Electric Vehicle Charging Load Prediction Method Based on Nonlinear Auto-Regressive Neural Networks.
ICCEIC(2023)
摘要
Accurately predicting the charging load of electric vehicles is fundamental for studying the impact of large-scale electric vehicle integration on the power grid and is an important measure for ensuring the safe and economical operation of charging stations. To improve the accuracy of ultra-short-term charging load forecasting at electric vehicle charging stations, a prediction method based on the NAR (Nonlinear Autoregressive) neural network is proposed. The model selects Levenberg-Marquardt (LM) as the network training method and employs a recursive prediction approach to forecast medium- to long-term clock deviation, along with a designed data preprocessing scheme based on clock deviation variation. The NAR model is utilized to conduct short-term forecasting of the charging load of electric vehicles and is experimentally compared with the Backpropagation (BP) neural network method. The results demonstrate that the NAR network model exhibits good predictive performance for charging load forecasting, outperforming the BP neural network model, with prediction error values lower than those of the BP neural network. Therefore, the NAR neural network proves effective in addressing nonlinear problems with dynamic characteristics and temporal relationships, presenting advantages of high accuracy and low error in resolving electric vehicle charging station load prediction issues.
更多查看译文
关键词
Electric vehicles,load modeling,charging load forecasting,NAR neural network
AI 理解论文
溯源树
样例
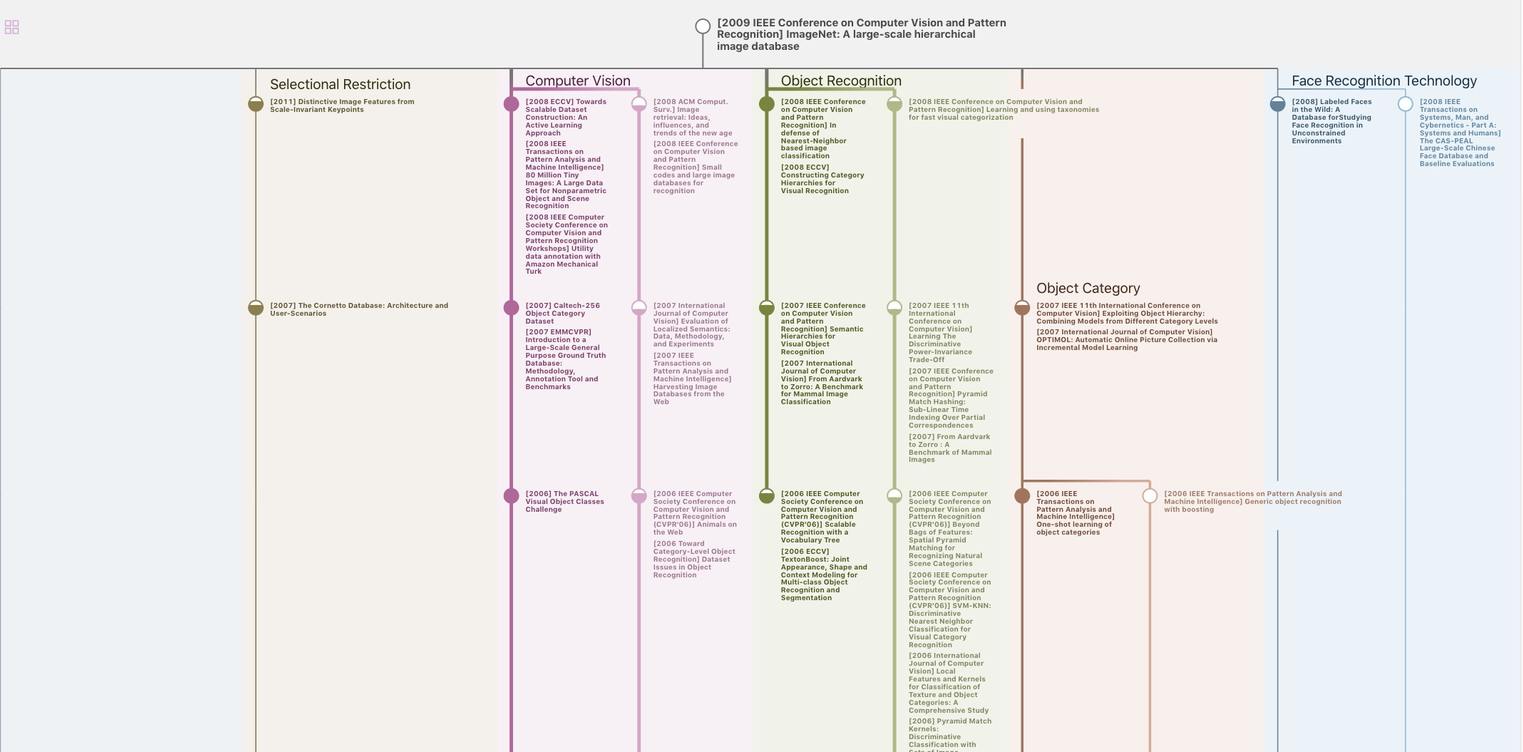
生成溯源树,研究论文发展脉络
Chat Paper
正在生成论文摘要