An Accelerated Distributed Stochastic Gradient Method with Momentum
CoRR(2024)
摘要
In this paper, we introduce an accelerated distributed stochastic gradient
method with momentum for solving the distributed optimization problem, where a
group of n agents collaboratively minimize the average of the local objective
functions over a connected network. The method, termed “Distributed Stochastic
Momentum Tracking (DSMT)”, is a single-loop algorithm that utilizes the
momentum tracking technique as well as the Loopless Chebyshev Acceleration
(LCA) method. We show that DSMT can asymptotically achieve comparable
convergence rates as centralized stochastic gradient descent (SGD) method under
a general variance condition regarding the stochastic gradients. Moreover, the
number of iterations (transient times) required for DSMT to achieve such rates
behaves as 𝒪(n^5/3/(1-λ)) for minimizing general smooth
objective functions, and 𝒪(√(n/(1-λ))) under the
Polyak-Łojasiewicz (PL) condition. Here, the term 1-λ denotes the
spectral gap of the mixing matrix related to the underlying network topology.
Notably, the obtained results do not rely on multiple inter-node communications
or stochastic gradient accumulation per iteration, and the transient times are
the shortest under the setting to the best of our knowledge.
更多查看译文
AI 理解论文
溯源树
样例
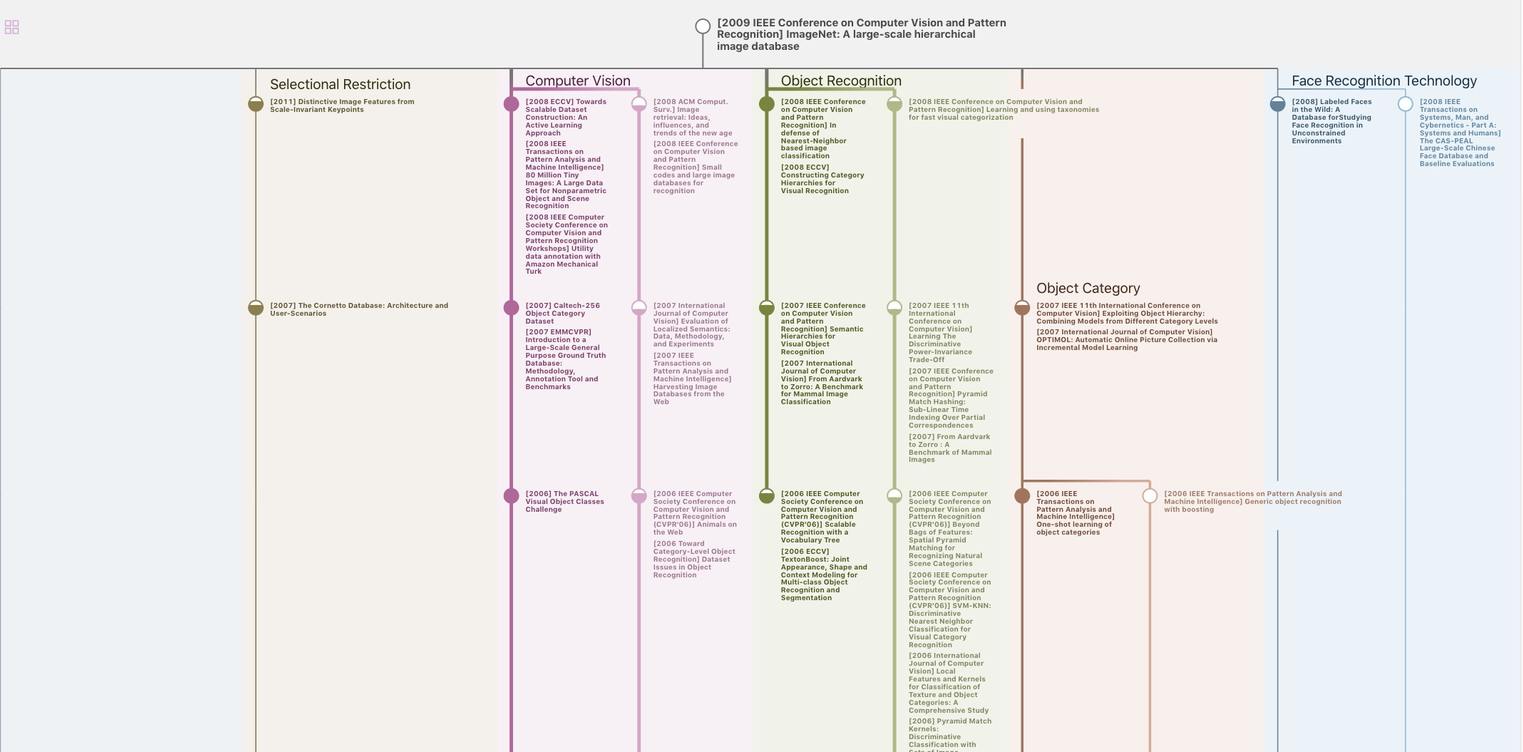
生成溯源树,研究论文发展脉络
Chat Paper
正在生成论文摘要