A Gated Recurrent Generative Transfer Learning Network for Fault Diagnostics Considering Imbalanced Data and Variable Working Conditions
IEEE TRANSACTIONS ON NEURAL NETWORKS AND LEARNING SYSTEMS(2024)
摘要
Transfer learning (TL) and generative adversarial networks (GANs) have been widely applied to intelligent fault diagnosis under imbalanced data and different working conditions. However, the existing data synthesis methods focus on the overall distribution alignment between the generated data and real data, and ignore the fault-sensitive features in the time domain, which results in losing convincing temporal information for the generated signal. For this reason, a novel gated recurrent generative TL network (GRGTLN) is proposed. First, a smooth conditional matrix-based gated recurrent generator is proposed to extend the imbalanced dataset. It can adaptively increase the attention of fault-sensitive features in the generated sequence. Wasserstein distance (WD) is introduced to enhance the construction of mapping relationships to promote data generation ability and transfer performance of the fault diagnosis model. Then, an iterative "generation-transfer" co-training strategy is developed for continuous parallel training of the model and the parameter optimization. Finally, comprehensive case studies demonstrate that GRGTLN can generate high-quality data and achieve satisfactory cross-domain diagnosis accuracy.
更多查看译文
关键词
Fault diagnosis,gated recurrent generative transfer learning network (GRGTLN),"generation-transfer" co-training training strategy,imbalances data,smooth conditional matrix
AI 理解论文
溯源树
样例
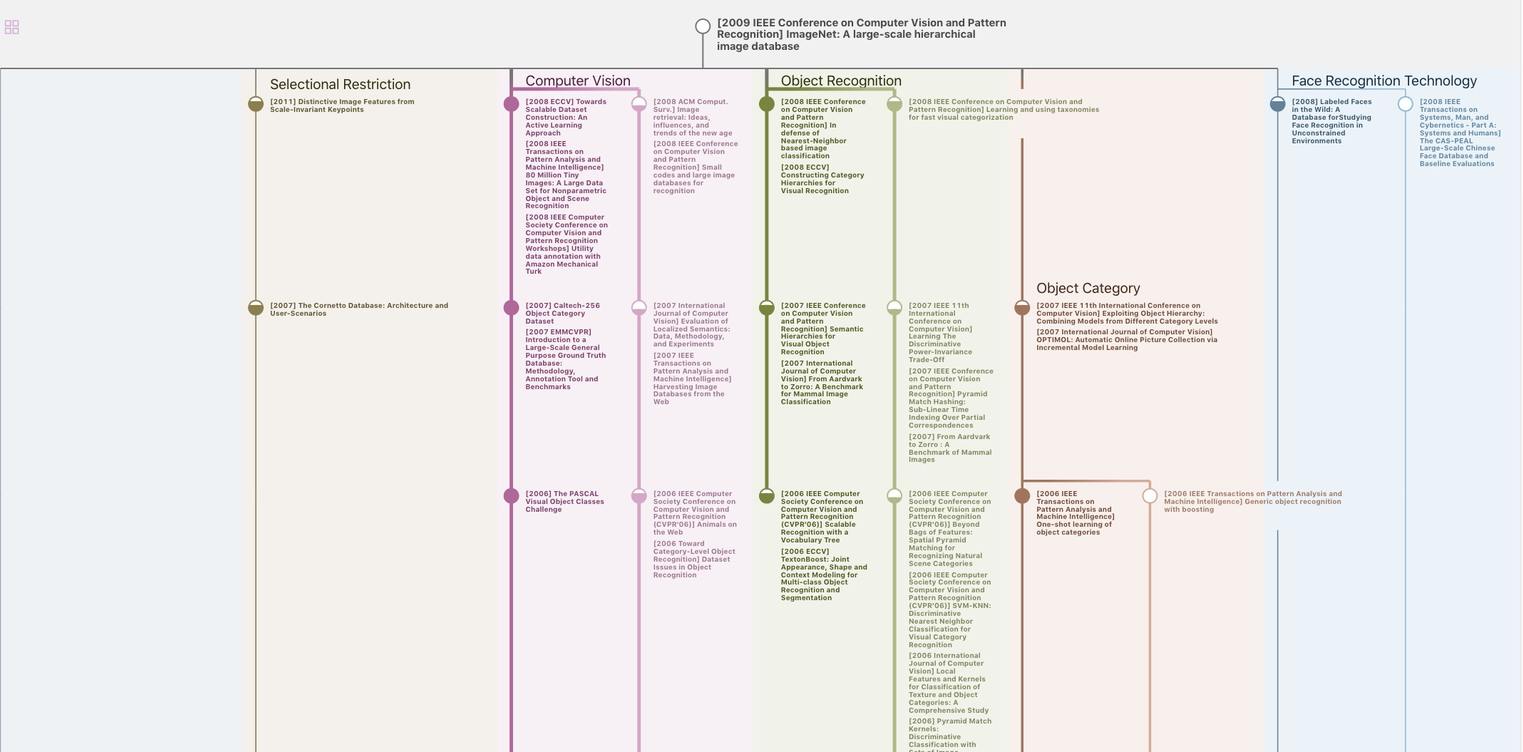
生成溯源树,研究论文发展脉络
Chat Paper
正在生成论文摘要