Pedestrian Tracking Using 3D LiDARs - Case for Proximity Scenario.
2023 IEEE 26th International Conference on Intelligent Transportation Systems (ITSC)(2023)
摘要
In this paper, we address a critical issue of pedestrian tracking using 3D LiDARs. In particular, the segmentation of 3D point clouds to identify individual pedestrians is not stable when multiple pedestrians walk in close proximity (e.g., walking in a group), which significantly degrades tracking accuracy. To cope with this issue, we introduce a CNN-based method that predicts the population in each 3D point cloud segment. The Kalman-filter-based tracking process is fully tailored to include this prediction. We evaluated the proposed method in both a crowded environment and a real-world scenario using 3D point clouds obtained from multiple LiDAR units installed in our university campus building. The evaluation resulted in a MOTA (Multiple Object Tracking Accuracy) score of 0.977, representing the accuracy of person detection and ID assignment in tracking multiple individuals.
更多查看译文
关键词
3D LiDAR,Pedestrian Tracking,Close Proximity,Point Cloud,Multiple Individuals,Tracking Accuracy,Accurate Assignment,3D Point Cloud,Tracking Process,Walking Group,Point Cloud Segmentation,Identity Assignment,Multiple Object Tracking,Crowding,Convolutional Neural Network,Convolutional Layers,K-means,Background Subtraction,Filtering Step,Bounding Box,Kalman Filter,Point Cloud Data,Pair Of Elements,Object In Frame,LiDAR Sensor,KITTI Dataset,Tracking Performance,Irregular Movements,Point Cloud Representation,3D Point Cloud Data
AI 理解论文
溯源树
样例
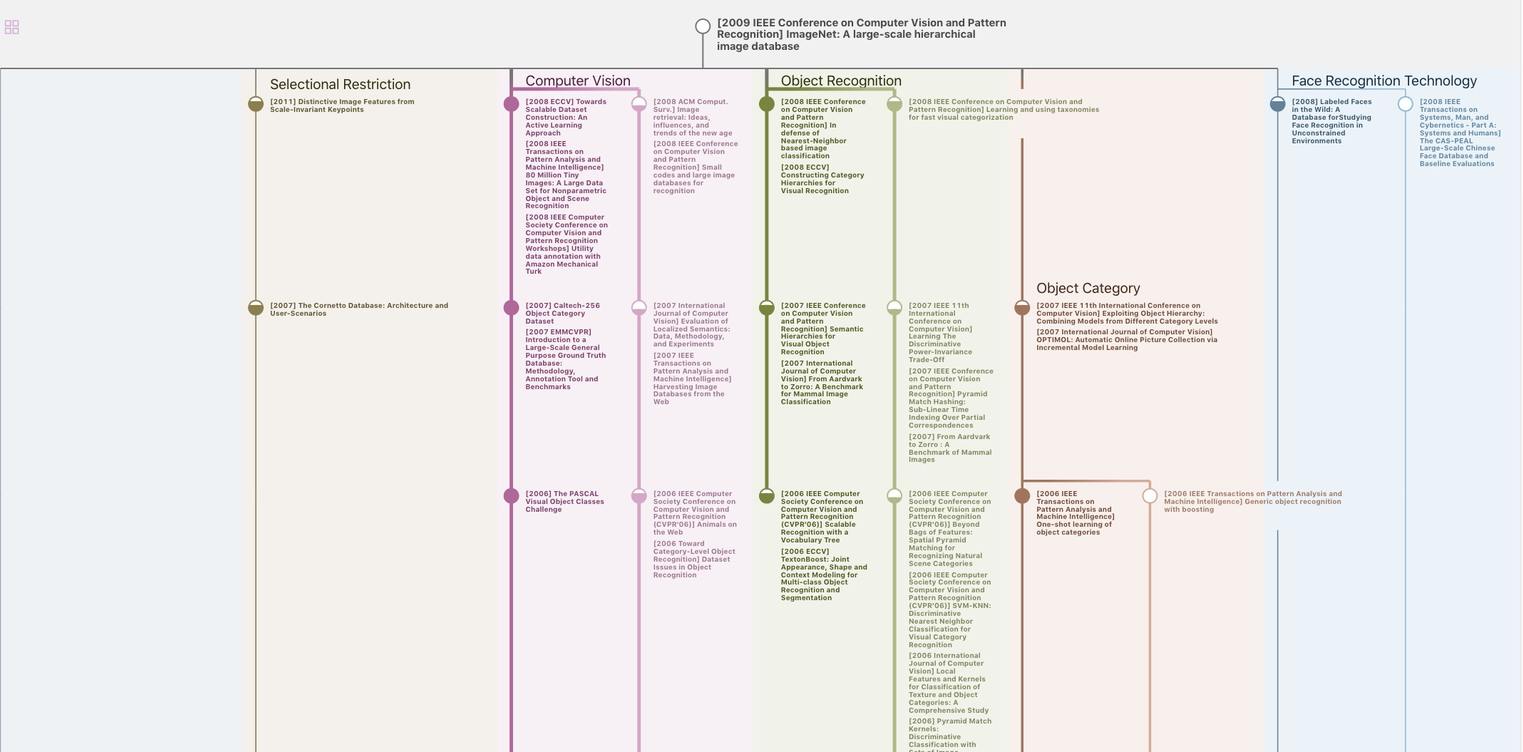
生成溯源树,研究论文发展脉络
Chat Paper
正在生成论文摘要