EM-Trans: Edge-Aware Multimodal Transformer for RGB-D Salient Object Detection
IEEE TRANSACTIONS ON NEURAL NETWORKS AND LEARNING SYSTEMS(2024)
摘要
RGB-D salient object detection (SOD) has gained tremendous attention in recent years. In particular, transformer has been employed and shown great potential. However, existing transformer models usually overlook the vital edge information, which is a major issue restricting the further improvement of SOD accuracy. To this end, we propose a novel edge-aware RGB-D SOD transformer, called, which explicitly models the edge information in a dual-band decomposition framework. Specifically, we employ two parallel decoder networks to learn the high-frequency edge and low-frequency body features from the low-and high-level features extracted from a two-steam multimodal backbone network, respectively. Next, we propose a cross-attention complementarity exploration module to enrich the edge/body features by exploiting the multimodal complementarity information. The refined features are then fed into our proposed color-hint guided fusion module for enhancing the depth feature and fusing the multimodal features. Finally, the resulting features are fused using our deeply supervised progressive fusion module, which progressively integrates edge and body features for predicting saliency maps. Our model explicitly considers the edge information for accurate RGB-D SOD, overcoming the limitations of existing methods and effectively improving the performance. Extensive experiments on benchmark datasets demonstrate that is an effective RGB-D SOD framework that outperforms the current state-of-the-art models, both quantitatively and qualitatively. A further extension to RGB-T SOD demonstrates the promising potential of our model in various kinds of multimodal SOD tasks.
更多查看译文
关键词
Edge-aware model,multimodal learning,salient object detection (SOD),transformer
AI 理解论文
溯源树
样例
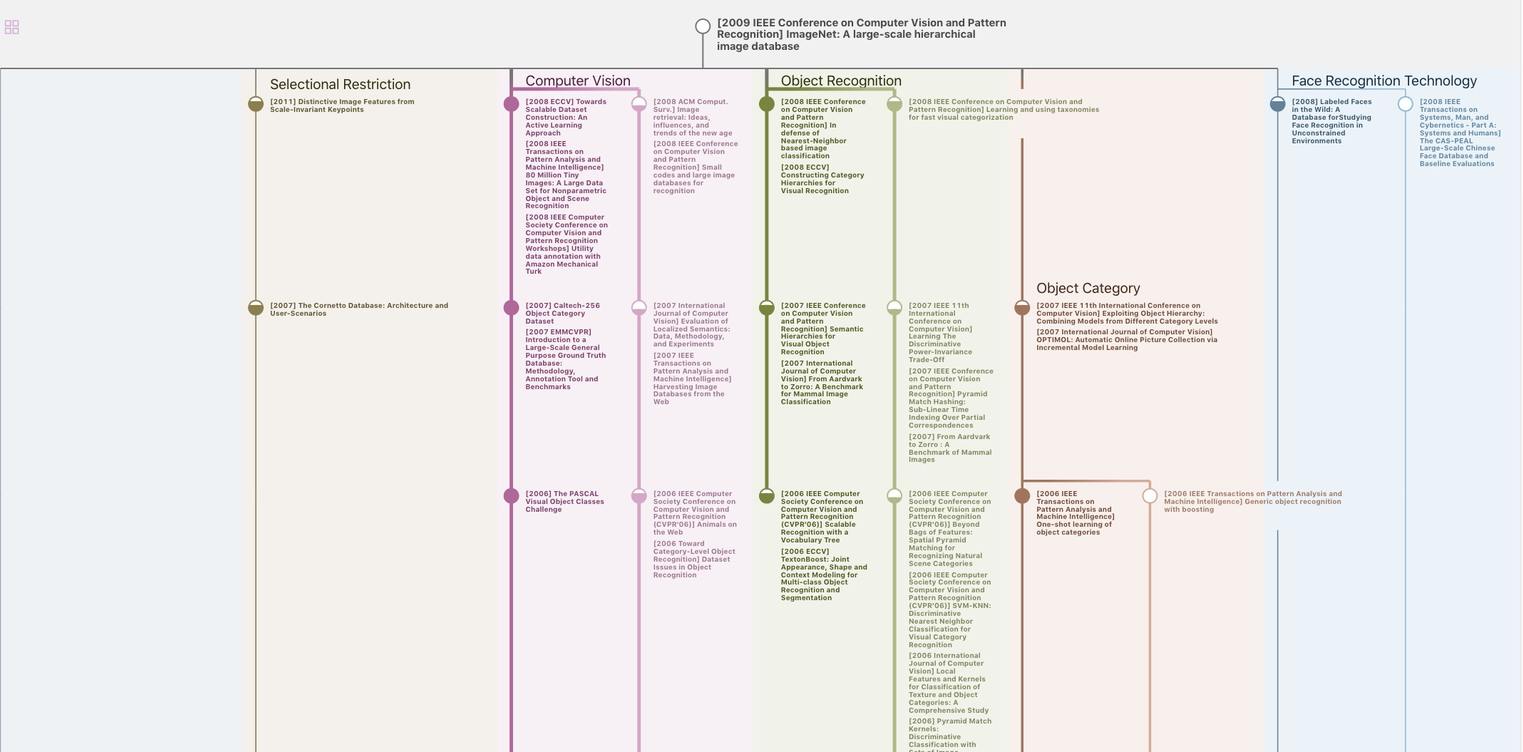
生成溯源树,研究论文发展脉络
Chat Paper
正在生成论文摘要