Communication Optimization for Multi-agent Reinforcement Learning-based Traffic Control System with Explainable Protocol.
2023 IEEE 26th International Conference on Intelligent Transportation Systems (ITSC)(2023)
摘要
This article studies the challenges of multi-agent traffic control systems with a specific focus on the feasibility of communication protocols. We present an innovative approach for optimizing communication in large-scale traffic control systems. In the context of ramp metering coordination, we design and analyze the proposed communication protocols. The first protocol operates without explicit semantic interpretation, providing a baseline for performance. The second builds on the concept of advantageous directions, integrating semantic meaning into communication for enhanced explainability. Comparative results show that our proposed system outperforms the ALINEA algorithm under both protocols. Despite the slightly superior performance of the non-semantic protocol, the advantageous direction protocol yields more interpretable and meaningful results, thereby underscoring the crucial role of explainability in deep learning models. Our approach offers novel insights for the development of interpretable machine learning-based traffic control algorithms. The broader implication of this study emphasizes the importance of addressing communication feasibility in large-scale traffic control systems, illuminating the path toward more efficient, scalable traffic control solutions.
更多查看译文
关键词
Multi-agent Systems,Traffic Control,Traffic System,Traffic Control System,Semantic,Deep Learning,Meaningful Results,Large-scale Systems,Communication Protocol,Communication Network,Global Status,Autonomous Vehicles,Optimal Policy,Position Vector,Shock Wave,Transition Model,Half Hour,Reward Function,Markov Decision Process,Local Observations,Saliency Map,Multi-agent Reinforcement Learning,Agent System,Perception Module,Central Server,Roadside Units,Function Of Agent
AI 理解论文
溯源树
样例
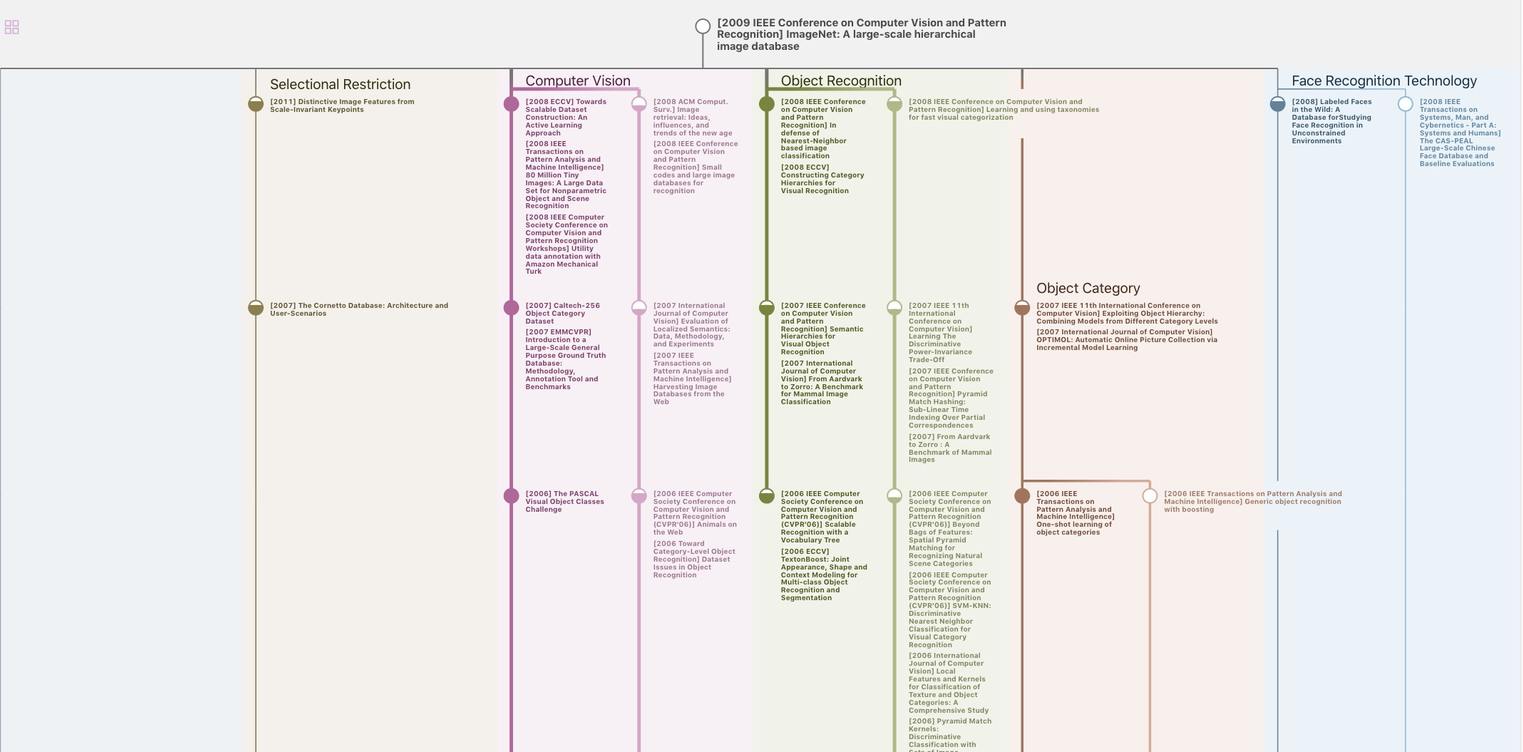
生成溯源树,研究论文发展脉络
Chat Paper
正在生成论文摘要