Generating Diverse Translation with Perturbed kNN-MT
CoRR(2024)
摘要
Generating multiple translation candidates would enable users to choose the
one that satisfies their needs. Although there has been work on diversified
generation, there exists room for improving the diversity mainly because the
previous methods do not address the overcorrection problem – the model
underestimates a prediction that is largely different from the training data,
even if that prediction is likely. This paper proposes methods that generate
more diverse translations by introducing perturbed k-nearest neighbor machine
translation (kNN-MT). Our methods expand the search space of kNN-MT and help
incorporate diverse words into candidates by addressing the overcorrection
problem. Our experiments show that the proposed methods drastically improve
candidate diversity and control the degree of diversity by tuning the
perturbation's magnitude.
更多查看译文
AI 理解论文
溯源树
样例
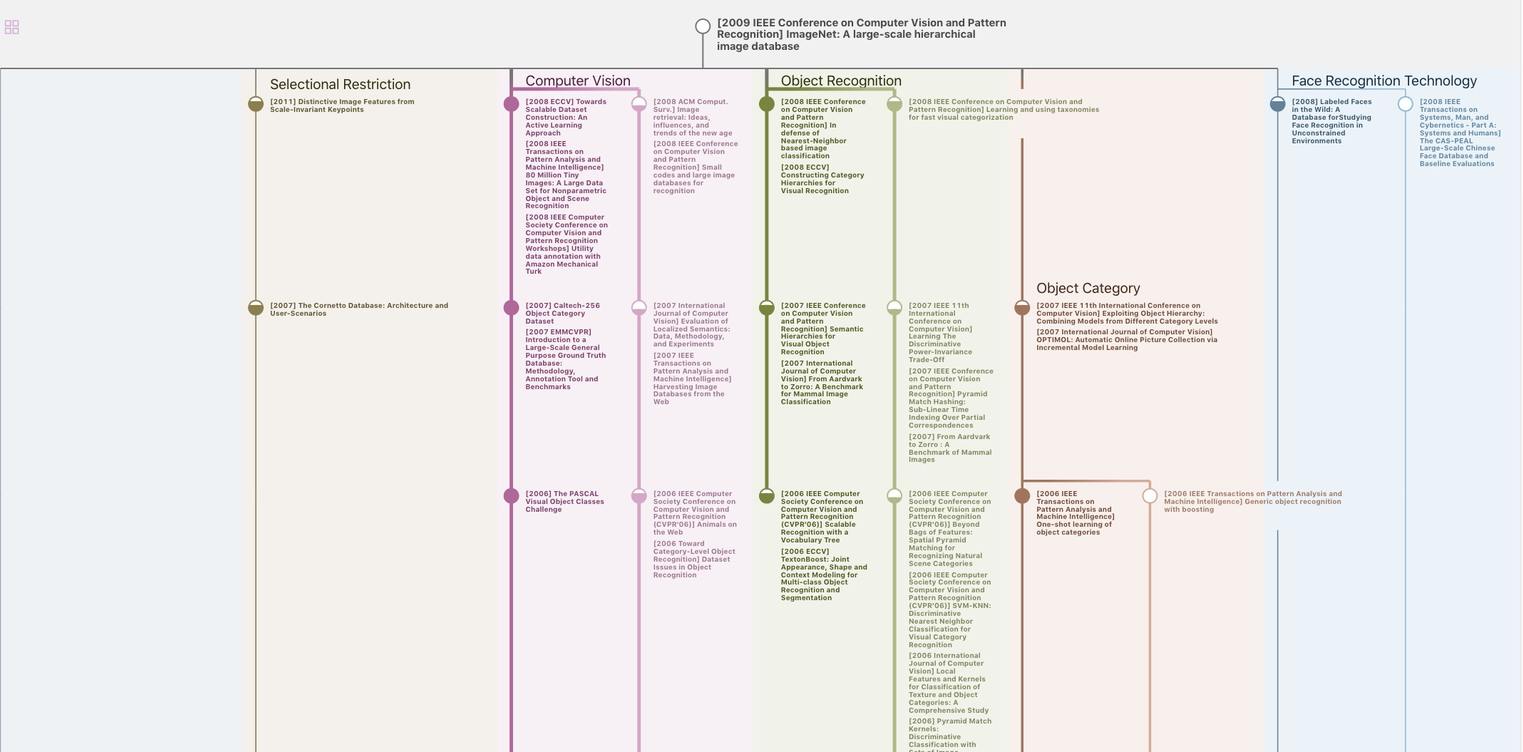
生成溯源树,研究论文发展脉络
Chat Paper
正在生成论文摘要