Semantic Segmentation for Tooth Cracks Using Improved DeepLabv3+ Model
Heliyon(2024)
摘要
Objective: Accurate and prompt detection of cracked teeth plays a critical role for human oral health. The aim of this paper is to evaluate the performance of a tooth crack segmentation model (namely, FDB-DeepLabv3+) on optical microscopic images. Method: The FDB-DeepLabv3+ model proposed here improves feature learning by replacing the backbone with ResNet50. Feature pyramid network (FPN) is introduced to fuse muti-level features. Densely linked atrous spatial pyramid pooling (Dense ASPP) is applied to achieve denser pixel sampling and wider receptive field. Bottleneck attention module (BAM) is embedded to enhance local feature extraction. Results: Through testing on a self-made hidden cracked tooth dataset, the proposed method outperforms four classical networks (FCN, U-Net, SegNet, DeepLabv3+) on segmentation results in terms of mean pixel accuracy (MPA) and mean intersection over union (MIoU). The network achieves an increase of 11.41% in MPA and 12.14% in MIoU compared to DeepLabv3+. Ablation experiments shows that all the modifications are beneficial. Conclusion: An improved network is designed for segmenting tooth surface cracks with good overall performance and robustness, which may hold significant potential in computer-aided diagnosis of cracked teeth.
更多查看译文
关键词
Cracked teeth,Oral health,Semantic segmentation,DeepLabv3+
AI 理解论文
溯源树
样例
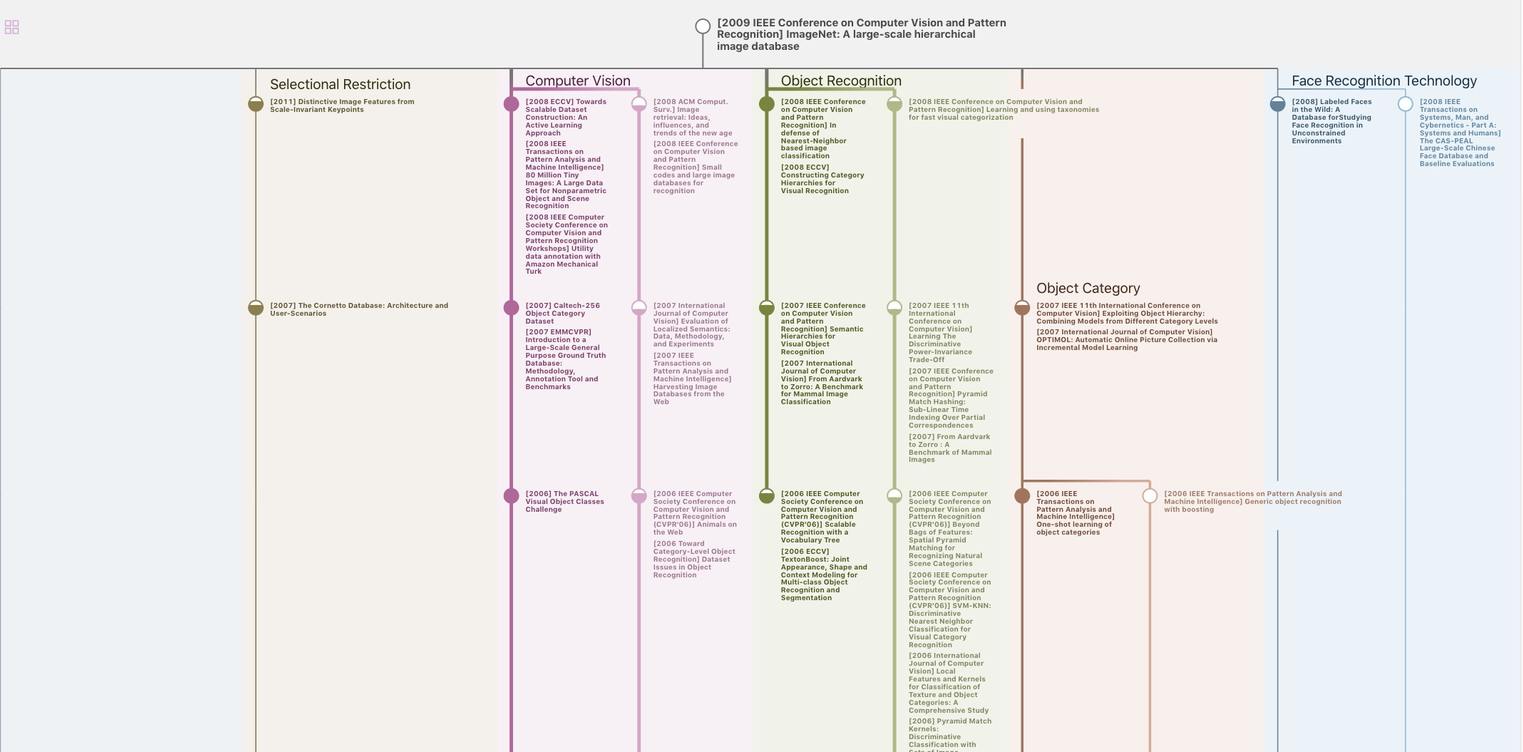
生成溯源树,研究论文发展脉络
Chat Paper
正在生成论文摘要