Diffusion-UDA: Diffusion-based unsupervised domain adaptation for submersible fault diagnosis
ELECTRONICS LETTERS(2024)
摘要
Deep learning has demonstrated notable success in mechanical signal processing with a large amount labelled data. However, the systems of the Jiaolong deep-sea submersible prone to malfunction are typically diverse, due to the high complexity of its structure and operational environment. Consequently, this diversity gives rise to variations in the types of sensor signals and their associated data distributions that require analysis. Unsupervised domain adaptation (UDA) uses transferable knowledge derived from the source domain and applies it to an unlabelled target domain in order to improve the reusability of pre-existing models and data. Inspired by the diffusion models that have the robust capabilities to transform data distributions across a large gap, we propose a novel diffusion-based unsupervised domain adaptation (diffusion-UDA) model, which leverages contrastive learning to alleviate the challenges of cross-domain analysis for fault diagnosis within different systems of the deep-sea submersible. Experimental results show the proposed method achieves state-of-the-art performance on various benchmarks. This letter proposes a novel domain adaptation approach diffusion-UDA for tackling the problem of labour-intensive and time-consuming labelling for the Jiaolong submersible sensor data. In this work, the diffusion model is applied in the transfer between different domains to obtain intermediate domains, and we use contrastive learning as the basic framework for inter-domain feature learning to improve the detection performance for the target domain. image
更多查看译文
关键词
artificial intelligence,fault diagnosis,signal processing
AI 理解论文
溯源树
样例
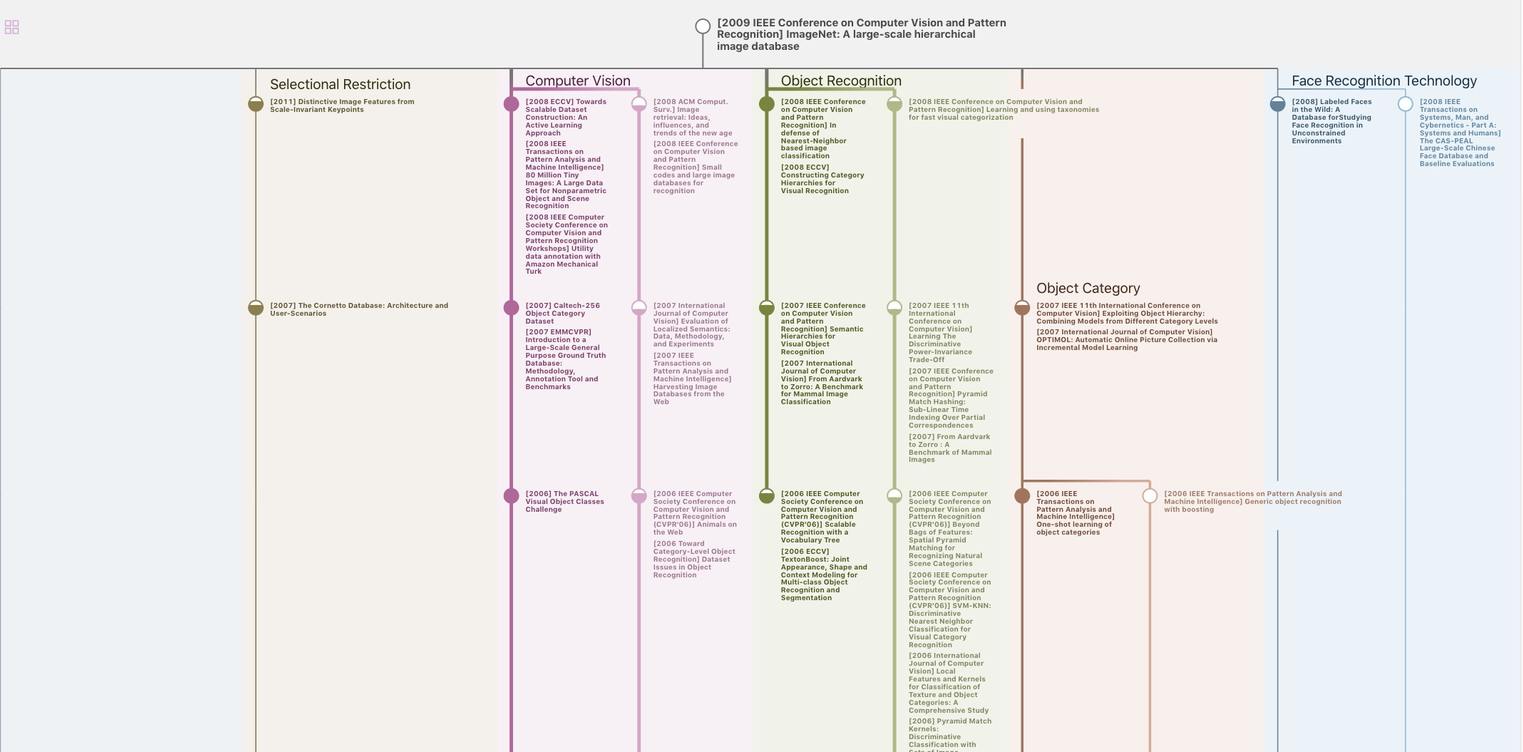
生成溯源树,研究论文发展脉络
Chat Paper
正在生成论文摘要