A Hybrid Model for Real-Time Traffic Prediction with Augmented Historical Data Using Traffic Simulation.
2023 IEEE 26th International Conference on Intelligent Transportation Systems (ITSC)(2023)
摘要
Real-time traffic prediction offers valuable tools for efficient traffic operation through timely and accurate information to road users and agencies. Despite the prevalence of data-driven approaches in traffic prediction with the advent of big data, these methods struggle to respond to unforeseen traffic situations during real-time prediction. To address this issue, we propose a hybrid model that integrates both data-driven and model-based approaches. Our model augments historical data using traffic simulation for unexpected traffic situations such as sudden speed drops on a road section, which can cause significant prediction errors. An artificial neural network is used to balance between the collected historical data and the augmented data. The proposed model was evaluated by predicting traffic conditions of an actual road section and significantly improved prediction accuracy. Our proposed method represents a state-of-the-art solution for enhancing real-time traffic prediction under the hybrid framework.
更多查看译文
关键词
real-time traffic prediction,hybrid model,machine learning,traffic simulation,deep neural networks
AI 理解论文
溯源树
样例
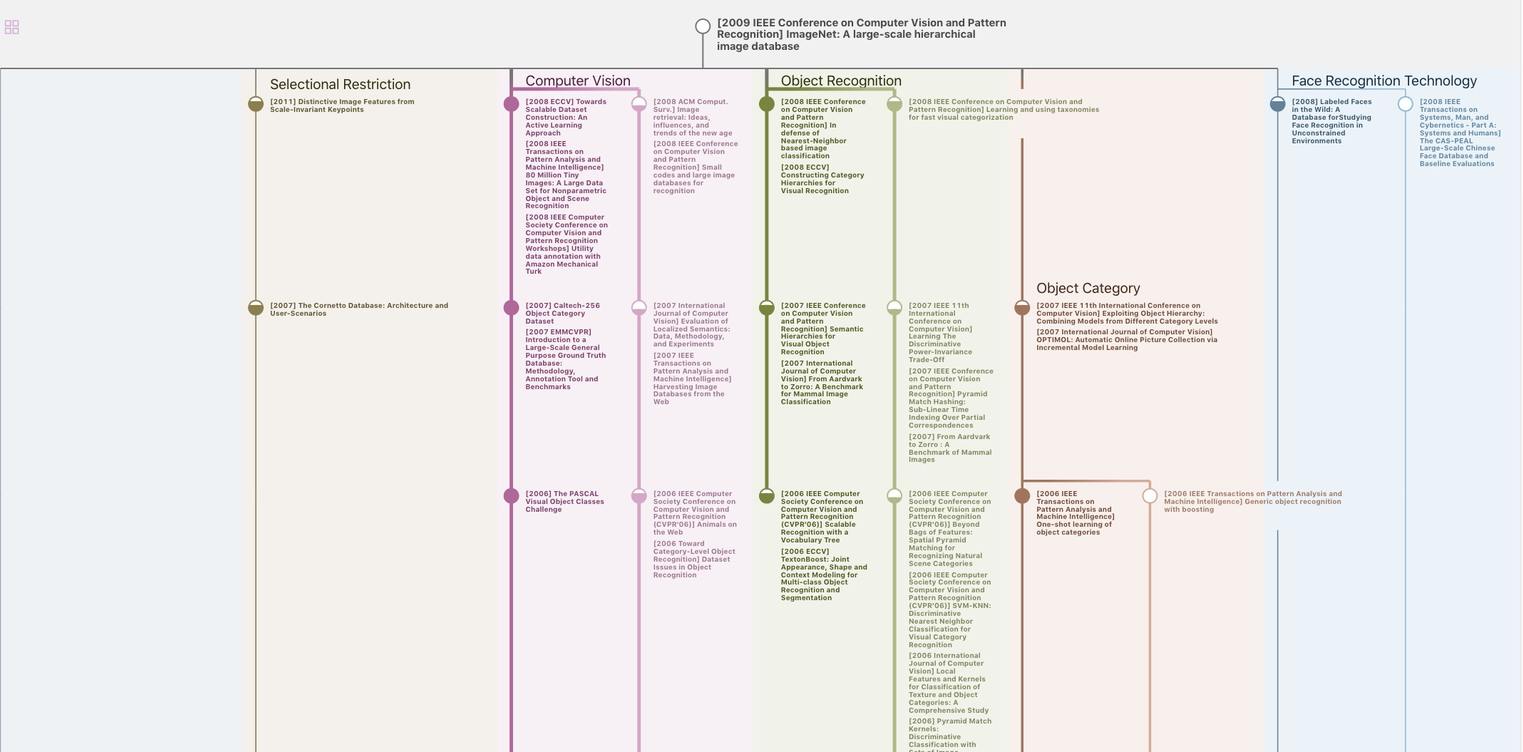
生成溯源树,研究论文发展脉络
Chat Paper
正在生成论文摘要