational methods to predict ADRs. The performance of computational methods is contingent upon the defined dataset. In some studies, the known drug-adverse reaction associations are regarded as positive while the unknown drug-adverse reaction associations are regarded as negative data. This consequently creates an unbalanced dataset, which can lead to inaccurate predictions from models and cause the classifiers to be flawed. We propose a framework named Adverse Drug Reaction using the Voting Ensemble Training Approach (ADRP-VETA) for ADR problem to overcome unbalanced dataset challenges. We construct the similarity vector of each drug with other drugs based on chemical structure as a drug feature. Also, the similarity vector of each ADR with other ADRs is computed based on the Unified Medical Language System (UMLS) as adverse reaction feature. With this approach, we can leverage the similarity of the features to more accurately capture the intricate relationships between drugs and adverse reactions. We compare ADRP-VETA to three state-of-the-art models and find that it outperforms them, achieving an AUC-ROC of 91% and an AUC-PR of 89.8%. Furthermore, we assess ADRP-VETA’s ability to predict rare adverse reactions, and find that its AUC-ROC and AUC-PR are 83.3% and 92.2%, respectively. As a case study, we focus on the associations between liver-injury adverse reactions and three drugs.
更多查看译文
关键词
adverse drug reaction,machine learning,random forest,rare adverse reactions,unbalanced dataset
AI 理解论文
溯源树
样例
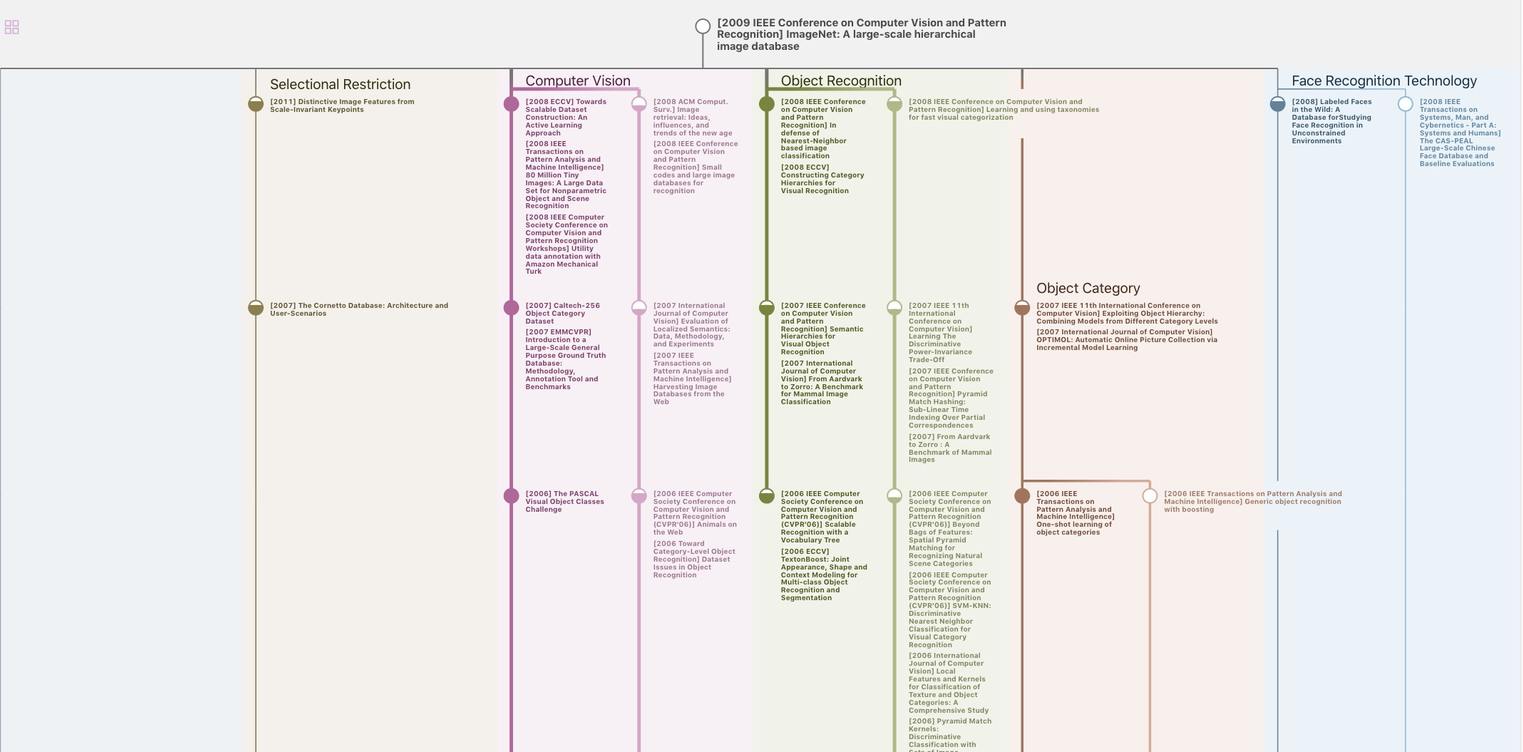
生成溯源树,研究论文发展脉络
Chat Paper
正在生成论文摘要