An Intention-Based Multi-Modal Trajectory Prediction Framework for Overtaking Maneuver.
2023 IEEE 26th International Conference on Intelligent Transportation Systems (ITSC)(2023)
摘要
Understanding the intention of other traffic participants and anticipating their future trajectories can help autonomous vehicle make decisions in advance. The proposed intention-based multi-modal trajectory prediction framework (IMTPF) aims to predict the complex overtaking trajectories of human-driven rear vehicle on highways to assist autonomous vehicles in decision-making. The framework uses a modified LSTM encoder-decoder and a novel social pooling operation to model spatial interactions. Nine motions are defined to show the multi-modal nature of rear vehicle during overtaking, and Time-to-Collision (TTC) is used to measure the risk. The trajectory prediction performance is analyzed based on capturing the rear vehicle's overtaking intention. The experimental results demonstrate that the proposed method outperforms other trajectory prediction methods.
更多查看译文
关键词
Trajectory Prediction,Multimodal Trajectory,Autonomous Vehicles,Future Trajectories,Root Mean Square Error,Bimodal,Convolutional Layers,Constant Velocity,Lateral Position,ReLU Activation Function,Prediction Horizon,Spatial Grid,Future Position,Vehicle Motion,Lane Change,Motion Prediction,Collision Risk,Geometric Size,Longitudinal Position,Convolutional Long Short-term Memory,Context Vector,Physics-based Approach,Longitudinal Prediction,Constant Velocity Model,Hidden State,Impact Velocity,Time Step
AI 理解论文
溯源树
样例
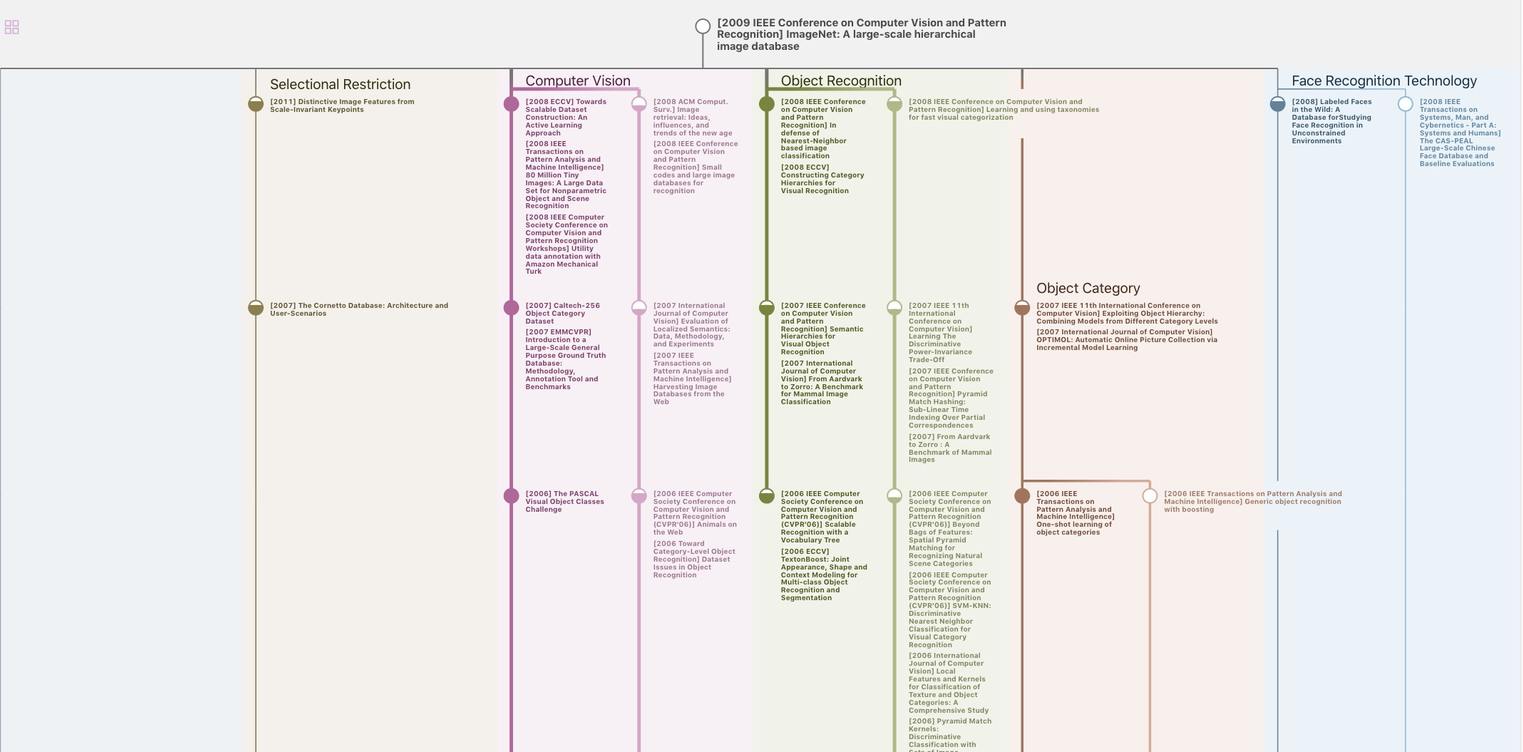
生成溯源树,研究论文发展脉络
Chat Paper
正在生成论文摘要