Predicting Fitness Related Traits Using Gene Expression and Machine Learning
bioRxiv (Cold Spring Harbor Laboratory)(2024)
摘要
Evolution by natural selection occurs at its most basic through the change in frequencies of alleles; connecting those genomic targets to phenotypic selection is an important goal for evolutionary biology in the genomics era. The relative abundance of gene products expressed in a tissue can be considered a phenotype intermediate to the genes and genomic regulatory elements themselves, and more traditionally measured macroscopic phenotypic traits such as flowering time, size, or growth. The high-dimensionality, low sample size nature of transcriptomic sequence data is a double-edged sword, however, as it provides abundant information but makes traditional statistics difficult. Machine learning has many features which handle high-dimensional data well and is thus useful in genetic sequence applications. Here we examined the association of fitness-components with gene expression data in Ipomoea hederacea (Ivyleaf Morning Glory) grown under field conditions. We combine the results of three different machine learning approaches and find evidence that expression of photosynthesis-related genes is likely under selection. We also find that genes related to stress and light response were overall important in predicting fitness. With this study we demonstrate the utility of machine learning models for smaller samples, and their potential application for understanding natural selection.### Competing Interest StatementThe authors have declared no competing interest.
更多查看译文
关键词
Feature Selection
AI 理解论文
溯源树
样例
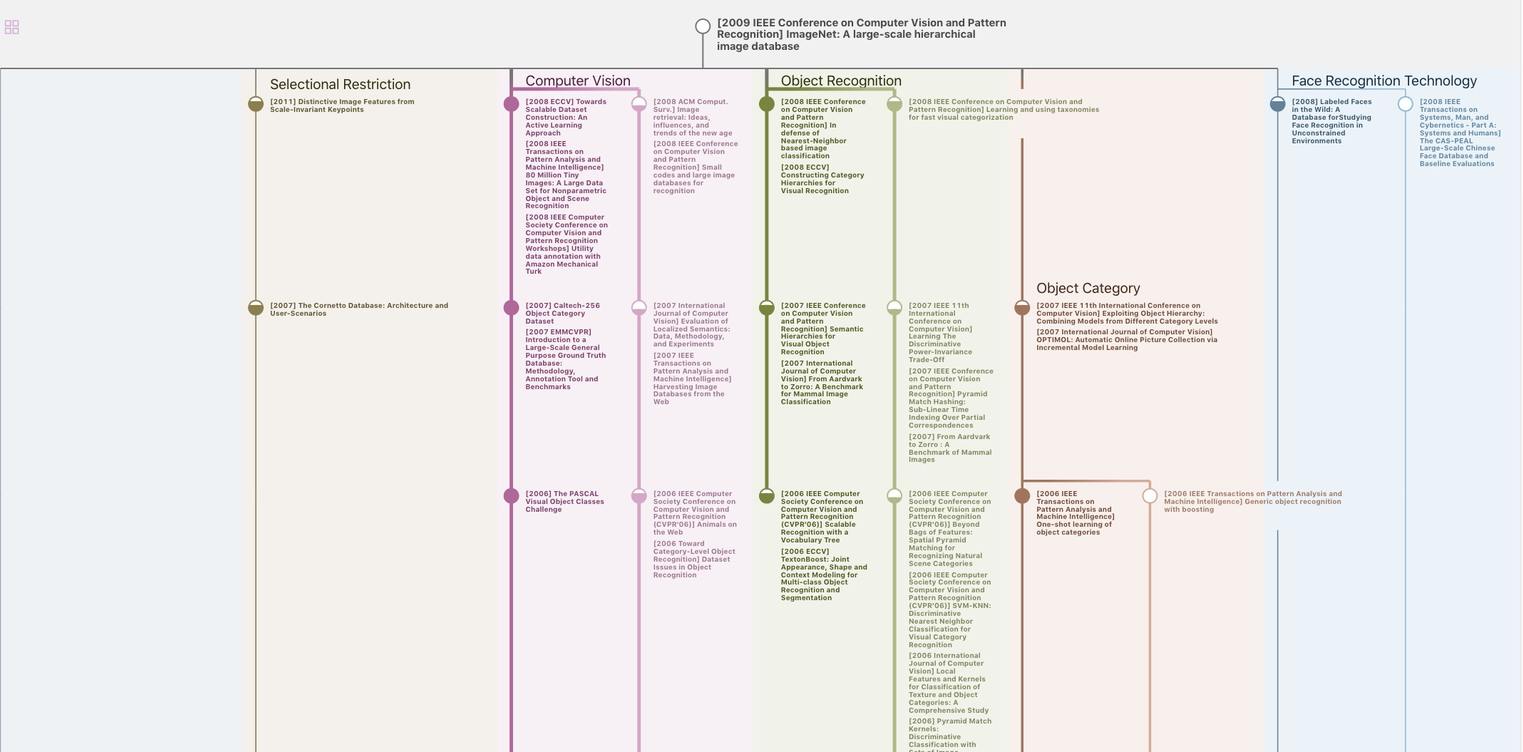
生成溯源树,研究论文发展脉络
Chat Paper
正在生成论文摘要