Adversarial Generation of Safety-Critical Lane-Change Scenarios for Autonomous Vehicles.
2023 IEEE 26th International Conference on Intelligent Transportation Systems (ITSC)(2023)
摘要
Autonomous vehicles must undergo a comprehensive performance evaluation before being deployed in real-world traffic, and testing in safety-critical scenarios is essential for identifying scenarios that autonomous vehicles cannot handle. Given the rarity of safety-critical scenarios, it is necessary to investigate how to systematically generate these scenarios. In this paper, we propose an adversarial method to efficiently generate safety-critical scenarios through deep reinforcement learning. We first formulate the typical lane-change scenarios based on the Markov Decision Process and then train the background vehicles to aggressively interfere with the autonomous vehicle under test and create some risky situations. We also propose a reasonableness reward to avoid the extreme adversarial behavior of the background vehicles, making the scenarios reasonable and informative for the autonomous vehicle testing. Simulation results show that the generated scenarios are more critical in terms of safety than those in the naturalistic environment, significantly degrading the performance of the vehicle under test and providing a basis for improving the model.
更多查看译文
关键词
Autonomous Vehicles,Scenario Generation,Lane Change Scenario,Adversarial Generation,Deep Learning,Typical Scenario,Deep Reinforcement Learning,Markov Decision Process,Learning Process,Transition State,Unreasonable,Term In Eq,Actor Network,Reward Function,Rewarding Effects,Critic Network,Lane Change,Adversary Model,Longitudinal Distance,Reinforcement Learning Problem,Target Vehicle,Velocity Limits,Knowledge-based Methods
AI 理解论文
溯源树
样例
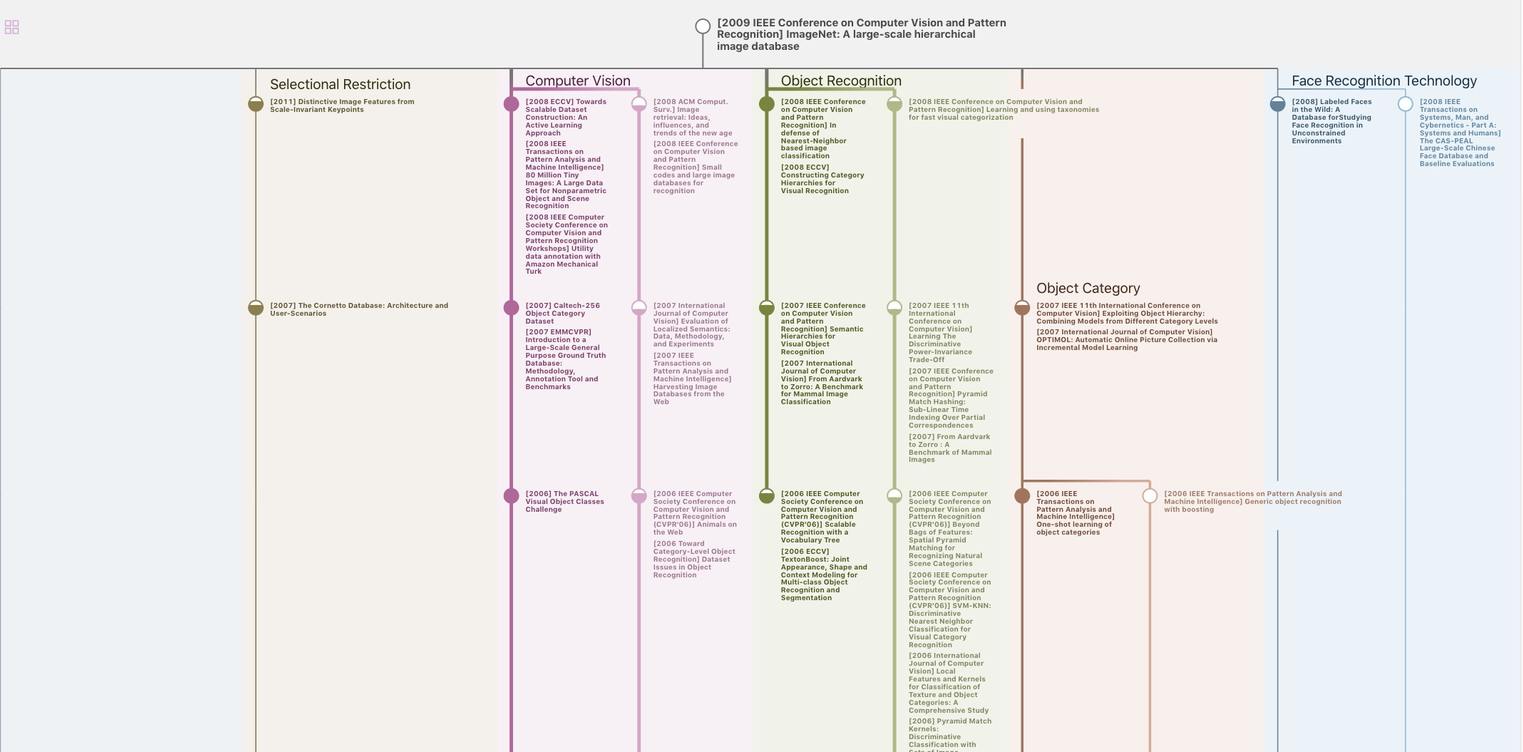
生成溯源树,研究论文发展脉络
Chat Paper
正在生成论文摘要