Self-Supervised Learning of Visual Robot Localization Using LED State Prediction as a Pretext Task
IEEE ROBOTICS AND AUTOMATION LETTERS(2024)
摘要
We propose a novel self-supervised approach for learning to visually localize robots equipped with controllable LEDs. We rely on a few training samples labeled with position ground truth and many training samples in which only the LED state is known, whose collection is cheap. We show that using LED state prediction as a pretext task significantly helps to learn the visual localization end task. The resulting model does not require knowledge of LED states during inference. We instantiate the approach to visual relative localization of nano-quadrotors: experimental results show that using our pretext task significantly improves localization accuracy (from 68.3% to 76.2%) and outperforms alternative strategies, such as a supervised baseline, model pre-training, and an autoencoding pretext task. We deploy our model aboard a 27-g Crazyflie nano-drone, running at 21 fps, in a position-tracking task of a peer nano-drone. Our approach, relying on position labels for only 300 images, yields a mean tracking error of 4.2 cm versus 11.9 cm of a supervised baseline model trained without our pretext task.
更多查看译文
关键词
Deep Learning for visual perception,deep learning methods,micro/nano robots
AI 理解论文
溯源树
样例
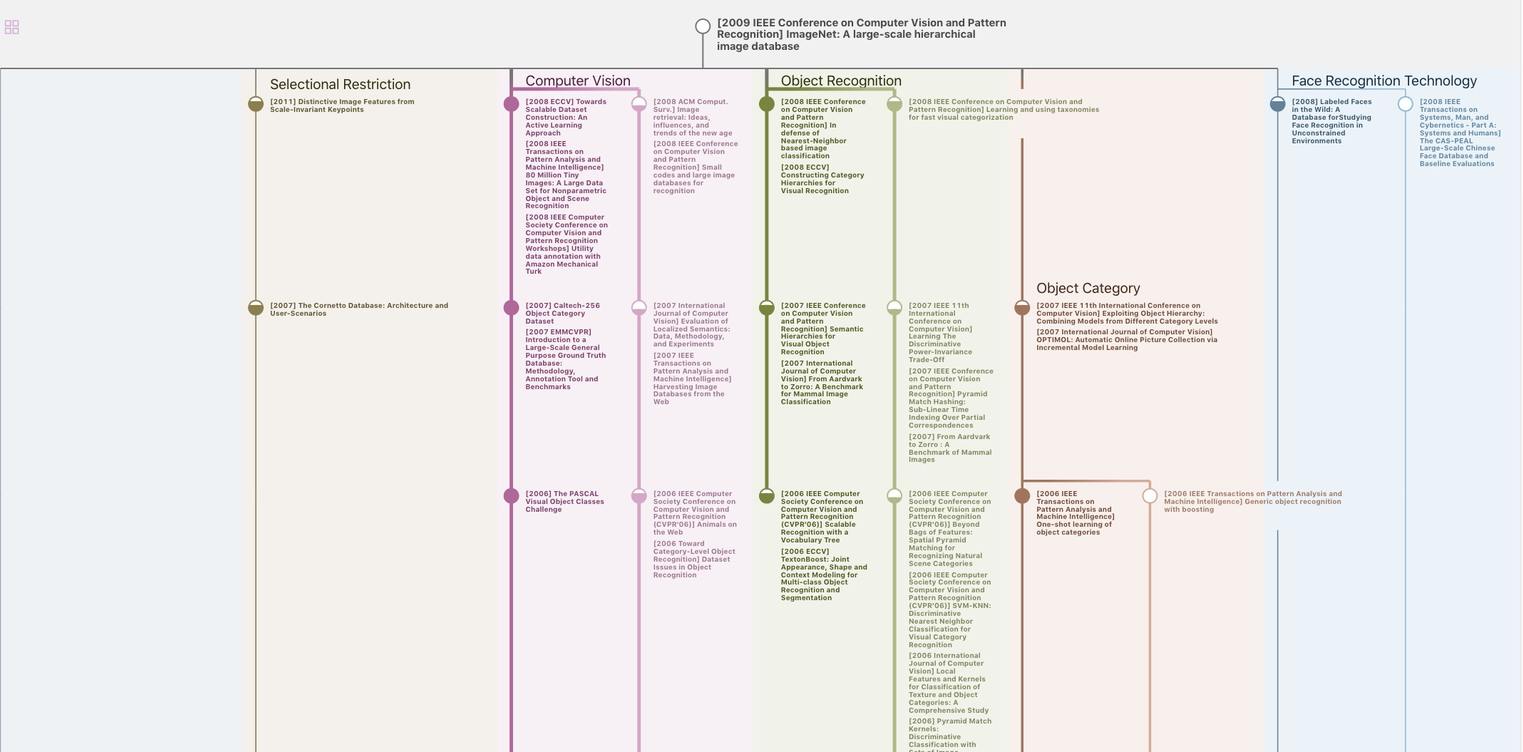
生成溯源树,研究论文发展脉络
Chat Paper
正在生成论文摘要