Machine-learning-aided prediction and optimization of struvite recovery from synthetic wastewater
JOURNAL OF WATER PROCESS ENGINEERING(2024)
摘要
Struvite recovery from wastewater is a promising direction for recovering phosphorus and nitrogen nutrients. However, traditional experiment-basis optimization of struvite crystallization conditions is time-consuming, labor-intensive, and limited to the number of variables. Machine learning (ML) was conducted here to help achieve favorable experimental struvite recovery from synthetic wastewater. Single-target and multi-target prediction of P_recovery and N_recovery using seven process parameters as inputs were performed by gradient boosting regression and random forest (RF) models. The RF models, with test R2 of 0.86-0.94 and RMSE of 5.48-10.17, outperformed the GBR ones for both single- and multi-target predictions. The effects of various process conditions on struvite crystallization were clarified by ML model interpretation. To obtain high phosphorus and nitrogen recoveries, the RF prediction model was used to optimize the crystallization conditions of struvite, which were then experimentally validated. The preferable experimental verification results, with relative errors for the ten optimum solutions' P_recovery and N_recovery being 0.18-4.67% and 0.12-7.32%, respectively, indicate the great potential of using ML to promote struvite formation for recovering P and N.
更多查看译文
关键词
Magnesium ammonium phosphate,Artificial intelligence,Nitrogen recovery,Phosphorus recovery,Struvite crystallization,Machine learning
AI 理解论文
溯源树
样例
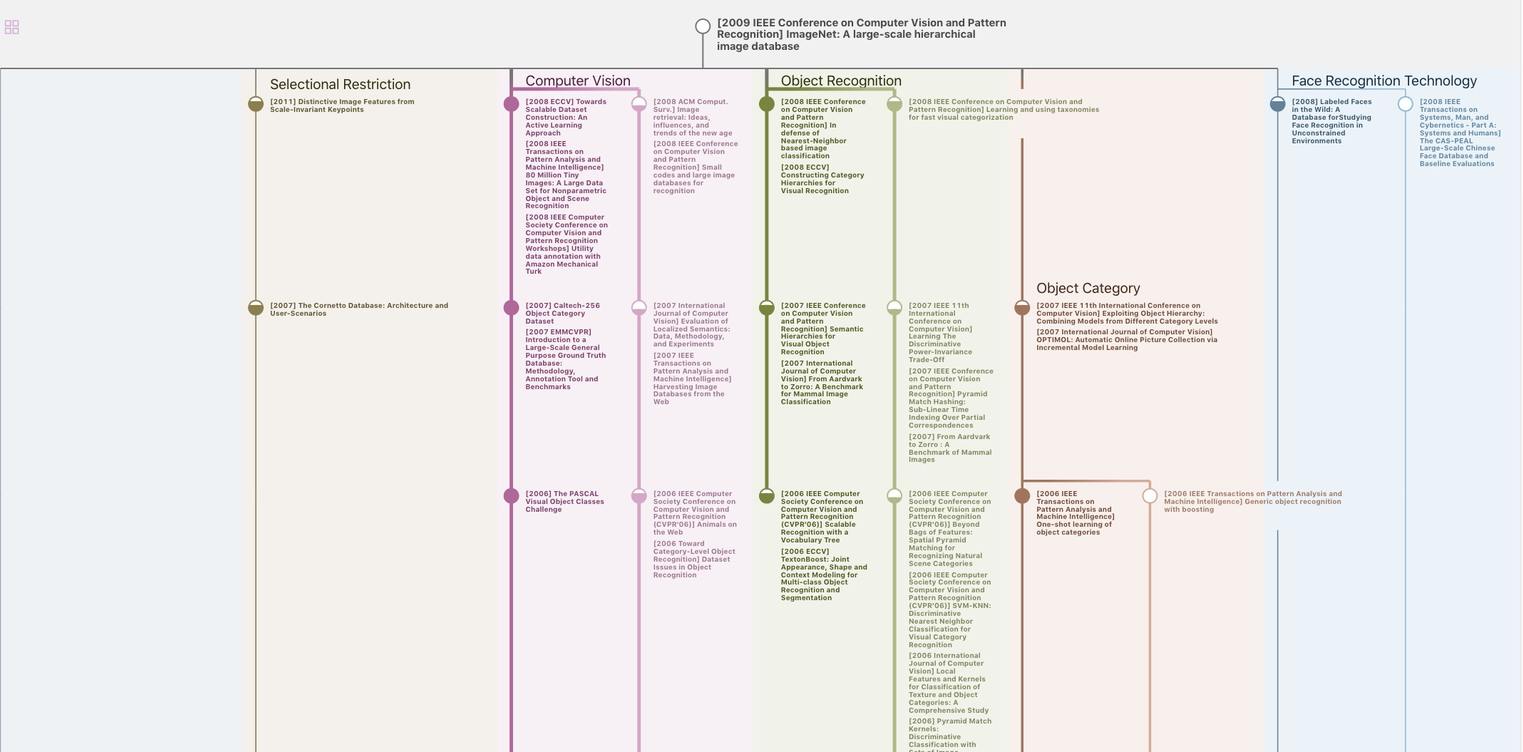
生成溯源树,研究论文发展脉络
Chat Paper
正在生成论文摘要