Expressivity of parameterized quantum circuits for generative modeling of continuous multivariate distributions
arxiv(2024)
摘要
Parameterized quantum circuits have been extensively used as the basis for
machine learning models in regression, classification, and generative tasks.
For supervised learning their expressivity has been thoroughly investigated and
several universality properties have been proven. However, in the case of
quantum generative modeling, the situation is less clear, especially when the
task is to model distributions over continuous variables. In this work, we
focus on expectation value sampling-based models; models where random variables
are sampled classically, encoded with a parametrized quantum circuit, and the
expectation value of fixed observables is measured and returned as a sample. We
prove the universality of such variational quantum algorithms for the
generation of multivariate distributions. Additionally, we provide a detailed
analysis of these models, including fundamental upper bounds on the
dimensionality of the distributions these models can represent. We further
present a tight trade-off result connecting the needed number of measurements
and qubit numbers in order to have universality for a desired dimension of
output distribution within an error tolerance. Finally we also show that the
data encoding strategy relates to the so-called polynomial chaos expansion,
which is an analog of the Fourier expansion. Our results may help guide the
design of future quantum circuits in generative modeling tasks.
更多查看译文
AI 理解论文
溯源树
样例
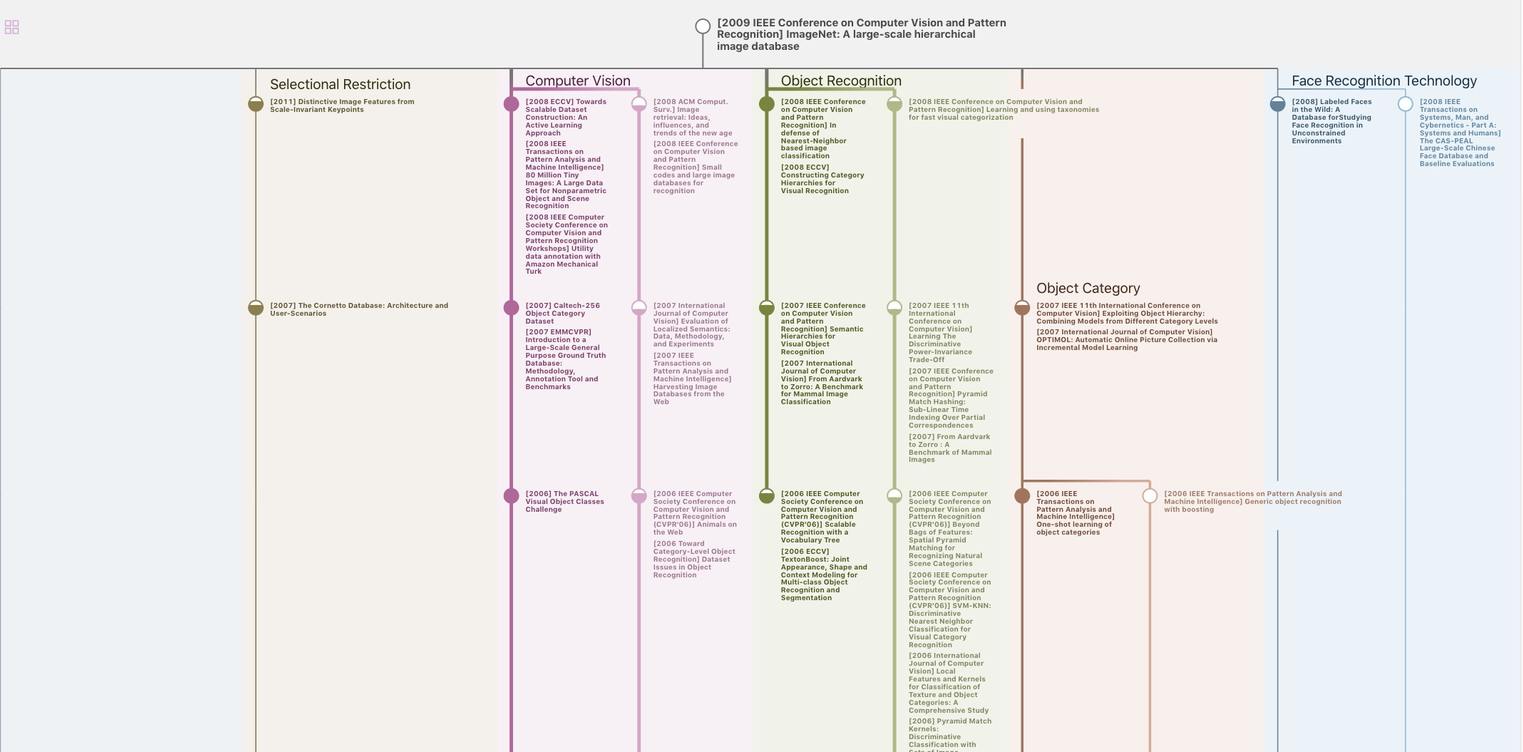
生成溯源树,研究论文发展脉络
Chat Paper
正在生成论文摘要