Stochastic MPC with Situation-Aware Dynamic Risk Assessment for Autonomous Driving.
2023 IEEE 26th International Conference on Intelligent Transportation Systems (ITSC)(2023)
摘要
In this paper, we propose a novel interplay between stochastic model predictive control and situation-aware dynamic risk assessment (SINADRA) for autonomous driving. With the help of SINADRA a Bayesian network is engineered which infers a probabilistic behavior classification for a surrounding actor. This classification is considered in the chance constraints of the model predictive controller (MPC). The MPC can guarantee no constraint violations up to a chosen probability. If a constraint violation occurs, the system is handled by an emergency braking assistant (EBA) ensuring the safety of the overall system. The approach of combining MPC with SINADRA results in a less conservative driving behavior while not introducing additional safety violations. The probability of interference by the EBA can be used as a design parameter, that can be tuned by the manufacturer or possibly by the end-consumer through a safe interface.
更多查看译文
关键词
Autonomous Vehicles,Model Predictive Control,Bayesian Model,Stochastic Model,Constraint Violation,Behavior Classification,Stochastic Control,Emergency Braking,Low Risk,Time Step,Optimization Problem,Monte Carlo Simulation,Cost Function,Predictor Of Behavior,Parametrized,Free Flow,Speed Limit,Hard Constraints,Automated Vehicles,Lane Change,Carbonic Anhydrase II,Conditional Value At Risk,Vehicle Acceleration,Driver Behavior,Advanced Driver Assistance Systems,Risk Scenarios,Reference Velocity,Safety Perspective,Leaf Node,State-space Model
AI 理解论文
溯源树
样例
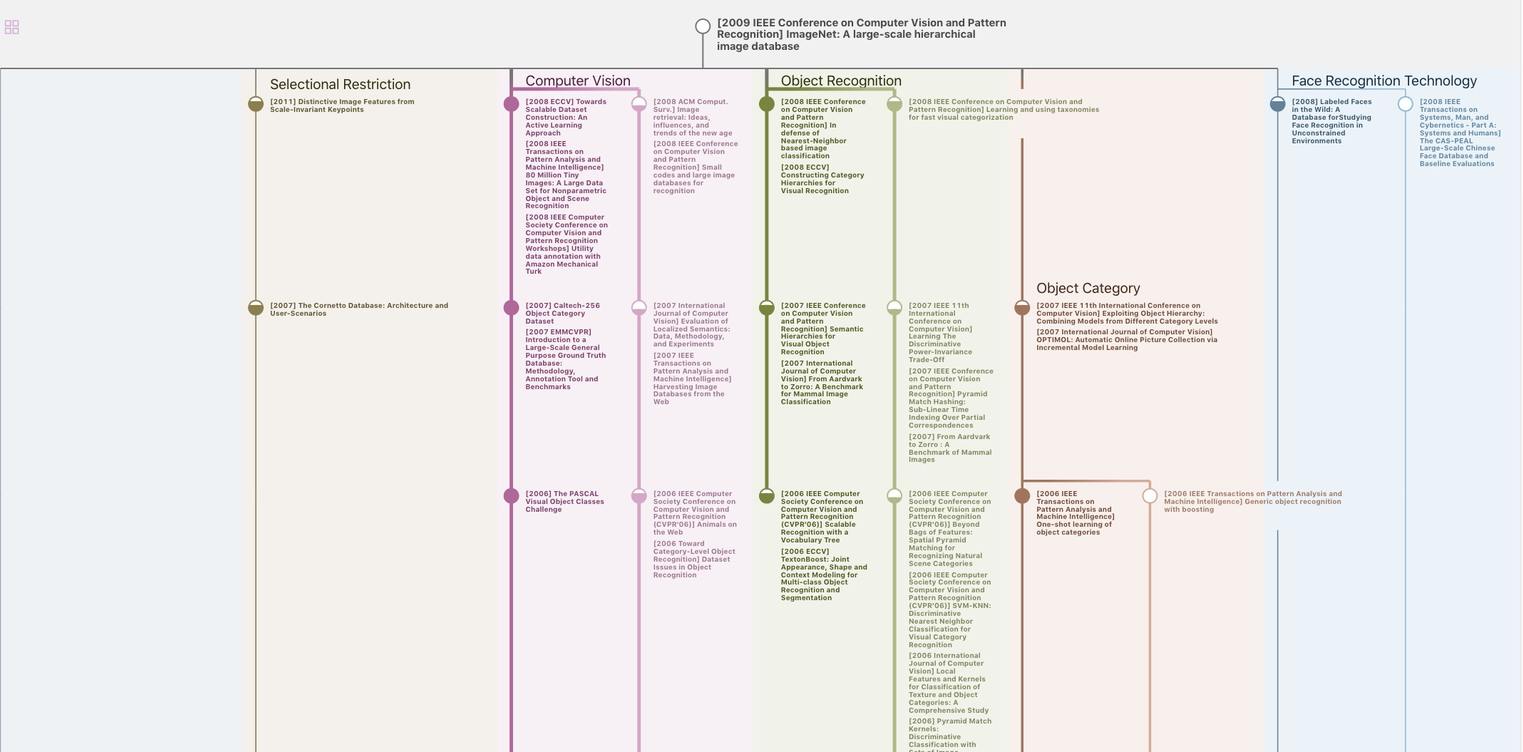
生成溯源树,研究论文发展脉络
Chat Paper
正在生成论文摘要